Simulating Potential Population Growth of Wild Pig,
Sus scrofa, in Texas
Janell M. Mellish, Aaron Sumrall, Tyler A. Campbell, Bret A. Collier,
William H. Neill, Billy Higginbotham, and Roel R. Lopez
Southeastern Naturalist, Volume 13, Issue 2 (2014): 367–376
Full-text pdf (Accessible only to subscribers.To subscribe click here.)
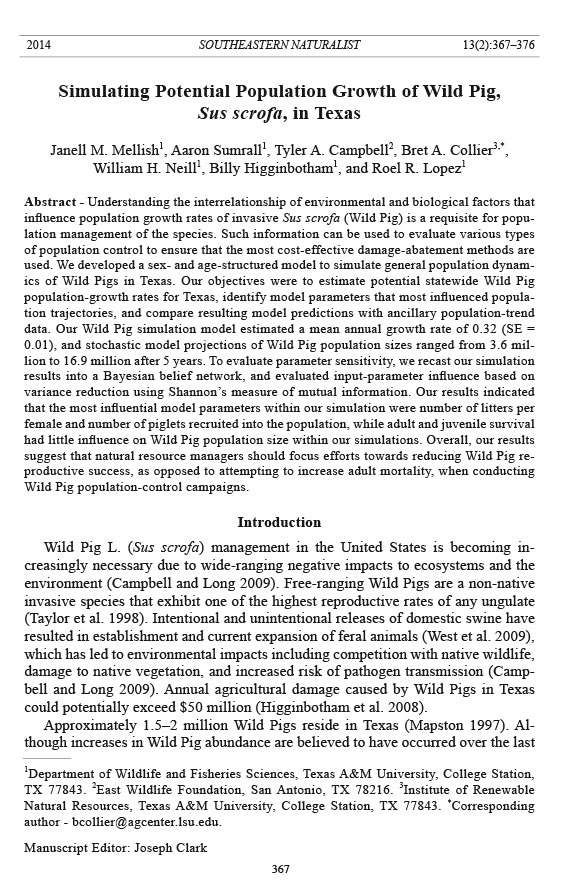
Southeastern Naturalist
367
J.M. Mellish, et al.
22001144 SOUTHEASTERN NATURALIST 1V3o(2l.) :1336,7 N–3o7. 62
Simulating Potential Population Growth of Wild Pig,
Sus scrofa, in Texas
Janell M. Mellish1, Aaron Sumrall1, Tyler A. Campbell2, Bret A. Collier3,*,
William H. Neill1, Billy Higginbotham1, and Roel R. Lopez1
Abstract - Understanding the interrelationship of environmental and biological factors that
influence population growth rates of invasive Sus scrofa (Wild Pig) is a requisite for population
management of the species. Such information can be used to evaluate various types
of population control to ensure that the most cost-effective damage-abatement methods are
used. We developed a sex- and age-structured model to simulate general population dynamics
of Wild Pigs in Texas. Our objectives were to estimate potential statewide Wild Pig
population-growth rates for Texas, identify model parameters that most influenced population
trajectories, and compare resulting model predictions with ancillary population-trend
data. Our Wild Pig simulation model estimated a mean annual growth rate of 0.32 (SE =
0.01), and stochastic model projections of Wild Pig population sizes ranged from 3.6 million
to 16.9 million after 5 years. To evaluate parameter sensitivity, we recast our simulation
results into a Bayesian belief network, and evaluated input-parameter influence based on
variance reduction using Shannon’s measure of mutual information. Our results indicated
that the most influential model parameters within our simulation were number of litters per
female and number of piglets recruited into the population, while adult and juvenile survival
had little influence on Wild Pig population size within our simulations. Overall, our results
suggest that natural resource managers should focus efforts towards reducing Wild Pig reproductive
success, as opposed to attempting to increase adult mortality, when conducting
Wild Pig population-control campaigns.
