Validation of a Macroinvertebrate-based Index of
Nutrient Status in Streams Using Macroinvertebrate,
Water-chemistry, and Diatom Data
Richard J. Horwitz, Andrew Tuccillo, Donald F. Charles, Shane Neiffer, and Thomas Belton
Northeastern Naturalist, Volume 23, Issue 4 (2016): 532–554
Full-text pdf (Accessible only to subscribers. To subscribe click here.)
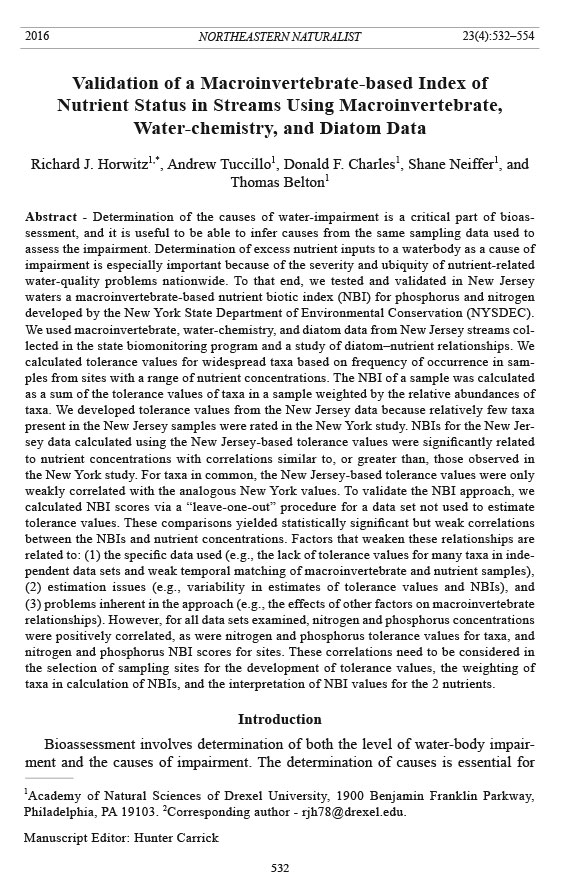
Access Journal Content
Open access browsing of table of contents and abstract pages. Full text pdfs available for download for subscribers.
Current Issue: Vol. 30 (3)

Check out NENA's latest Monograph:
Monograph 22
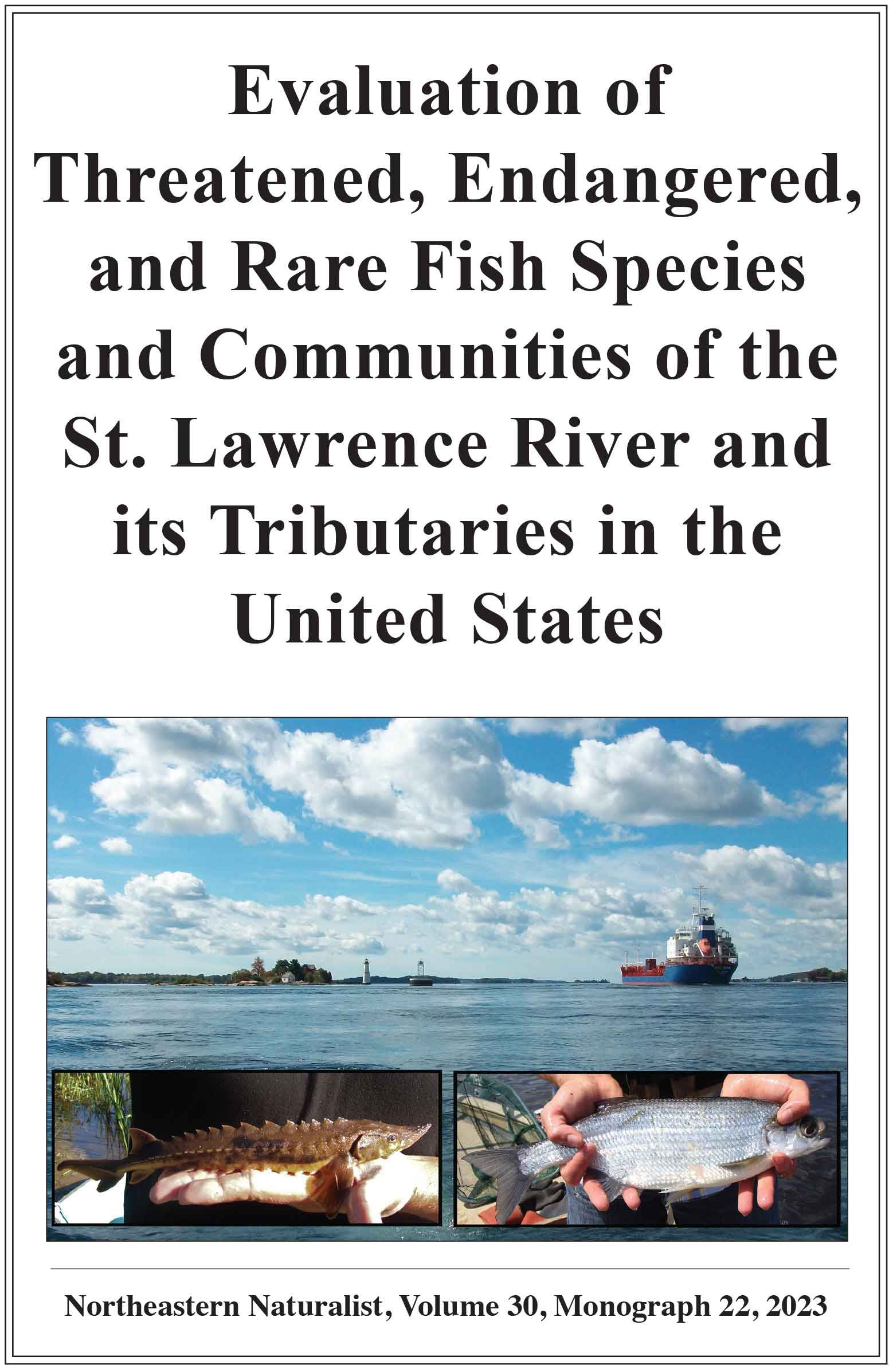
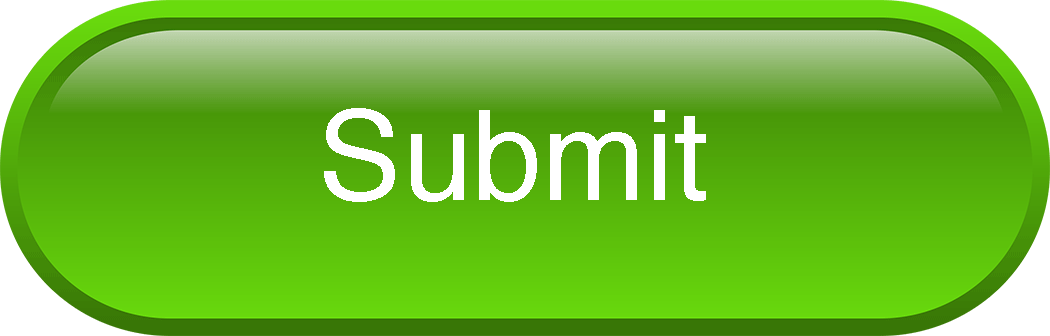


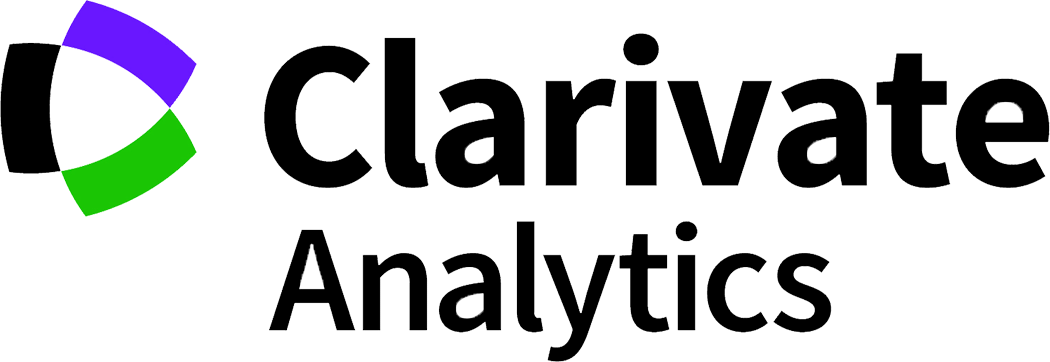
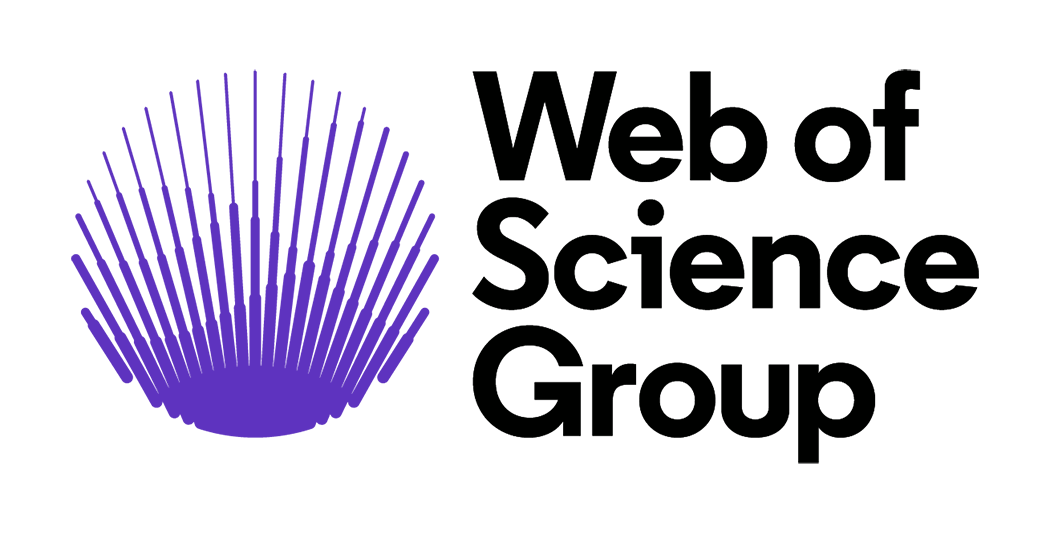
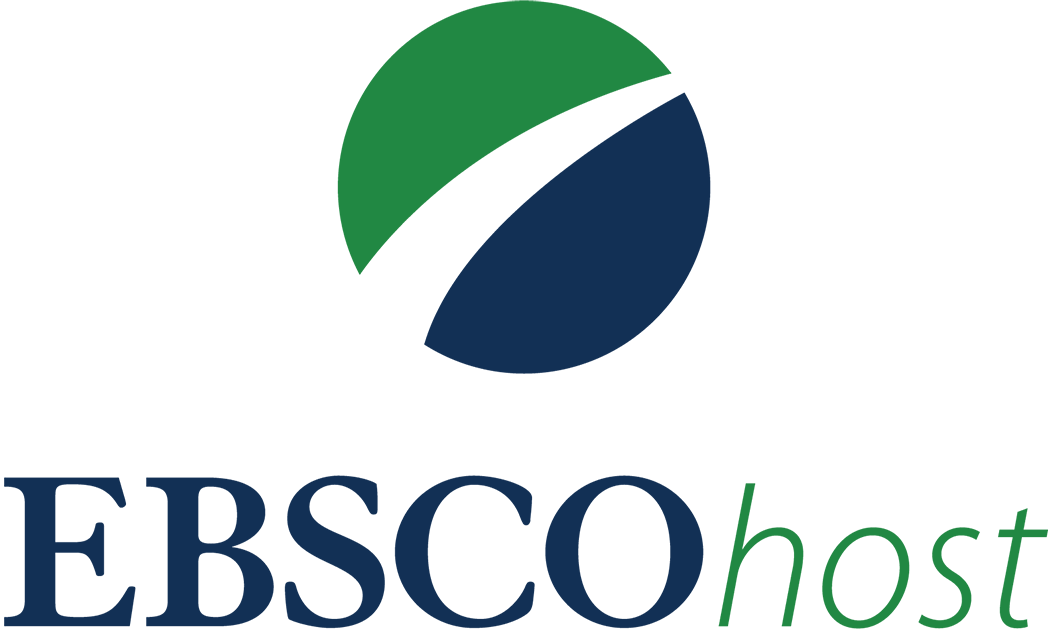
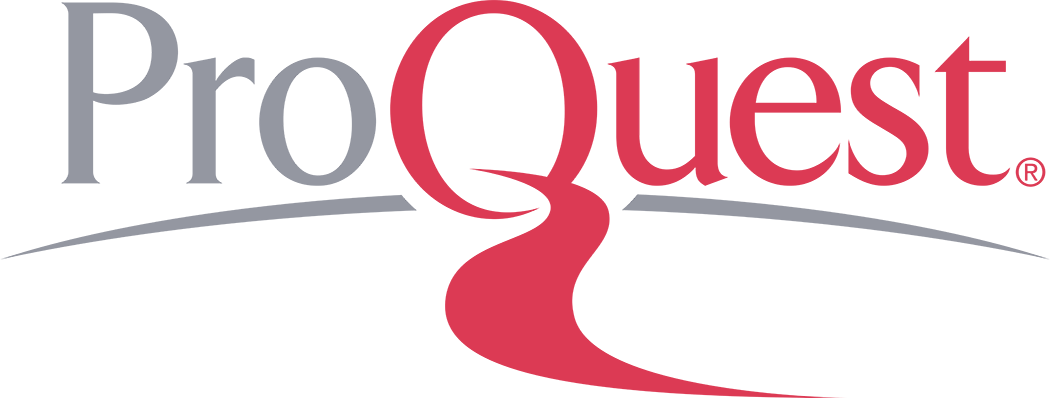
Northeastern Naturalist
532
R.J. Horwitz, A. Tuccillo, D.F. Charles, S. Neiffer, and T. Belton
22001166 NORTHEASTERN NATURALIST 2V3(o4l). :2533,2 N–5o5. 44
Validation of a Macroinvertebrate-based Index of
Nutrient Status in Streams Using Macroinvertebrate,
Water-chemistry, and Diatom Data
Richard J. Horwitz1,*, Andrew Tuccillo1, Donald F. Charles1, Shane Neiffer1, and
Thomas Belton1
Abstract - Determination of the causes of water-impairment is a critical part of bioassessment,
and it is useful to be able to infer causes from the same sampling data used to
assess the impairment. Determination of excess nutrient inputs to a waterbody as a cause of
impairment is especially important because of the severity and ubiquity of nutrient-related
water-quality problems nationwide. To that end, we tested and validated in New Jersey
waters a macroinvertebrate-based nutrient biotic index (NBI) for phosphorus and nitrogen
developed by the New York State Department of Environmental Conservation (NYSDEC).
We used macroinvertebrate, water-chemistry, and diatom data from New Jersey streams collected
in the state biomonitoring program and a study of diatom–nutrient relationships. We
calculated tolerance values for widespread taxa based on frequency of occurrence in samples
