Genetic Population Structure and Accuracy of
Morphological Assessment in Alosa aestivalis (Blueback
Herring) and A. pseudoharengus (Alewife)
Christopher Kan, John Lichter, and Vladimir Douhovnikoff
Northeastern Naturalist, Volume 24, Issue 4 (2017): 482–498
Full-text pdf (Accessible only to subscribers. To subscribe click here.)
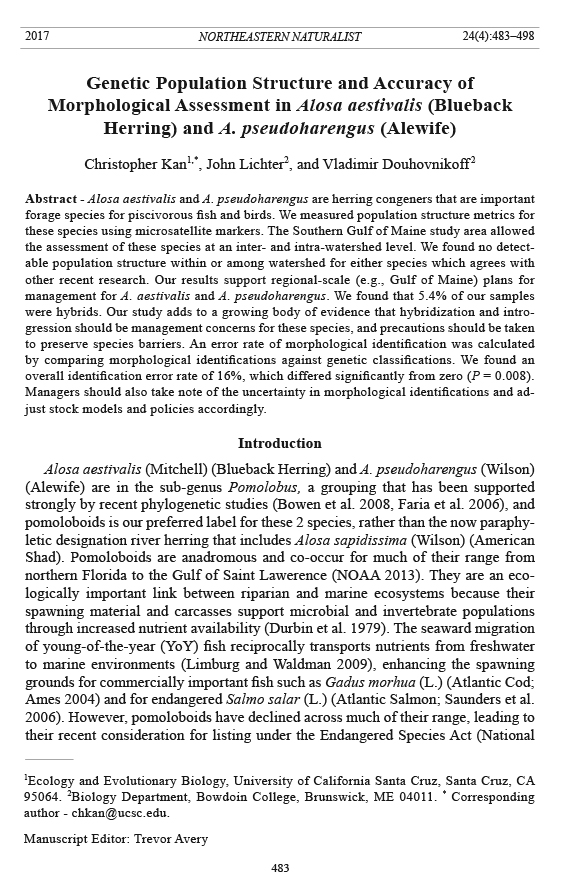
Access Journal Content
Open access browsing of table of contents and abstract pages. Full text pdfs available for download for subscribers.
Current Issue: Vol. 30 (3)

Check out NENA's latest Monograph:
Monograph 22
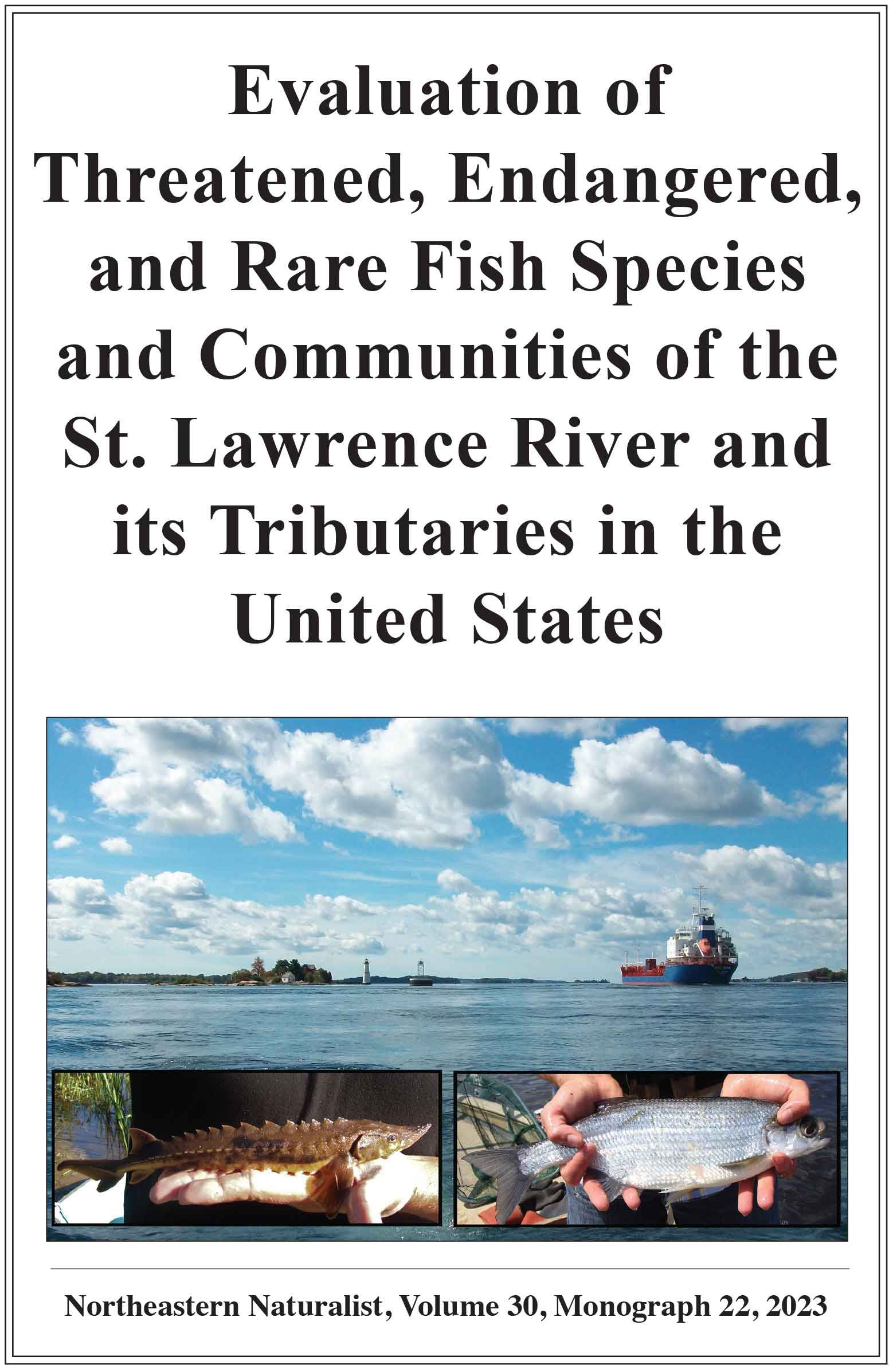
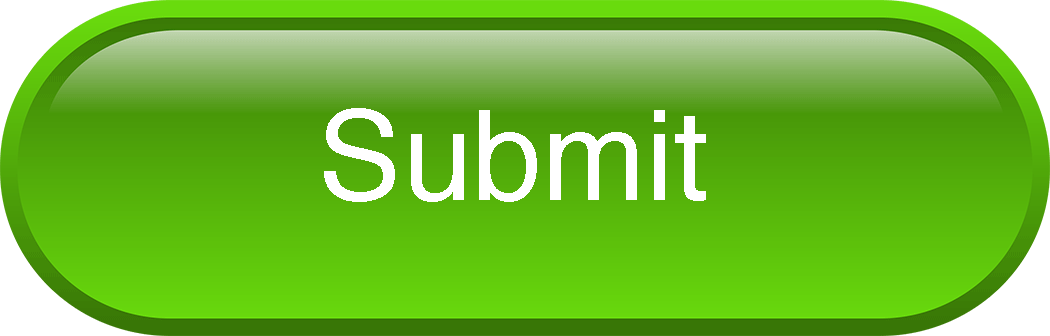