Introduction
Wild Pig L. (Sus scrofa) management in the United States is becoming increasingly
necessary due to wide-ranging negative impacts to ecosystems and the
environment (Campbell and Long 2009). Free-ranging Wild Pigs are a non-native
invasive species that exhibit one of the highest reproductive rates of any ungulate
(Taylor et al. 1998). Intentional and unintentional releases of domestic swine have
resulted in establishment and current expansion of feral animals (West et al. 2009),
which has led to environmental impacts including competition with native wildlife,
damage to native vegetation, and increased risk of pathogen transmission (Campbell
and Long 2009). Annual agricultural damage caused by Wild Pigs in Texas
could potentially exceed $50 million (Higginbotham et al. 2008).
Approximately 1.5–2 million Wild Pigs reside in Texas (Mapston 1997). Although
increases in Wild Pig abundance are believed to have occurred over the last
1Department of Wildlife and Fisheries Sciences, Texas A&M University, College Station,
TX 77843. 2East Wildlife Foundation, San Antonio, TX 78216. 3Institute of Renewable
Natural Resources, Texas A&M University, College Station, TX 77843. *Corresponding
author - bcollier@agcenter.lsu.edu.
Manuscript Editor: Joseph Clark
Southeastern Naturalist
J.M. Mellish, et al.
2014 Vol. 13, No. 2
368
20 years, population trends in Wild Pigs are poorly understood because reliable
survey methods for Wild Pigs do not exist (Reidy et al. 2011) and the relationship
between population growth and demography is understudied (Adams et al. 2005).
Our objectives were to simulate likely Wild Pig population dynamics, identify
model parameters most influential on population trajectories, and compare model
estimates with statewide harvest trend data. In so doing, our goal was to test the
population model with ancillary data, estimate growth rates, and identify control
methods most effective at limiting that population growth.
Methods
Model structure and parameterization
We used a stochastic age- and sex-structured model written in R (R Core Development
Team 2004) for our simulations (Fig. 1; see Supplemental R code, available
online at https://www.eaglehill.us/SENAonline/suppl-files/s13-2-2055-Collier-s1
and, for BioOne subscribers, at http://dx.doi.org/10.1656/S2057.s1). The model
tracked, on a semi-annual basis, population size and demography of both sexes
(male, female) in 3 mutually exclusive age classes: piglets (P; birth to 6 months
old), juveniles (J; 6 months to 1 yr), and adults (A; ≥1 year old). Optimally, ageand
sex-specific population-demographic estimates would be available for the
population of interest in Texas, but this information is unavailable for Wild Pigs in
most states because research efforts focused on Wild Pig demography are limited
(Ditchkoff et al. 2012). Thus, we conducted a literature review and compiled a suite
of demographic-parameter estimates, from which we based age-specific estimates
for use in our simulations (Table 1). We used model expressions and incorporated
demographic variation using binomial or Poisson random variates within the simu-
Figure 1. Conceptual model for simulation of Wild Pig population dynamics in Texas.
Southeastern Naturalist
369
J.M. Mellish, et al.
2014 Vol. 13, No. 2
lation model following Phillips and White (2003). We conducted 1000 simulations
using random combinations of demographic parameters drawn from a uniform
distribution bounded by the minimum and maximum estimated values we garnered
from the literature.
Statewide population data
Three separate, statewide data sources were used to independently estimate
trends in harvest of Wild Pigs in Texas, and to evaluate the model population
trajectories from our study. We estimated growth rate from these harvest-trend
data sets and compared those results to our model projections. The first data set
was based on aerial-eradication permits granted during 2005 to 2008 to private
landowners by Texas Parks and Wildlife Department (TPWD). The second data
set was based on the number of live-trapped Wild Pigs in Texas sold to processing
plants for human consumption, which we obtained for the period 2004–2008
from the Texas Department of Agriculture. Finally, we obtained 2000–2008 data
Table 1. Baseline population estimates (mean [M], low [L], high [H]) for parameterizing the Wild
Pig simulation model.
Age Mortality Sex ratio Reproductive Litter Litters/ Juvenile:adult Initial
class rate1 (m:f)2 age3 size4 year5 ratio6 abundance7
Piglet 0.14 (M) 0.5 391,111
0.16 (L)
0.12 (H)
Juvenile 0.15 (M) 0.5 195,556
0.16 (L)
0.14 (H)
Adult 0.08 (M) 2.0 1,246,666
0.1 (L)
0.06 (H)
Combined 0.50 (M) 6 months (M) 5.64 (M) 1.50 (M) 1:2.0 (M) 1,833,333 (M)
0.48 (L) 7 months (L) 5.34 (L) 1.33 (L) 1:1.6 (L) 1,666,666 (L)
0.52 (H) 5 months (H) 5.94 (H) 1.67 (H) 1:2.5 (H) 2,000,000 (H)
1Data sources: Adkins and Harveson (2007), Baber and Coblentz (1987), Barrett (1978), Diong
(1982), Gabor et al. (1999), Hayes et al. (2009) Saunders (1993), and Wood and Brenneman (1977).