from sites with a range of nutrient concentrations. The NBI of a sample was calculated
as a sum of the tolerance values of taxa in a sample weighted by the relative abundances of
taxa. We developed tolerance values from the New Jersey data because relatively few taxa
present in the New Jersey samples were rated in the New York study. NBIs for the New Jersey
data calculated using the New Jersey-based tolerance values were significantly related
to nutrient concentrations with correlations similar to, or greater than, those observed in
the New York study. For taxa in common, the New Jersey-based tolerance values were only
weakly correlated with the analogous New York values. To validate the NBI approach, we
calculated NBI scores via a “leave-one-out” procedure for a data set not used to estimate
tolerance values. These comparisons yielded statistically significant but weak correlations
between the NBIs and nutrient concentrations. Factors that weaken these relationships are
related to: (1) the specific data used (e.g., the lack of tolerance values for many taxa in independent
data sets and weak temporal matching of macroinvertebrate and nutrient samples),
(2) estimation issues (e.g., variability in estimates of tolerance values and NBIs), and
(3) problems inherent in the approach (e.g., the effects of other factors on macroinvertebrate
relationships). However, for all data sets examined, nitrogen and phosphorus concentrations
were positively correlated, as were nitrogen and phosphorus tolerance values for taxa, and
nitrogen and phosphorus NBI scores for sites. These correlations need to be considered in
the selection of sampling sites for the development of tolerance values, the weighting of
taxa in calculation of NBIs, and the interpretation of NBI values for the 2 nutrients.
Introduction
Bioassessment involves determination of both the level of water-body impairment
and the causes of impairment. The determination of causes is essential for
1Academy of Natural Sciences of Drexel University, 1900 Benjamin Franklin Parkway,
Philadelphia, PA 19103. 2Corresponding author - rjh78@drexel.edu.
Manuscript Editor: Hunter Carrick
Northeastern Naturalist Vol. 23, No. 4
R.J. Horwitz, A. Tuccillo, D.F. Charles, S. Neiffer, and T. Belton
2016
533
the development of effective plans for attaining water-quality standards and designated
uses (Yoder and DeShon 2003). Excessive nutrient inputs are a widespread
cause of eutrophic impairment, leading to the growth of nuisance levels of algae
and decreased dissolved oxygen (DO) levels in nearby receiving waters as well as
downstream rivers, lakes, and estuaries. However, problematic algal growth may
occur where eutrophication is not as evident, (e.g., in streams with high rates of
oxygenation). As a result, both nutrient and dissolved-oxygen criteria may be required
to provide a full assessment for the protection of water quality. It would be
efficient if data from existing sampling programs could be used both to quantify impairment
and to distinguish causes of impairments, including excess nutrient inputs.
For example, Yoder and Rankin (1995) used multi-metric indices to distinguish 9
different groups of anthropogenic activities that may lead to impairment. Excess
nutrients were associated with many of these sources, such as conventional wastewater-
treatment plants, combined-sewer overflows, nonpoint agriculture sources,
and livestock access. Bryce and Hughes (2003) distinguished mining sources from
agricultural sources using multi-metric fish, benthic macroinvertebrate, and diatom
multi-metric indices. They noted that agricultural effects were associated with several
stressors, such as sedimentation and nutrient enrichment. Other studies (e.g.,
Bae et al. 2011, Bedoya et al. 2011, Hering et al. 2006, Piliere et al. 2014) have
correlated responses of indices to different stressors, including nutrients, but did not
design specific indices for responses to groups of stressors.
The New York State Department of Environmental Conservation (NYSDEC)
developed nutrient biotic indices (NBIs) for evaluating nutrient conditions using
data on benthic macroinvertebrate assemblages (Smith et al. 2007). These NBIs are
directly linked to nutrient concentrations, rather than the activities that produce a
variety of ecological impacts.
In this paper, we test the precision and generality of the NBIs, using a
methodology similar to Smith et al. (2007) on an independent macroinvertebrate and
water-chemistry data set from New Jersey (NJ). The basic objectives of the study
were to: (1) determine the generality of the NBI approach by applying it to data from
NJ, (2) determine the robustness of tolerance values for macroinvertebrate species
developed in New York (NY) by using them with the NJ data, and (3) validate the robustness
of the NBI method by correlating NBIs to chemistry and inferred-chemistry
data that are independent of the chemistry data used to calculate the NBIs.
We calculated the NBIs as the average of the nutrient-tolerance values for taxa
in each sample, weighted by the proportion of each taxon in the sample. We determined
the nutrient-tolerance values for common macroinvertebrate taxa from
modal values of nutrient concentrations where these taxa were collected. These
NBIs are similar to inference models (ter Braak and Juggins 1993) in structure because
they use observed nutrient-concentration–species-occurrence relationships
to infer nutrient status from the sum of species occurrences in samples. However,
the method does not directly estimate nutrients but defines an ordinal scale (the
NBIs). The 2 NBIs correlate with increasing mean total phosphorus (TP) and nitrate
+ nitrite (NO3) values, respectively, and define a 3-tiered scale of nutrient
Northeastern Naturalist
534
R.J. Horwitz, A. Tuccillo, D.F. Charles, S. Neiffer, and T. Belton
2016 Vol. 23, No. 4
status (i.e., oligotrophic, mesotrophic, and eutrophic) using cluster analysis of
invertebrate assemblage data. The NBIs appear to accurately reflect differences in
stream trophic state.
Aquatic macroinvertebrates have been used for freshwater monitoring and
assessment for several decades by many agencies including the New Jersey Department
of Environmental Protection (NJDEP) and its Ambient Macroinvertebrate
Network (AMNET) program. AMNET has over 760 non-tidal stations statewide for
evaluating the attainment of aquatic-life use criteria under the US Clean Water Act,
based on metrics of overall impairment calculated from assemblage data (http://
www.nj.gov/dep/wms/bfbm/amnet.html). The NJDEP has also collected water
samples at a number of sites where macroinvertebrate sampling was conducted.
NJ shares 5 ecoregions with NY, 3 of which are contiguous. Therefore, NJ
represents a favorable situation for testing the applicability of the NYSDEC macroinvertebrate
NBIs. For this study, we followed Smith et al. (2007) and used the
relationships between NBIs and water-chemistry measurements to test the ability of
the NBIs to detect nutrient impairment. However, it is important to note that parameters
other than nutrient concentration may be equally important for bioassessment,
such as excessive algal growth, changes in algal (diatom) community structure,
and/or oxygen depletion. Subsequent development of the NBIs could be strengthened
by matching NBI scores to these significant endpoints. For example, Smith et
al. (2013) used break-point analyses to determine the nutrient concentrations that
define thresholds for changes in macroinvertebrate and diatom assemblages. In addition,
the biological condition gradient (BCG) was used for New Jersey streams
to link macroinvertebrates (NJDEP 2007) to changes in ecosystem structure and
function; algal–diatom metrics (trophic diatom index) were developed and augmented
by BCG to quantify nutrient effects on water quality (Charles et al. 2010,
Hausmann et al. 2016)
Methods
Tasks and work flow
The basic approach of this study was to validate the NY method, both in terms
of statistical approach and the specific macroinvertebrate tolerance values. Specific
tasks were to:
1. Compile existing water-chemistry data for NJ sites, and develop a database
containing linked water-chemistry and AMNET macroinvertebrate data.
2. Obtain NY nutrient-tolerance values for macroinvertebrate taxa and calculate
NO3 and TP NBIs for NJ sites based on the NY tolerance value.
3. Relate the NO3 and TP NBIs to water-chemistry data, to validate use of the
NY method and tolerance values.
4. Develop new tolerance-values based on NJDEP data and use these to
calculate new NBIs.
5. Relate the new NBIs to water-chemistry data.
6. Validate the new NBIs using a leave-one-out approach.
Northeastern Naturalist Vol. 23, No. 4
R.J. Horwitz, A. Tuccillo, D.F. Charles, S. Neiffer, and T. Belton
2016
535
7. Compare the macroinvertebrate NBIs with the diatom-based nutrient indices
developed for NJDEP by PCER to validate the NY method.
8. Compare tolerance values developed for NY and NJ as a measure of the
robustness of the estimation procedure.
The data flow for these tasks is outlined in Figure 1.
Data compilation
The NJDEP AMNET data were collected at each site from multiple, traveling Dframe
kick samples within riffle and run habitats. The "jab and sweep" method was
employed (Barbour et al. 1999); a minimum of 20 jabs/sweeps were conducted, proportioned
approximately to the numbers of each habitat type present. In all cases,
stream-distance sampled approached, but did not exceed, 100 m. Level of effort was
consistent for all sites (NJDEP 2012). These data consisted of relative abundances
Figure 1. Schematic of data sources and calculations used for the 5 methods of NBI validation.
Rectangles represent data sources, hexagons denote calculated indices, and circles
indicate statistical comparisons. Single-headed, solid arrows indicate the use of data sources
in or the direction of index estimation. Double-headed, solid lines show entities compared
in the statistical analyses. A dashed line shows analyses performed using leave-one-out
validation. A single line around a circle indicates that the regression variables are not independent
because the chemistry data were used to estimate the nutrient index. A double line
around a circle indicates independence of the regression variables. The suffixes d, c, and t
refer to assemblage data, water chemistry data, and tolerance values, respectively.
Northeastern Naturalist
536
R.J. Horwitz, A. Tuccillo, D.F. Charles, S. Neiffer, and T. Belton
2016 Vol. 23, No. 4
of macroinvertebrate taxa. The individuals from the sample were identified to the
lowest practicable taxonomic level, usually genus or species: 60% of records were
identified to species level, 10% to morphospecies, 37% to genus, and 2% (Corixidae,
Sphaeriidae, Enchytraeidae, and Chironomidae) to a higher level.
Two data sets were available for our study. The main data set contained raw
data for macroinvertebrates from 97 sites (Fig. 2). These data have also been used
to relate macroinvertebrate assemblages to land-use and fish data (Flinders et al.
2008). The NJDEP provided water-chemistry data from 1996 to 2007 from these
sites. We excluded data from southern NJ from most analyses because of possible
differences in taxa composition between these sites and those in northern NJ. Our
second data set was provided by D.F. Charles and consisted of water-chemistry and
diatom samples taken at the same time at 29 AMNET sites. We employed nutrient
concentrations and diatom-inferred nutrient concentrations from the second data
set for comparison with NBIs. Macroinvertebrate data from the second data set
were not included in the analyses discussed in this paper, although we included
them in additional validation procedures not reported here (R.J. Horwitz et al.,
unpubl. data).