2Data sources: Adkins and Harveson (2007), Baber and Coblentz (1987), Beldon and Frankenberger
(1990), Diong (1982), Duncan (1974), Gabor et al. (1999), Hagen and Kephart (1980), Pine
and Gerdes (1973), Saunders (1993), Sweeney et al. (1979), Taylor et al. (1998), and Wood and
Brenneman (1977).
3Data sources: Beldon and Frankenberger (1990), Diong (1982), Duncan (1974), Pine and Gerdes
(1973), Springer (1977), Taylor et al. (1998), and Wood and Brenneman (1977).
4Data sources: Baber and Coblentz (1987), Barrett (1978), Beldon and Frankenberger (1990), Diong
(1982), Duncan (1974), Gabor et al. (1999), Graves (1984), Hagen and Kephart (1980), Hellgren
(1993), Pine and Gerdes (1973), Saunders (1993), Springer (1977), Sweeney et al. (1979), Taylor et
al. (1998), and Wood and Brenneman (1977).
5Data sources: Adkins and Harveson (2007), Baber and Coblentz (1987), Barrett (1978), Diong
(1982), Pine and Gerdes (1973), Rollins et al. (2007), Taylor et al. (1998), and West et al. (2009).
6Data sources: Adkins and Harveson (2007), Diong (1982), Duncan (1974), and Ga bor et al. (1999).
7Data sources: Rollins et al. (2007), and West et al. (2009).
Southeastern Naturalist
J.M. Mellish, et al.
2014 Vol. 13, No. 2
370
based on aerial gunning and other damage-control activities (e.g., trapping, ground
shooting) conducted as part of the Wild Pig damage-control program of the United
States Department of Agriculture, Animal and Plant Health Inspection Services’
Wildlife Services (WS) program in Texas. We had to assume that relative effort
for each data set was consistent among years, and for plotting we scaled all time to
start at year 1 for consistency.
Graphical causal model
We developed a Bayesian belief network (BBN) implemented in Netica (Norsys
Software Corp.) to assist with model evaluation, as our BBN represents a causal
model of the biological system under study (Fig. 2; Marcot et al. 2006, Pearl 1988).
Bayesian belief networks use probabilistic expressions to describe relationships between
model components conditional on knowledge (evidence) contained in other
variables within the system (Peterson and Evans 2003). As belief can never be >1,
the allocation of belief within each node is used to show certainty (or uncertainty)
associated with the state of each biological variable.
Model output consisted of input parameters and intermediate model-based estimates
with ending population-simulation results output as a case file (database
of findings; Lee and Rieman 1997, Peterson and Evans 2003). Within a BBN,
parameters work in concert with each other (i.e., a change in one parameter could
influence several model parameters). Thus, to evaluate parameter sensitivity, we
used the internal sensitivity functions in Netica (Marcot et al. 2001) to calculate
the reduction in variance using Shannon’s measure of mutual information (Pearl
1988). Unlike typical sensitivity analyses where a single parameter is varied ±
some fixed percentage and compared to an arbitrary baseline, sensitivity analysis
in a BBN evaluates changes to the probability distribution of the response variable
based on changes to the probability distribution of causally related model nodes
(Marcot et al. 2001). Sensitivity was expressed as the percent reduction in variance
of the query variable (Q) or response variable of interest given the specification
in the findings variables (F) or variables conditionally related to the query variable.
We relate variance-reduction estimates from sensitivity analysis with reduced
uncertainty in the predicted model outcome (Pearl 1988). Predicted variation was
exhibited as the spread of the posterior probability estimates across the possible
outcome states.