Methods used to measure individual water-chemistry parameters differed among
datasets. For consistency, we included only TP, NO3, and total nitrogen (TN) data in
our analyses. These parameters were measured using methods listed in Table 1. For
Figure 2. Map of NJ and NY study sites and the ecoregions in which sites are located.
Northeastern Naturalist Vol. 23, No. 4
R.J. Horwitz, A. Tuccillo, D.F. Charles, S. Neiffer, and T. Belton
2016
537
Table 1. Parameters and method names for nutrient analyses in NJDEP and NJAI data sets.
Agency
Source Agency characteristic method
Parameter Occurrence agency name code Agency method name
Nitrate + nitrite NJDEP measurements USGS Nitrite + nitrate, water, I-2545-90 Nitrogen, nitrite + nitrate, colorimetry,
(main and validation data sets) filtered, mg/L as N cadmium reduction-diazotization,
automated-segmented flow
Nitrate + nitrite NJDEP measurements EPA Nitrogen, nitrite (NO2) + 353.2 Nitrate (as N) Automated diazotization
(main and validation data sets) nitrate (NO3) as N w/o Cd reduction column
Total phosphorus NJDEP measurements EPA Phosphorus as P 365.4 Total phosphorus after block digestion
(main and validation data sets)
Total phosphorus NJDEP measurements USGS Phosphorus, water, I-4610-91 Determination of total phosphorus by a
(main and validation data sets) unfiltered, mg/L Kjeldahl digestion method and an automated
colorimetric finish that includes dialysis
Nitrate + nitrite NJDEP measurements USGS Nitrogen, nitrite + nitrate, I-2546-91 Nitrogen, nitrite + nitrate, low ionic-strength
(validation data set only) dissolved water, colorimetry, cadmium reductiondiazotization,
automated-segmented flow
Total phosphorus PCER measurements EPA Phosphorus as P 365.2 Phosphorus by single-reagent colorimetry
(main data set and NJAI study)
Nitrate + nitrite PCER measurements EPA Nitrogen, nitrite (NO2) + 353.2 Nitrate-nitrite nitrogen by cadmium
(main data set and NJAI study) nitrate (NO3) as N reduction
Total Kjeldahl PCER measurements (NJAI only) EPA Total Kjeldahl nitrogen 351.2 Total Kjeldahl nitrogen by semi-automated
nitrogen colorimetry
Northeastern Naturalist
538
R.J. Horwitz, A. Tuccillo, D.F. Charles, S. Neiffer, and T. Belton
2016 Vol. 23, No. 4
many sites, only N or P data were available. As a result, sample sizes for analyses
involving each nutrient were smaller than the total number of sites in the database.
We conducted 5 analyses to provide varied approaches to validation (Fig. 1):
1. We used NY tolerance values (from Smith et al. 2007) to calculate NBIs for
the NJ data; regressions of these NBIs with the chemistry data from NJ provide
a validation of the NY method. This was appropriate because data used
to estimate the nutrient optima (i.e., the NY macroinvertebrate and chemistry
data) were not used in the regression. A high correlation indicates
the generality of both the NBI approach and the specific tolerance-values
estimated by Smith et al. (2007); a low correlation could result from differences
in tolerance of each taxon across the region, presence of many taxa in
the NJ data for which NY did not estimate tolerance values, problems with
taxonomic precision and accuracy, problems with relevance with the chemistry
data, or lack of generality of the NBI approach.
2. We calculated NJ tolerance values using the NJ macroinvertebrate and
chemistry data; the macroinvertebrate data and tolerance values were
used to calculate NBIs; regressions of these NBIs and the NJ chemistry
data are directly comparable to the evaluation technique used by Smith et
al. (2007). However, the NBIs are not independent of the chemistry data,
and thus do not provide an independent evaluation. The NBIs provide a
test of the generality of the approach, but do not provide a true validation.
3. We used the NJ macroinvertebrate and chemistry data from the main data
set to estimate tolerance values, macroinvertebrate data from the diatom
sites and these tolerance values to calculate NBIs, and the regression of
these NBIs along with the inferred-concentration indices derived from the
diatom analyses to assess the consistency of the macroinvertebrate NBIs
with the diatom-based nutrient indices. These tests provide a stronger
test and validation of the NBI approach because they correlate NBIs with
data independent of those used to estimate the NBIs, and they use inferred-
chemistry data, which show fewer problems with relevance of the
chemistry data to the macroinvertebrate assemblages. A low correlation
suggests problems with the NBI approach, in terms of the basic approach
or statistical estimation issues.
4. We used the NJ macroinvertebrate and chemistry data to estimate weighted-
average nutrient concentrations for each taxon. The weighted-average
nutrient concentrations were used to infer nutrient-concentration indices,
which we then compared to the measured nutrient concentrations. We
employed a leave-one-out procedure (see below) to conduct these calculations
so that the inferred nutrient-indices were independent of the
measured nutrient-concentrations. This analysis provides a validation of
major elements of the NBI approach. The leave-one-out procedure efficiently
uses the data, avoiding loss of precision in validating separate
data sets. As with approach (3), a low correlation suggestions theoretical
or statistical problems with the NBI approach.
Northeastern Naturalist Vol. 23, No. 4
R.J. Horwitz, A. Tuccillo, D.F. Charles, S. Neiffer, and T. Belton
2016
539
5. We employed a procedure similar to approach (4), but we omitted
the leave-one-out procedure. A comparison of approaches (4) and (5)
indicates the importance of the non-independence of the inferred and
measured chemistry values; if methods (4) and (5) produce similar values
of correlation, the non-independence of the NBI and test data may not be
a large problem. However, a much higher correlation for (4) than for (5)
would suggest that the apparent success of the NBI approach may depend
on the non-independence of the evaluation.
Data matching and filtering
Smith et al. (2007) used water-chemistry data collected within 90 days of the
macroinvertebrate sampling. However, very few nutrient and macroinvertebrate
samples from NJ sites were collected at the same or similar times. Therefore,
we linked the water-chemistry data from the main data set to macroinvertebrate
samples if the water samples were collected in the same season and ≤5 years of
macroinvertebrate sample collection. We defined seasons as: spring = 1 March–31
May, summer = 1 June–31 August, fall = 1 September–31 November, and winter =
1 December–28/29February.
At many sites, 1 macroinvertebrate sample corresponded to >1 nutrient measurement
that met the temporal matching criteria. In these cases, we used the
averages among all the eligible measurements of a nutrient. For the main database,
averages of TP and NO3 were 2.65 and 4.15, respectively.
We removed extremely high and low nutrient values prior to analyses using a
qualitative technique whereby we viewed a distribution of the data points using
JMP 7 software (SAS, Cary, NC), and identified points within the long tails at both
ends for exclusion.
After all the above criteria were applied, samples from 97 sites were available
for analysis as part of the main database. We excluded taxa found in less than 2%
of sites (Smith et al. 2007), resulting in a total of 254 invertebrate taxa.
Calculation of nutrient optima for macroinvertebrate taxa and NBIs for
samples
We calculated the nutrient optima, NBIs, and NO3 and TP as follows (see Smith
et al. 2007 for further details). We ordered the set of sample sites used to calculate
optima by the appropriate nutrient concentration (i.e., NO3 or TP) and divided them
into 15 bins. The nutrient ranges within bins were the same as those used by Smith
et al. (2007). The distribution of NJ nutrient data differed somewhat from that of
the NY dataset. As a result, the number (ni) of samples in each bin (bi.) differed
somewhat, typically with more samples in the higher nutrient-concentration bins.
We calculated the mean nutrient concentration of each bin (mi) and the frequency
(fi) of each taxon (t) in each bin (i.e., the proportion of ni samples in which the taxon
was present). The nutrient optima were calculated as:
Ot = Σ(fi * mi) / Σ(fi).
We ordered and divided into 11 bins nutrient optima with approximately equal
Northeastern Naturalist
540
R.J. Horwitz, A. Tuccillo, D.F. Charles, S. Neiffer, and T. Belton
2016 Vol. 23, No. 4
numbers of taxa in each bin. We defined the rank of each bin as the tolerance values
(TVi) of each taxon in that bin. The TVis ranged from 0 to 10, with the lowest value
corresponding to occurrence at low nutrient-concentrations.
For each sample, we calculated a nutrient value (NBIT) from the sum of the tolerance
values of taxa in the sample weighted by the proportion (pi) of each taxon in
the sample:
NBIT = Σ(pt * TVt).
For samples in which tolerance values were not available for all taxa in the samples,
we used the proportion of individuals among all rated individuals, instead of the
proportion of individuals in the entire sample.
Application of NY-based tolerance values to NJ data (method 1)
The first application of the NBI strategy to NJ streams used the taxon-specific
tolerance values for NY (Smith et al. 2007) to calculate NBI scores for NJ sites.
Taxon coding differences between NY and NJ identifications were resolved by
eliminating differences below the species level and by including “nr. taxon x”designations
(i.e., specimen was either species x or a very similar species). However,
even after this level of resolution, there was relatively low overlap between the NJ
and NY taxonomic lists, so that NY tolerance values could be applied to only 69 of
254 NJ taxa (about 27.5%). We calculated NBI scores of NJ sites using the process
described in Smith et al. (2007); the abundances of unrated NJ taxa were ignored by
the calculations. We regressed the TP and NO3 NBI scores against log-transformed
TP and NO3 values.
Calculation of tolerance values using NJ data and of new NBIs (method 2)
We calculated a second set of tolerance values for all NJ taxa, except rare taxa,
using macroinvertebrate-abundance and nutrient data collected in NJ. We determined
tolerance values using the same methods as Smith et al. (2007) and described
above, using their 15 original nutrient bins to calculate nutrient optima in the first
phase of the calculations. We regressed the resulting NBI scores against corresponding
log-transformed nutrient values as described above.
Macroinvertebrate NBIs and diatom-based nutrient indices (method 3)
We conducted a regression of the TP- and NO3-based NBI scores from sites used
to develop the diatom nutrient index with the diatom-inferred TP and TN values.
This analysis compares nutrient status estimated from macroinvertebrates with that
from diatoms. Multiple diatom samples were taken at the majority of sites, resulting
in multiple inferred nutrient values. In these cases, the inferred values were averaged
before comparison with NBI scores.
Nutrient inference from macroinvertebrate assemblages and leave-one-out
validation
We used the macroinvertebrate data in the main data set to infer NO3 and TP
concentrations by calculating nutrient optima for each taxon (excluding rare
Northeastern Naturalist Vol. 23, No. 4
R.J. Horwitz, A. Tuccillo, D.F. Charles, S. Neiffer, and T. Belton
2016
541
taxa) and then inferring nutrient-concentration indices for each sample from the
nutrient optima:
INFi = Σ(fit * Ot) / Σ(fi)
where fit is the proportion of taxon t in sample i, and Ot is the nutrient optimum for
taxon t. These estimates are not scaled to truly estimate nutrient concentrations, but
are directly related to such estimates. The main use of these indices is for correlation
with measured concentrations; thus, scaling these indices to form a concentration
estimate is not necessary. For simplicity, we refer to these indices as inferred nutrients
in the remainder of the paper. We performed this procedure in 2 ways. One
(method 4) included all data, so that the nutrient inferences for each sample were
based on nutrient optima which were partly based on data from that sample. The
other (method 3), a leave-one-out procedure, calculated nutrient optima over all
samples except one, and then used these optima to infer nutrient concentrations in
the excluded sample. We employed this procedure over all samples (excluding each
sample in turn), so that each inferred-nutrient concentration was independent of the
measured-nutrient data for that sample.
We undertook several other types of validation with different data sets to estimate
tolerance values and evaluate NBIs derived from these tolerance values,
which produced results similar to those reported here (R.J. Horwitz et al., unpubl.
data).
Statistical analyses
We regressed NBI scores and inferred nutrient-concentrations against log-transformed
nutrient values. In some cases, we regressed residuals against factors which
might affect the accuracy of the regressions. We performed all data manipulations
with Excel and Access (Microsoft), and statistical analyses using JMP 7 and SigmaPlot
(Systat Software, Inc.).
Results
Application of NY-based tolerance values to NJ data
The relationships between the NBI scores calculated with NY-based tolerance
values and log-transformed nutrient concentrations were positive and significant
but very weak (Table 2: rows 1a, 1b). The poor relationships were at least partially
due to the relatively low proportion of taxa (2–67%) assigned tolerance values in
any given sample, which in turn stemmed from the relatively low overlap between
the 2 taxonomic lists and the occurrence of some taxa with high abundance in
single samples. A few taxa that were widespread and abundant in the NJ samples,
e.g., Dugesia tigrina (Girard) (up to 60% relative abundance) and Neocleon (up to
31% relative abundance), were not reported in the NY samples. Other taxa were
found in only a few NJ samples, but in high relative abundance (10–50%) in single
samples. These taxa include Amphinemura delosa (Ricker), Diamesa nivoriunda
(Fitch), Dubiraphia, Eurylophella temporalis (McDunnough), Isonychia arida
(Say), Leuctra truncate Claassen, Nemoura, Palucidella articulata (Ehrenberg),
Northeastern Naturalist
542
R.J. Horwitz, A. Tuccillo, D.F. Charles, S. Neiffer, and T. Belton
2016 Vol. 23, No. 4
Table 2. Relationships between tolerance values, NBI values, and nutrient concentrations. TP inflo and N inflo are the inferred concentrations using the
leave-one-out procedure; TP infall and N infall are the inferred concentrations using all data..
Source of data Regression results
Macroinvertebrate Chemistry
Method Index Tolerance values data data n R2 P R2 R2
logTP &
log(NO3 NBI-P &
NBI-P vs log(TP) or TN) NBI-N
1a NBI NYSDEC Main Main 68 0.19 less than 0.0002 0.38 0.58
2a NBI Main dataset Main Main 68 0.45 less than 0.0001 0.62
3a NBI Main dataset Diatom Diatom inferred 29 0.62 less than 0.0001 0.61 0.73
TP inflo vs TP inf vs
P inf vs log(TP) TP infall NO3 inf
4a Inferred nutrient Main dataset Main Main 68 0.43 less than 0.00001 0.63 0.73
5a Inferred nutrient Main dataset (leave-one-out) Main Main 68 0.12 less than 0.0039 0.72
Nitrogen ([Nitrate + nitrite]
or [Total nitrogen])
1b NBI NYSDEC Main Main 97 0.15 less than 0.0001
2b NBI Main dataset Main Main 97 0.51 less than 0.0001
3b NBI Main dataset Diatom Diatom inferred 29 0.66 less than 0.0001
N inflo vs
N inf vs log(NO3 or TN) N infall
4b Inferred nutrient Main dataset Main Main 97 0.47 less than 0.00001 0.75
5b Inferred nutrient Main dataset (leave-one-out) Main Main 97 0.18 less than 0.000014
Northeastern Naturalist Vol. 23, No. 4
R.J. Horwitz, A. Tuccillo, D.F. Charles, S. Neiffer, and T. Belton
2016
543
Paralauterborniella, Paratendipes albimanus (Meigen), Perlesta, Plumatella, 2
Prosimulium spp., 2 Pesudocleon spp., and 5 species of Symphitopsyche. Other differences
reflect different levels of identification between the NJ and NY programs,
particularly of non-insect taxa. For example, species of the amphipod genera Caecidotea
and Gammarus, the gastropod genera Physella and Pisidium, and several
annelids were reported by NJ, while NY reported only generic identifications of amphipods
and gastropods and suborder of annelids. Conversely, NY reported species
of Drunella, while NJ reported only a generic identification. Some other genera,
such as Ephemerella, Microtendipes, Nais, Orthcladius, Polypedilum, Simulium,
and Stenelmis, were represented in both states, but each with at least 1 species not
found in the other state.
Residuals from the NBI–nutrient regressions were weakly negatively related to
the number of taxa assessed (TP: n = 68, R2 = 0.11, P < 0.0051; NO3: n = 97, R2 =
0.08, P < 0.0039). The lack of a strong temporal association between the chemistry
and macroinvertebrate samples may have also played a role in the weakness of the
correlation, but there were no significant relationships between the residuals of TP
or NO3 and the inter-sample interval.
Calculation of tolerance values using NJ data
The regressions of the NBI scores calculated with NJ-based tolerance values
against log-transformed nutrient concentrations were positive, significant, and
moderately strong (Table 2: rows 2a, 2b); R2 values (0.45 and 0.51 for TP and NO3,
respectively) were similar or higher than those reported by Smith et al. (2007): R2 =
0.46 for P-NBI and TP and 0.32 for N-NBI and NO3.
For shared taxa, the NJ-based tolerance values were only weakly correlated with
the NY-based tolerance values (TP: n = 69, R2 = 0.13, P < 0.0026; NO3: n = 69, R2 =
0.06, P < 0.048).
Relationships between NBIs and nutrient concentrations inferred from diatoms
NBI scores calculated from NJ-based tolerance values were correlated with
diatom-based inferred TN and TP indices (Ponader et al. 2007; Fig. 3). The relationships
were fairly strong despite relatively low sample numbers and poor temporal
association between samples (Table 2: rows 3a, 3b).