Results
Our Wild Pig model estimated that mean annual growth rate was 0.32 (SE =
0.01). Based on the range of input parameters used in our modeling exercise, simulated
mean population size increased over a 5-year period from 1.8 million
Wild Pigs to approximately 9.1 million. However, stochastic model projections
of Wild Pig population growth ranged from 3.6 million to 16.9 million after 5
years. Based on our sensitivity analysis, parameters identified in our graphical
causal model indicated that the number of piglets surviving to recruitment (variance
reduction of 85.6%) and number of litters per female (variance reduction of 58%)
Southeastern Naturalist
371
J.M. Mellish, et al.
2014 Vol. 13, No. 2
Figure 2. Predicted
range of residual
population size (expressed
as a percentage
of total
population size) of
Wild Pigs in Texas
conditional on different
specifications
of knowledge
regarding the number
of litters per
female (expressed
as proportion). As
an example, the belief
histogram for
node Population
Size (Yr 5) shows
the predicted belief
(probability) that the
residual population
size at year 5 will be
within a given size
class, conditional
on knowledge of the
number of litters per
female (expressed
as proportion) using
a scenario where no
updating of knowledge
has occurred
(2a), a scenario
where knowledge
is added assuming
the number of litters
per female is within
the minimum range
based on the simulation
input values
(2b), and a scenario
where knowledge
is added assuming
the number of litters
per female is within
the maximum range
based on the simulation
input values
(2c).
Southeastern Naturalist
J.M. Mellish, et al.
2014 Vol. 13, No. 2
372
were the primary population parameters driving growth. For example, as shown
in Figure 2, subtle changes in the number of litters per female (changing from a
maximum of 0.29 to 0.35) can cause significant changes in the predicted population
size. We found only a limited impact of piglet survival (variance reduction of
1.72%) and sub-adult population size (variance reduction of 1.0%) on sensitivity of
population predictions, and no other parameters within our model reduced variance
>1%. Additionally, our sensitivity analysis indicated that neither adult nor juvenile
survival rate had any significant impact on Wild Pig population projections over the
course of our simulations. Annual intrinsic growth rates from processing plant numbers,
TPWD’s aerial removal data, and removal data from Texas WS varied from
0.19–0.25, with a mean of 0.21 (Fig. 3). Observed increases in statewide Wild Pig
population-trend data were significantly less than population trajectories simulated
using our model (r = 0.32).
Discussion
Simulation models involving free-ranging Sus scrofa have been constructed
to assess disease-control efforts in Australia (Pech and Hone 1988), harvesting
strategies in Italy (Focardi et al. 1996), management actions in Switzerland
(Neet 1995), and the influence of environmental conditions in Spain (Uzal and
Nores 2004). As with any modeling exercise, the validity of the model output is
dependent upon the quality of parameters used. Our model, which used available
Figure 3. Intrinsic growth rates estimated based on Wild Pig processing plant numbers,
Texas Parks and Wildlife Department’s aerial removal data, and removal data from United
States Department of Agriculture, Animal and Plant Health Inspection Services Wildlife
Services program in Texas.
Southeastern Naturalist
373
J.M. Mellish, et al.
2014 Vol. 13, No. 2
estimates from the literature and encompassed a variety of regions and environments,
suggests that annual population growth rate for Wild Pigs in Texas is
approximately 0.32. The model’s projection of a growing population is consistent
with anecdotal reports of increases in Wild Pig damage and range expansion
throughout Texas and the southeastern United States, but the rate generated is
less than that indicated by available data on the recent growth trends in Wild Pigs
harvested in Texas. If the processing and removal data reflects a consistent effort
and efficiency of harvest over the years and thus accurately follows the trend of
the actual population size of Feral Pigs, then our model would need to be adjusted
in some manner to better reflect all of the factors that might in reality be affecting
population growth of Feral Pigs. On the other hand, our model may indicate that
over the years, the removal efforts have been getting less effective and are capturing
a smaller and smaller percentage of the actual population. If that is the case,
then we may be seeing a much larger increase in the number of Wild Pigs than
might be expected based on the limited available data. Specifically, our population
projections indicated that Wild Pig population size could, assuming our input
parameters were accurate and our model structure appropriate, quintuple within a
5-year period.