Nutrient optima: Estimation and validation of NJ-based tolerance values
The relationships between inferred and measured nutrients using all data were
highly significant (Table 2: rows 4a, 4b). The r2 values were slightly lower than
those between the NBIs and measured nutrients (rows 1 vs 3). However, the inferred
nutrient-concentrations estimated using the leave-one-out procedure were much
less correlated with the observed nutrient concentrations (Fig. 3; Table 2: rows 5a,
5b). For TP, the slope of both inferred TP–measured TP relationships were much
less than 1 (0.086 for inference with all data, and 0.037 for leave-one-out inference).
The difference between the logs of the 2 methods of inferring TP increased with nutrient
concentration (Fig. 4). At low TP concentrations, the inferred concentrations
Northeastern Naturalist
544
R.J. Horwitz, A. Tuccillo, D.F. Charles, S. Neiffer, and T. Belton
2016 Vol. 23, No. 4
Figure 3. Relationship between NBI scores from macroinvertebrates and nutrient concentrations
inferred from diatoms. (A) Total phosphorus and (B) NO3.
Northeastern Naturalist Vol. 23, No. 4
R.J. Horwitz, A. Tuccillo, D.F. Charles, S. Neiffer, and T. Belton
2016
545
Figure 4. Relationships between nutrient concentrations (mg/L) inferred from macroinvertebrate
data using the leave-one-out procedure versus measured nutrient concentrations. (A)
Total phosphorus and (B) NO3.
Northeastern Naturalist
546
R.J. Horwitz, A. Tuccillo, D.F. Charles, S. Neiffer, and T. Belton
2016 Vol. 23, No. 4
were slightly greater than the measured concentrations. At high TP-concentrations,
the inferred concentrations were much less than the measured concentrations. For
nitrate, the slope of inferred NO3 versus measured NO3 were also much less than 1
(0.20 for inference with all data and 0.10 for leave-one-out inference; Fig. 3). As
with TP, the difference between the inferred NO3 from the 2 methods increased with
nutrient concentration (Fig. 5), with large differences for a few points at each end
of the NO3 gradient.
Discussion
The biomass and/or species composition of benthic macroinvertebrates have
been related to nutrient enrichment, either as sources of enrichment or as other
chemical properties related to enrichment. Spieles and Mitsch (2000) found that average
diel dissolved oxygen and specific conductivity were the best environmental
predictors of invertebrate-community metrics in high- and low-nutrient constructed
wetlands; these variables, along with chemical oxygen demand and nitrate-nitrogen,
described nearly 90% of the invertebrate-community index (ICI) variation in
a 4-predictor regression model. Biggs et al. (2000) found that biomass and species
composition of invertebrates responded to nutrient-enrichment experiments, but the
response was mediated by the type of fish predator present. Camargo et al. (2004)
found that a multimetric assessment of nutrient enrichment in impounded rivers
may be a useful technique for the biological assessment of nutrient enrichment in
fluvial ecosystems. More recently, Smith et al. (2007) demonstrated a relationship
between macroinvertebrate assemblages (described by NBI scores) and nitrogen
and phosphorus concentrations for NY streams. These NBIs were subsequently
used by Smith et al. (2013) to determine change points in biological assemblages.
Using analogous methods for calculating NBIs, we found similar relationships between
NBI scores and chemical concentrations for NJ streams.
This similarity in strength of NBI-chemical data between the NY and NJ analyses
suggests that differences between the NY and NJ protocols (e.g., wider temporal
variation between macroinvertebrate and chemistry samples for NJ) did not greatly
affect the results. However, in both of these analyses, relationships were tested using
the same data that were used to estimate the nutrient optima for taxa. Use of the
NY tolerance values on the NJ dataset provides 1 type of independent validation,
i.e., tolerance values from NY data were tested using a second dataset. This analysis
produced weak relationships between NBI and water chemistry. The relationship
between estimates of nutrient optima of the same taxa from the NJ data and from
the NY data also tests the robustness of the tolerance estimates. When we included
taxa that were relatively common in samples from both states, the 2 sets of estimates
were only weakly correlated. This finding suggests imprecision in estimation
of optima, imprecision in measurement of water chemistry, interactions with other
factors, or different responses of macroinvertebrate taxa in the 2 states.
The leave-one-out procedure provided a second validation. There was a large
decrease in model fit from the all-data inference to the leave-one-out inference.
The difference in model fit shows the effect of extreme data points on estimation of
Northeastern Naturalist Vol. 23, No. 4
R.J. Horwitz, A. Tuccillo, D.F. Charles, S. Neiffer, and T. Belton
2016
547
Figure 5. Relationship between the difference in inferred nutrient concentrations by 2 procedures
(leave-one-out versus all data) and nutrient concentrations. (A) Total phosphorus
and (B) NO3.
Northeastern Naturalist
548
R.J. Horwitz, A. Tuccillo, D.F. Charles, S. Neiffer, and T. Belton
2016 Vol. 23, No. 4
nutrient optima because extreme points have a large effect on estimates of nutrient
optima. The low slopes of the inferred nutrient–measured concentration regressions
are an example of the edge effect (Birks 1998, ter Braak and Juggins 1993), which
commonly occurs in inference models. The edge effect may arise from the inherent
asymmetry in the distribution of taxa near the extremes of environmental gradients,
resulting in systematic bias of nutrient optima toward the mean. In many inference
models, the true environmental gradients are statistically recovered by de-shrinking
(Birks 1998).
In addition, we performed split data-validation using several different pairs of
training and validation data. Similar to our results for the leave-one-out tests, we
detected significant, but weak, relationships between NBIs and water chemistry in
these validations (R.J. Horwitz et al., unpubl. data).
Although our analyses indicate a relationship between macroinvertebrate
assemblages and nutrient concentrations, NBIs based on the NJ data did not precisely
infer nutrient concentrations in independent samples. This outcome does
not invalidate the general NBI approach. There are several aspects of the analyses
which could contribute to the weakness of the observed NBInutrient
relationships:
statistical issues, such as the difficulty of producing independent estimates of tolerance
values for all taxa in a set of samples, the quality of the water-chemistry data
available for analysis, the particular mathematical definition of the NBI, and the
availability of estimates of tolerance values for various macroinvertebrate taxa.
More importantly, the weak relationships detected relate to conceptual issues associated
with the NBIs, including the causal basis for and the inherent variability in
relationships between macroinvertebrate assemblages and nutrient concentrations.
Availability of tolerance values for taxa
Typically, the NBI for a sample was based on only a portion of the taxa present
in the sample. Tolerance values could not be accurately estimated for rare or
infrequent taxa, so NBI scores were based only on the widespread taxa present
in each sample. This issue is important in validations with separate data sets because
those used for estimating tolerances and testing NBIs may share relatively
few taxa. The leave-one-out validation avoided many of the problems associated
with different taxonomic lists, but the results were also relatively weak. As noted
above, the taxonomic resolution of macroinvertebrate data affects the availability
of tolerance values.
Larger data sets would provide more samples with greater commonality in taxa
present, which would allow more-accurate tolerance estimates for a larger number
of taxa. A larger data set could also allow creation of sub-sets comprised of the
species common to all, which would minimize effects of other significant factors.
Tolerance values could be calculated from compilations of data from diverse
sources. This approach could provide robust information on a large number of taxa,
and resultant tolerance values would be independent of the data used to calculate
NBIs. However, such meta-analyses would encounter problems with taxonomic
consistency and accuracy of identifications.
Northeastern Naturalist Vol. 23, No. 4
R.J. Horwitz, A. Tuccillo, D.F. Charles, S. Neiffer, and T. Belton
2016
549
Quality and relevance of water-chemistry data
The consistency and relevance of the nutrient data affect estimation of tolerance
values and the relationship between NBI scores and nutrient concentrations. Most
of the water-chemistry samples were taken independently of the macroinvertebrate
samples, and the data for individual sites were several years apart in many cases.
Also, chemistry measurements were made using a variety of different methods (see
Table 1). We selected TP and NO3 as relevant nutrient forms that were measured
consistently across different programs. Other forms of P and N might exhibit a different
nutrient response by macroinvertebrates, but there were no consistent data
for these forms.
The response of macroinvertebrates to nutrients is temporally integrated, however,
which is one of the main advantages for using biological indices. Therefore,
we would not expect correlations between macroinvertebrate indices and a few
point-samples of nutrients to be as high as correlations with averages of more measurements
made over a longer period of time. For the diatom subset, the correlation
between P-NBIs and diatom-inferred TP was higher than any of the relationships
between NBIs and measured chemistry values. The inferred values represent temporally
integrated diatom responses.