If Wild Pig population parameters are more closely represented by the higher
parameter estimates, then we expect the population after 5 years to approach 16
million, and if population parameters are more closely represented by the lower
range of parameter estimates, then we expect the population after 5 years to be
approximately 3 million. Obviously, no population is going to have consistent
population parameters over a long time frame, so it is likely that the mostlikely
population growth will be intermediate to the extremes. We note that in all
projected population-growth estimates, we did not account for density-dependence
effects to determine the potential rate of growth of Wild Pigs in Texas. The
functional form for dependence, at what scale density-dependence operates, or
what vital rates density dependence would impact are unknown (Ditchkoff et al.
2012). However, density-dependence processes will likely play a role in Wild Pig
population regulation as populations continue to expand, likely manifesting its
impact through reductions in population-level recruitment.
Our sensitivity analysis, when couched in a graphical causal model, provides
us with the ability to evaluate which parameters are most significantly influencing
population growth predictions without having to hold other parameters static in
concert with additional simulation runs. We identified 2 general parameter categories
that should be addressed by managers. First, we found that efforts towards
reducing survival of adults and juvenile Wild Pigs by managers will have poor
success. Attempts to influence survival, of adults, while intuitive to some, has had
little success at large scales for several species (Alisauskas et al. 2011, Brown et
al. 2000); thus, it is not surprising that localized increases in adult mortality have
little impact on range-wide dynamics (Hanson et al. 2009). However, in situations
where short-term damage abatement is of primary concern (e.g., agricultural
loss), then direct removal of Wild Pigs may have short-term and immediate local
Southeastern Naturalist
J.M. Mellish, et al.
2014 Vol. 13, No. 2
374
impacts but contribute little to population-level reduction. Second, our results indicate
that management should focus efforts on methods to reduce reproduction,
specifically the number of litters per year and piglet survival. Work in developing a
fertility-control agent for Wild Pigs in a disease-management context is underway
(Campbell et al. 2010, Sanders et al. 2011). However, at present there is no oral
fertility-control agent registered by the Environmental Protection Agency for use
in Wild Pig populations.
We hypothesize that these targeted and intensive management strategies can
result in population reductions at the scale that management is applied. In Texas,
droughts are frequent and when they occur, Wild Pig fecundity is naturally reduced
(Taylor et al. 1998). Furthermore, Wild Pigs are often concentrated near sources of
free water during drought, and food resources are scarce then. Both of these conditions
facilitate efficient lethal control at local scales (Muir and McEwen 2007).
However, given our modeling results, it is likely that the use of a variety of control
methods deployed in a purposeful and integrated fashion (Campbell and Long
2009) will be required to slow growth of Wild Pig populations. Our graphical causal
model, while admittedly coarse, will benefit managers by simplifying interpretation
of model results, allowing for updating when additional data are collected, and for
testing of alternative structures (Marcot et al. 2001).
Acknowledgments
We thank the Texas A&M Institute of Renewable Natural Resources and Department of
Wildlife and Fisheries Science at Texas A&M University for financial and logistical support
to conduct research.
Literature Cited
Adams, C.E., B.J. Higginbotham, D. Rollins, R.B. Taylor, R. Skiles, M. Mapston, and S.
Turman. 2005. Regional perspectives and opportunities for Feral Hog management in
Texas. Wildlife Society Bulletin 33:1312–1320.
Adkins R.N., and L.A. Harveson. 2007. Demographic and spatial characteristics of Feral
Hogs in the Chihuahuan Desert, Texas. Human–Wildlife Interactions 1:152–160.
Alisauskas, R.T., R.F. Rockwell, K.W. Dufour, E.G. Cooch, G. Zimmerman, K.L. Drake,
J.O. Leafloor, T.J. Moser and E.T. Reed. 2011. Harvest, survival, and abundance of
mid-continent Lesser Snow Geese relative to population reduction efforts. Wildlife
Monographs 179.
Baber, D.W., and B.E. Coblentz. 1987. Diet, nutrition, and conception in Feral Pigs on
Santa Catalina Island. Journal of Wildlife Management 51:306–317.
Barrett, R.H. 1978. The Feral Hog on the Dye Creek Ranch, California. Hilgardia 46:283–
355.
Belden, R.C., and W.B. Frankenberger. 1990. Biology of a Feral Hog population in southcentral
Florida. Proceedings Annual Conference Southeast Association Fish Wildlife
Agencies 44:231–242.
Brown, T.L., D.J. Decker, S.J. Riley, J.W. Ench, T.B. Lauber, P.D. Curtis, and G.F. Mattfeld.
2000. The future of hunting as a mechanism to control White-tailed Deer populations.
Wildlife Society Bulletin 28:797–807.
Southeastern Naturalist
375
J.M. Mellish, et al.
2014 Vol. 13, No. 2
Campbell, T.A., and D.B. Long. 2009. Feral Swine damage and damage management in
forested ecosystems. Forest Ecology and Management 257:2319–232 6.
Campbell, T.A., M.R. Garcia, L.A. Miller, M.A. Ramirez, D.B. Long, J. Marchand, and F.
Hill. 2010. Immunocontraception of male Feral Swine with a recombinant GnRH vaccine.
Journal of Swine Health and Production 18:1 18–124.
Diong, C.H. 1982. Population biology and management of the Feral Pig (Sus scrofa L.) in
Kipahula Valley, Maui. Ph.D. Dissertation. University of Hawaii, Honolulu, HI.
Ditchkoff, S.S., D.B. Jolley, B.D. Sparklin, L.B. Hanson, M.S. Mitchell, and J.B. Grand.
2012. Reproduction in a population of Wild Pigs (Sus scrofa) subjected to lethal control.
Journal of Wildlife Management 76:1235–1240.
Duncan, R.W. 1974. Reproductive biology of the European Wild Hog (Sus scrofa) in the
Great Smoky Mountains National Park. M.Sc. Thesis. University of Tennessee, Knoxville,
TN.
Focardi, S., S. Toso, and E. Pecchioli. 1996. The population modeling of Fallow Deer and
Wild Boar in a Mediterranean ecosystem. Forest Ecology and Manag ement 88:7–14.
Gabor, T.M., E.C. Hellgren, R.A. Van Den Bussche, and N.J. Silvy. 1999. Demography,
sociospatial behaviour, and genetics of Feral Pigs in a semi-arid environment. Journal
of Zoology-London 247:311–322.
Graves, H.B. 1984. Behaviour and ecology of Wild and Feral Swine (Sus scrofa). Journal
of Animal Science 58:482–493.
Hagen D.R., and K.B. Kephart. 1980. Reproduction in Feral Swine. I. Comparison of ovulatory
rate and litter size. Biology of Reproduction 22:550–552.
Hanson, L.B., M.S. Mitchell, J.B. Grand, D.B. Jolley, B.D. Sparklin, and S.S. Ditchkoff.
2009. Effect of experimental manipulation on survival and recruitment of Feral Pigs.
Wildlife Research 36:185–191.
Hayes, R., S. Riffell, R. Minnis, and B. Holder. 2009. Survival and habitat use of Feral Hogs
in Mississippi. Southeastern Naturalist. 8:411–426.
Hellgren, E.C. 1993. Biology of Feral Hogs (Sus scrofa) in Texas. Pp. 50–58, In C.W. Hanselka
and J.F. Cadenhead (Eds.). Feral Swine: A Compendium for Resource Managers.
Texas Agricultural Extension Service, Kerrville, TX.
Higginbotham, B., G. Clary, L. Hysmith, and M. Bodenchuk. 2008. Statewide Feral Hog
abatement pilot project, 2006–2007. Texas AgriLife Extension Service. Available online
at http://feralhogs.tamu.edu/files/2010/05/06-07-Feral-Hog-Abatement-Pilot-Project.
pdf. Accessed 7 December 2011.
Lee, D.C., and B.E. Rieman. 1997. Population viability assessment of salmonids by probabilistic
networks. North American Journal of Fisheries Management 17:1144–1157.
Mapston, M.E. 1997. Feral Hog control in Texas. Pp. 2.1–2.3, In K.L. Schmitz (Ed). Proceedings:
National Feral Swine Symposium. USDA, Animal and Plant Health Inspection
Services, Riverdale, MD.
Marcot, B.G., R.S. Holthausen, M.G. Raphael, M.M. Rowland, and M.J. Wisdon. 2001.
Using Bayesian belief networks to evaluate fish and wildlife population viability under
land-management alternatives from an environmental impact statement. Forest Ecology
and Management 153:29–42.