Uptake of nutrients by periphyton reduces nutrient levels. In streams where P
is limiting, P may be reduced to very low concentrations. As a result, observed Pconcentrations
may be lower than the concentrations which stimulated periphyton
growth and resultant macroinvertebrate assemblages, weakening relationships
between biota and observed nutrient concentrations. In systems with correlated
sources of N and P, N may more accurately indicate high nutrient-loading.
The estimation of nutrient optima depends on the distribution of water chemistry
among samples used to make the estimates. Gaps in the distribution of nutrient
levels and low numbers of samples representing extreme nutrient levels weaken
the precision and accuracy of the estimates of nutrient optima. The NJ data had a
greater range in nutrient concentrations than the NYSDEC data used by Smith et
al. (2007), which might allow better estimates of nutrient optima. However, we
used the same nutrient bins as Smith et al., which may have obscured changes in
macroinvertebrate assemblages at extreme nutrient concentrations.
Causal basis of macroinvertebrate-nutrient relationships
The approach used by Smith et al. (2007) and in this study is empirical and
does not assume or incorporate causal bases for relationships between macroinvertebrate
assemblages and nutrient concentrations. Unlike algal responses (e.g.,
Charles et al. 2010, Ponader et al. 2007), macroinvertebrate responses are likely
to be indirect, reflecting either trophic effects or correlations between nutrients
and other factors which directly affect macroinvertebrates, especially fluctuating
oxygen concentrations and potentially other agricultural runoff-related inputs such
as pesticides. Trophic responses could include differences in macroinvertebrate
feeding groups (e.g., grazers) in response to the quantity and type of algal food.
Macroinvertebrates could be affected by changes in predator (e.g., fish) densities
Northeastern Naturalist
550
R.J. Horwitz, A. Tuccillo, D.F. Charles, S. Neiffer, and T. Belton
2016 Vol. 23, No. 4
or diet in response to nutrient-driven changes in production. Increases in nutrients
commonly occur in agricultural or urban watersheds, where changes in sedimentation,
geomorphology, hydrology, and temperature could have direct effects on
macroinvertebrates. Analyses of the relationships of estimated nutrient optima and
characteristics of macroinvertebrate taxa (e.g., feeding type, tolerance ratings) may
provide evidence of some of these causal relationships. Such analyses could be used
to improve estimates of nutrient optima or calculation of NBI scores.
The appropriate level of taxonomic resolution for these analyses is uncertain.
Estimated tolerance values frequently differed among species within genera, so that
species-level analyses should provide more-precise relationships than analyses using
higher-level taxonomy. However, finer levels of resolution result in fewer points
for the estimation of nutrient responses and fewer taxa with estimated tolerance
values available for estimating NBI scores.
Mathematical definition of NBIs
For each macroinvertebrate taxon, we calculated the nutrient optimum based on
frequency of occurrence across different nutrient levels. Use of relative abundance
within samples could improve sensitivity of the optima. For each sample, we calculated
the NBI from nutrient optima and relative abundance of each taxon (excluding
rare taxa). Various ways of weighting different taxa, e.g., giving more weight to
sensitive taxa, and more-precise estimates of optima could improve sensitivity of
the NBI. NBIs are subject to 2 estimation issues. First, generalist macroinvertebrate
taxa may be common but provide little information on nutrient status. Second, estimates
of nutrient optima for uncommon species are more likely to be imprecise.
To reduce the effects of these issues, the weight of each taxon to the overall index
could be inversely related to some measure of the specificity and reliability of that
taxon’s nutrient tolerance. For example, the weight could be inversely related to
the range or standard deviation of the distribution of the taxon across the nutrient
gradient or weighted by the square root of the sample size. As noted above, use of
information on feeding type, and sensitivity to other factors or other characteristics
of macroinvertebrate taxa could also improve the NBI.
For many bioassessment uses, accurate estimation of nutrients across the range
of observed concentrations is less important than detection of situations with very
high nutrients. Both nutrient indices and tests of nutrient indices may be designed
to predict and evaluate such conditions. As noted above, a large dataset is necessary
to provide a sufficient number of samples from sites with high nutrient-conditions
to develop a specific high-nutrient index.
Inherent variability between macroinvertebrate assemblages and nutrient
concentrations
Even with well-matched chemistry and macroinvertebrate data, and improved
methods of calculating NBIs, the NBI–nutrient relationships could be weakened
by a variety of factors including the influence of other variables on macroinvertebrate
assemblages such as habitat, hydrology, contaminants, pH, alkalinity,
Northeastern Naturalist Vol. 23, No. 4
R.J. Horwitz, A. Tuccillo, D.F. Charles, S. Neiffer, and T. Belton
2016
551
temperature, and oxygen concentrations, and by the tolerance of many taxa to
a range of nutrient conditions. As noted above, development of a larger data
set for estimating and testing NBIs would allow filtering or subsetting data to
limit or incorporate effects of other factors. Habitat quality may also play a role.
For example, Ashton et al. (2014) examined N associated with macroinvertebrate
communities in wadeable streams in Maryland, and found that an index
of biological integrity (IBI) was significantly associated with multiple nutrient
measures; however, the response of intolerant taxa was predominantly influenced
by a nutrient–forest-cover gradient, whereas habitat quality had a greater effect
on tolerant taxa. Thus, multiple lines of evidence may be needed to highlight
the effects of excessive nutrients in streams on macroinvertebrate communities
and taxa in Maryland, whose loss may not be reflected in metrics that form the
basis of biological criteria. Refinement of indicator taxa and development of a
nutrient-sensitive index is therefore warranted before water-quality thresholds
for aquatic life can be accurately quantified.
In addition, a recent USEPA expert workshop on the development of nutrient-
enrichment indicators in streams (USEPA 2014) noted that the indicators
most sensitive to nutrient pollution in streams and most predictive of impacts
to higher trophic levels include TN and TP, chlorophyll-a, percent visual coverage
of algae and in-stream macrophytes, and measures of algal assemblages
(e.g., diatoms and soft-bodied algae). However, the USEPA (2014) report also
acknowledged that most states routinely monitor only fish and macroinvertebrates,
and that the public recognizes the linkage between adverse effects on
fish and invertebrates and the impairment of aquatic life. The experts participating
in the workshop (USEPA 2014) concluded that commonly used fish and
macroinvertebrate indices may be less sensitive nutrient-pollution indicators
than other indicators (e.g., algae) and that refined and/or species-level metrics
for macroinvertebrates (specifically calibrated to be responsive to nutrient effects)
continue to show promise. However, there can be a significant temporal
lag between high nutrient-concentrations and adverse effects to some higher
trophic levels, making it difficult to proactively prevent nutrient impairment.
The experts identified the following research needs: (1) development of a single,
standardized, primary-producer indicator that integrates the productivity of
various producers into a single indicator; (2) improved understanding of the linkages
between nutrient measures, primary-producer measures, and higher trophic
levels that often are used to quantify aquatic-life impairment; (3) identification
of a minimum data-set size necessary to characterize stressor–response relationships;
and (4) development of regional stressor–response relationships linking
nutrient concentrations to algal-assemblage indicators, algal abundance, and
nutrient-sensitive macroinvertebrate indicators.
It should also be noted that correlations between N and P concentrations
are common among sites (e.g., Bae et al. 2011, Bedoya 2011), and responses
of macroinvertebrate taxa to the 2 nutrients may also be correlated. Both types
of correlations lead to inherent correlations in N and P indices and prevent
Northeastern Naturalist
552
R.J. Horwitz, A. Tuccillo, D.F. Charles, S. Neiffer, and T. Belton
2016 Vol. 23, No. 4
complete separation of responses to each nutrient individually. For example, if all
sites with high N also had high P and vice versa, there would be no way to determine
which nutrient was causing a response among macroinvertebrate taxa, even
if taxa were responding to single nutrients. Analogously, if some taxa are most
frequent at lower concentrations of both nutrients, absence of those taxa (which
would drive up the NBI) would not be useful to distinguish high-P from high-N
conditions (although presence would be informative). Smith et al. (2013) related
N endpoints to the NBI-P.
There were positive correlations between TP and NO3 optima and between the
corresponding tolerance values of taxa for both the NY- and NJ-based estimates.
For the NY estimates, the coefficient of determination (R2) between TP and NO3
nutrient optima was 0.58 and the R2 between tolerance values was 0.50. For the
NJ-based estimates (estimated from the main data set), the R2 between the 2 nutrient-
optima was 0.27 and the R2 between tolerance values was 0.26.