Marcot, B.G., J.D. Steventon, G.L. Sutherland, and R.K. McCann. 2006. Guidelines for
developing and updating belief networks applied to ecological modeling and conservation.
Canadian Journal of Forest Research 36:3063–3074.
Muir, T.J., and G. McEwen. 2007. Methods and strategies for managing Feral Hog damage
in grain production areas in central Texas. Pp. 445–450, In G.W. Witmer, W.C. Pitt,
and K.A. Fagerstone (Eds.). Managing Vertebrate Invasive Species: Proceedings of an
Southeastern Naturalist
J.M. Mellish, et al.
2014 Vol. 13, No. 2
376
International Symposium. USDA/APHIS/WS National Wildlife Research Center, Fort
Collins, CO. 481 pp.
Neet, C.R. 1995. Population dynamics and management of Sus scrofa in western Switzerland:
A statistical modeling approach. IBEX J.M.E. 3:188−191.
Pearl, J. 1988. Probabilistic reasoning in intelligent systems: Networks of plausible inference.
Morgan-Kaufmann, San Mateo, CA.
Pech, R.P., and J. Hone. 1988. A model of the dynamics and control of an outbreak of foot
and mouth disease in Feral Pigs in Australia. Journal of Applied Ecology 25:63−77.
Peterson, J.T., and J.W. Evans. 2003. Quantitative decision analysis for sport-fisheries management.
Fisheries 28:10–21.
Pine, D.S., and G.L. Gerdes. 1973. Wild Pigs in Monterey County, California. California
Fish and Game 59:126−137.
Phillips, G.E., and G.C. White. 2003. Pronghorn population response to Coyote control:
Modeling and management. Wildlife Society Bulletin 31:1162–1175.
R Core Development Team. 2014. R: A language for environmental and statistical computing.
R Foundation for Statistical Computing. ISBN 3-900051-00-3. Available online at
http://www.R-project.org. Accessed 15 January 2014.
Reidy, M.M., T.A. Campbell, and D.G. Hewitt. 2011. A mark–recapture technique for
monitoring Feral Swine populations. Rangeland Ecology and Management 64:316–318.
Rollins, D., B.J. Higginbotham, K.A. Cearley, and R.N. Wilkins. 2007. Appreciating Feral
Hogs: Extension education for diverse stakeholders in Texas. Human-Wildlife Interactions
1:192−198.
Sanders, D.L., F. Xie, R.E. Mauldin, L.A. Miller, M.R. Garcia, R.W. DeYoung, D.B. Long,
and T.A. Campbell. 2011. Efficacy of ERL-4221 as an ovotoxin for Feral Swine. Wildlife
Research 38:168–172.
Saunders, G. 1993. The demography of Feral Pigs (Sus Scrofa) in Kosciusko National Park,
New South Wales. Wildlife Research 20:559−569.
Springer, M.D. 1977. Ecologic and economic aspects of Wild Hogs in Texas. Pp. 37−46, In
G.W. Wood (Ed.). Research and Management of Wild Hog Populations. The Belle W.
Baruch Forest Science Institute of Clemson University, Georgetown, SC.
Sweeney, J.M., J.R. Sweeney, and E.E. Provost. 1979. Reproductive biology of a Feral Hog
population. Journal of Wildlife Management 43:555−559.
Taylor, R.B., E.C. Hellgren, T.M. Gabor, and L.M. Ilse. 1998. Reproduction of Feral Pigs
in southern Texas. Journal of Mammalogy 79:1325–1331.
Uzal, A., and C. Nores. 2004. Endogenous and exogenous constraints in the population
changes of Wild Boar (Sus scrofa Linneaus, 1758). Galemys 16:83−98.
West, B.C., A.L. Cooper, and J.B. Armstrong. 2009. Managing Wild Pigs: A technical guide.
Human Wildlife Interactions Monograph 1:1–55.
Wood, G.W., and R.E. Brenneman. 1977. Research and management of Feral Hogs on
Hobcaw Barony. Pp. 23–36, In G.W. Wood (Ed.). Research and Management of Wild
Hog Populations. The Belle W. Baruch Forest Science Institute of Clemson University,
Georgetown, SC.