In all of the chemistry data sets, there were positive correlations between the N
and P values. These values are underestimates of the true correlation between nutrient
concentrations because the estimates are affected by measurement errors. For
example, for the diatom subset, the correlation between measured N and P values in
the NJ algal index (NJAI) was higher than the correlation between the nutrient concentrations
in the NJDEP main data set. This difference may reflect the combination
of data sources and methods for chemistry measurements for the latter data. The
positive correlations between nutrient responses and the correlations between nutrient
concentrations together resulted in positive correlations between NBI scores for
nitrogen and phosphorus at a site. These correlations should be considered in the
interpretation of NBI analyses. Forming a single index of nutrient response, such as
the eutrophication index of Hering et al. (2006), avoids the problem of separating
the responses.
Conclusion
The NBI approach approximated the same success in NJ waters as in the NY
study (Smith et al. 2007), as measured by the relationship between NBI scores and
nutrient concentrations. However, the robustness of these relationships is uncertain
because the relationships in both cases were measured using the same data
sets used to estimate nutrient responses of taxa. Estimation of nutrient optima and
tolerance values from different data than those used for calculation of NBI scores
produced significant relationships between NBIs or inferred nutrients and nutrient
concentrations, but with high variance (low correlation) around the relationships.
Furthermore, for individual taxa, estimates of nutrient-tolerance values from the
NY data were not highly correlated with the tolerance values estimated from NJ
data. These results indicate that macroinvertebrate assemblages are related to nutrient
values but the relationships are not yet sufficiently characterized to allow them
unambiguously to infer nutrient values from assemblage data.
Northeastern Naturalist Vol. 23, No. 4
R.J. Horwitz, A. Tuccillo, D.F. Charles, S. Neiffer, and T. Belton
2016
553
Acknowledgments
We thank Leigh Lager, Paul Morton, and Kevin Berry of NJDEP for providing macroinvertebrate
and chemistry data. A.J. Smith of NYSDEC provided estimates of tolerance
values and explained aspects of the NY study, Shane Moser assisted with statistical analyses
and preparation of figures, David Velinsky provided advice on selection and interpretation
of nutrient data, Stephanie Kroll reviewed the manuscript, and Scott Haig prepared the map.
This work was funded by a grant from the NDEP and by the Ruth Patrick Chair in Environmental
Science at ANSDU.
Literature Cited
Ashton, M.J., R.P. Morgan, and S. Stranko. 2014. Relations between macroinvertebrates,
nutrients, and water-quality criteria in wadeable streams of Maryland, USA. Environmental
Monitoring and Assessment 186(2):1167–82.
Bae, M.-J., Y. Kwon, S.-J. Hwang, T.-S. Chon, H.-J Yand, I.-S. Kwak, J.-H. Park, S.-A.
Ham, and Y.-S. Park. 2011. Relationships between three major stream assemblages and
their environmental factors in multiple spatial scales. Annals of Limnology 47:S91–
S105.
Barbour, M.T., J. Gerritsen, B.D. Snyder, and J.B. Stribling. 1999. Rapid bioassessment
protocols for use in streams and wadeable rivers: Periphyton, benthic macroinvertebrates,
and fish. Second edition. EPA 841-B-99-002. Available online at https://archive.
epa.gov/water/archive/web/html/index-14.html. Accessed 2 December 2016.
Bedoya, D., E.S. Manolakos, and V. Novotny. 2011. Characterization of biological responses
under different environmental conditions: A hierarchical modelling approach.
Ecological Modeling 222:532–545.
Biggs, B.J., S.N. Francoeur, A.D. Huryn, R. Young, C.J. Arbuckle, and C.R. Townsend.
2000. Trophic cascades in streams: Effects of nutrient enrichment on autotrophic and
consumer benthic communities under two different fish predation regimes. Canadian
Journal of Fisheries and Aquatic Sciences 57:1380–1394.
Birks, H.J.B., D.G. Frey, and E.S. Deevey. 1998. Review #1: Numerical tools in palaeolimnology—
progress, potentialities, and problems. Journal of Paleolimnology 20:307–322.
Bryce, S.A., and R.M. Hughes. 2003. Variable assemblage-responses to multiple disturbance
gradients: Case studies in Oregon and Appalachia, USA. Pp. 539–560, In T.P.
Simon (Ed.). Biological Response Signatures: Indicator Patterns Using Aquatic Communities.
CRC Press, Boca Raton, FL. 576 pp.
Camargo, J.A., A. Alonso, and M. De La Puente. 2004. Multimetric assessment of nutrient
enrichment in impounded rivers based on benthic macroinvertebrates. Environmental
Monitoring and Assessment 96:233.
Charles, D.F., A.P. Tuccillo, and T.J. Belton. 2010. Diatoms and the biological-condition
gradient in New Jersey rivers and streams: A basis for developing nutrient guidance
levels. Patrick Center for Environmental Research Report 10-03 for NJDEP. Available
online at http://www.state.nj.us/dep/dsr/nutrient/bcg-talu-final-report.pdf. Accessed 2
December 2016.
Flinders, C.A., R.J. Horwitz, and T.J. Belton. 2008. Relationship of fish and macroinvertebrate
communities in the mid-Atlantic uplands: Implications for integrated assessments.
Ecological Indicators 8:588–598.
Hausmann, S., D. Charles, T. Belton, and J. Gerritsen. 2016. A diatom-based biologicalcondition
gradient (BCG) approach for assessing impairment and developing nutrient
criteria for streams. Science of the Total Environment 562:914–927.
Northeastern Naturalist
554
R.J. Horwitz, A. Tuccillo, D.F. Charles, S. Neiffer, and T. Belton
2016 Vol. 23, No. 4
Hering, D., R.K. Johnson, S. Kramm, S. Schmutz, K. Szoszkeiwicz, and P.F.M. Verdonschot.
2006. Assessment of European streams with diatoms, macrophytes, macroinvertebrates,
and fish: A comparative metric-based analysis of organism response to stress.
Freshwater Biology 51:1757–1785.
New Jersey Department of Environmental Protection. (NJDEP). 2007. Development of the
New Jersey high gradient macroinvertebrate index (HGMI). Final Report. Available
online at http://www.state.nj.us/dep/wms/bfbm/download/HGMI_report.pdf. Accessed
2 December 2016.
NJDEP. 2012. Ambient biomonitoring network, Northwest Region, round 4: Benthic macroinvertebrate
data, Vol. 1 of 2. Division of Water Monitoring and Standards Technical
Report. Available online at http://www.state.nj.us/dep/wms/bfbm/amnetRnd4.html. Accessed
12 December 2016.
Pilière, H., A.M. Schipper, A.M. Breure, L. Posthuma, D. de Zwart, S.D. Dyer, and M.A.J.
Huijbregts. 2014. Comparing responses of freshwater fish and invertebrate community
integrity along multiple environmental gradients. Ecological Indicators 43:215–226.
Ponader, K.C., D.F. Charles, D.R. Winter, and T. J. Belton. 2007. Diatom-based TP and TN
inference models and indices for monitoring nutrient enrichment of NJ streams. Ecological
Indicators 7:79–93.
Smith, A.J., R.W. Bode, and G.S. Kleppel. 2007. A nutrient biotic index (NBI) for use with
benthic macroinvertebrate communities. Ecological Indicators 7:371–386.
Smith, A.J., R.L. Thomas, J.K. Nolan, D.J. Velinsky, S. Klein, and B.T. Duffy. 2013. Regional
nutrient-thresholds in wadeable streams of New York State protective of aquatic
life. Ecological Indicators 29:455–67.
Spieles, D.J., and W. J. Mitsch. 2000. Macroinvertebrate community structure in high- and
low-nutrient constructed wetlands. Wetlands 20:716.
ter Braak, C.J.F., and S. Juggins. 1993. Weighted-averaging partial least squares regression
(WA-PLS): An improved method for reconstructing environmental variables from species
assemblages. Hydrobiologia 269/270:485–502.
US Environmental Protection Agency (USEPA). 2014. USEPA expert workshop: Nutrient
enrichment indicators in streams proceedings 16–18 April 2013. Office of Water EPA-
822-R-14-004. Available online at https://www.epa.gov/sites/production/files/2013-09/
documents/indicatorsworkshop.pdf.
Yoder, C.O., and J.E. DeShon. 2003. Using biological-response signatures within a framework
of multiple indicators to assess and diagnose causes and sources of impairments
to aquatic assemblages in selected Ohio Rivers and streams. Pp. 23–55, In T.P. Simon
(Ed.). Biological Response Signatures. CRC Press, Boca Raton, FL. 600 pp.
Yoder, C.O., and E.T. Rankin. 1995. Biological-response signatures and the area of degradation
value: New tools for interpreting multi-metric data. Pp. 263–286, In W.S. Davis
and T.P. Simon (Eds.). Biological Assessment and Criteria: Tools for Water-Resource
Planning And Decision Making. Lewis Publishers, Boca Raton, FL.