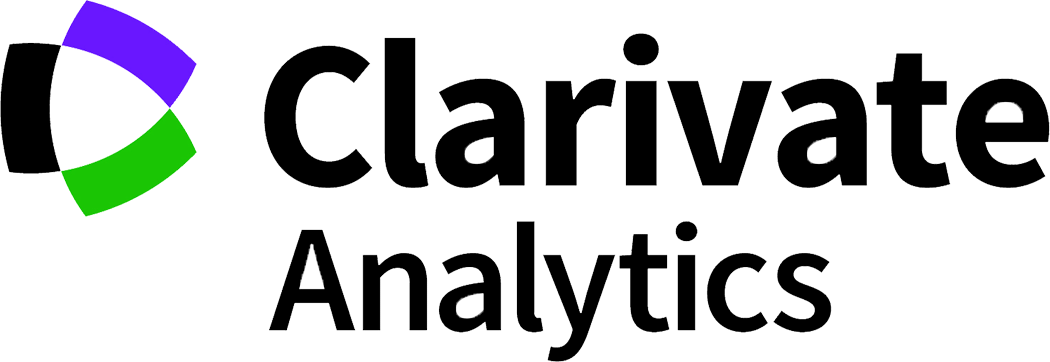
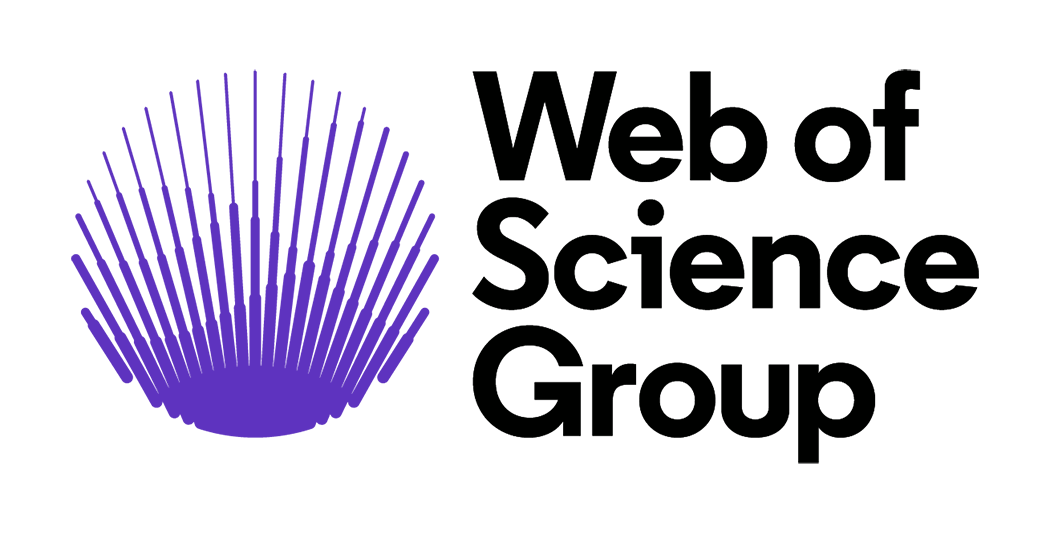
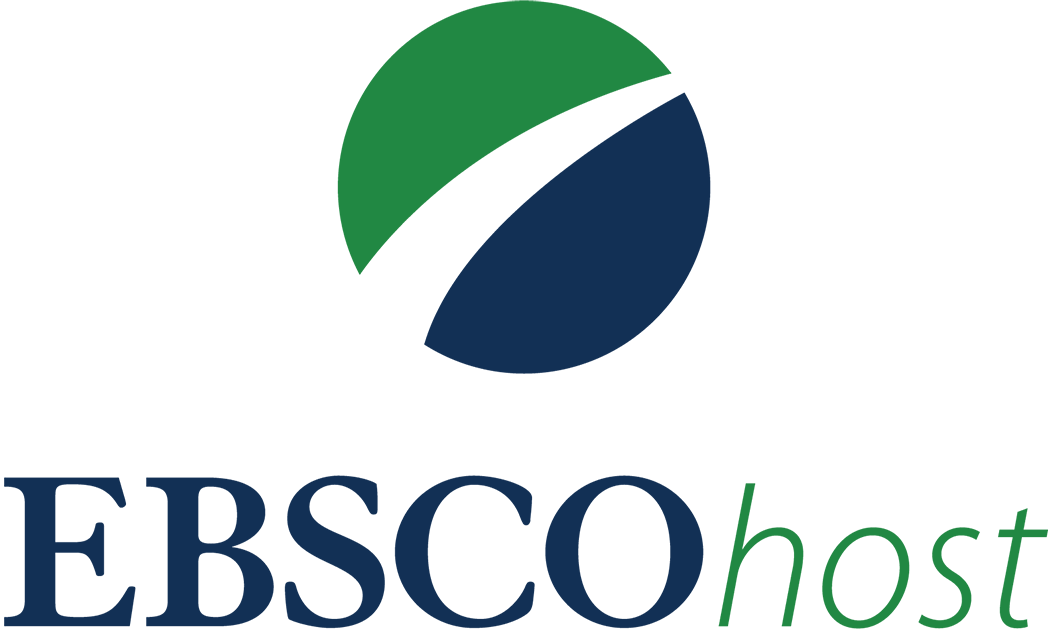
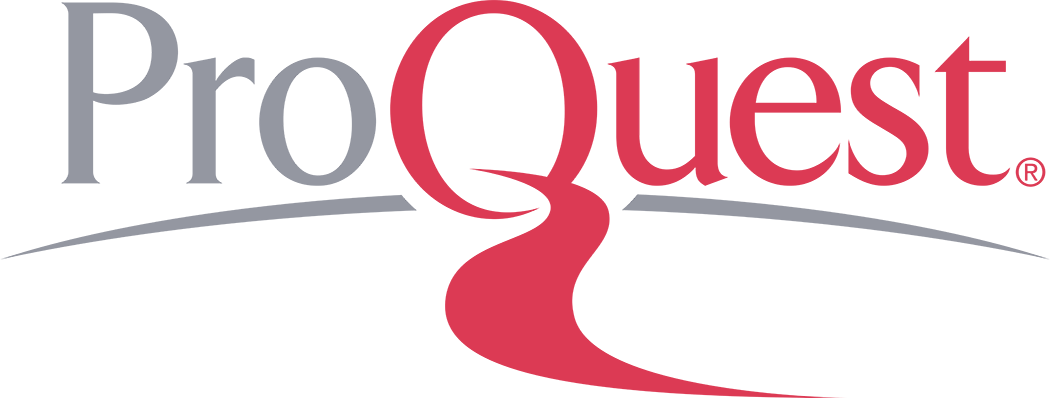
Northeastern Naturalist Vol. 24, No. 4
C. Kan, J. Lichter, and V. Douhovnikoff
2017
483
2017 NORTHEASTERN NATURALIST 24(4):483–498
Genetic Population Structure and Accuracy of
Morphological Assessment in Alosa aestivalis (Blueback
Herring) and A. pseudoharengus (Alewife)
Christopher Kan1,*, John Lichter2, and Vladimir Douhovnikoff
2
Abstract - Alosa aestivalis and A. pseudoharengus are herring congeners that are important
forage species for piscivorous fish and birds. We measured population structure metrics for
these species using microsatellite markers. The Southern Gulf of Maine study area allowed
the assessment of these species at an inter- and intra-watershed level. We found no detectable
population structure within or among watershed for either species which agrees with
other recent research. Our results support regional-scale (e.g., Gulf of Maine) plans for
management for A. aestivalis and A. pseudoharengus. We found that 5.4% of our samples
were hybrids. Our study adds to a growing body of evidence that hybridization and introgression
should be management concerns for these species, and precautions should be taken
to preserve species barriers. An error rate of morphological identification was calculated
by comparing morphological identifications against genetic classifications. We found an
overall identification error rate of 16%, which differed significantly from zero (P = 0.008).
Managers should also take note of the uncertainty in morphological identifications and adjust
stock models and policies accordingly.
Introduction
Alosa aestivalis (Mitchell) (Blueback Herring) and A. pseudoharengus (Wilson)
(Alewife) are in the sub-genus Pomolobus, a grouping that has been supported
strongly by recent phylogenetic studies (Bowen et al. 2008, Faria et al. 2006), and
pomoloboids is our preferred label for these 2 species, rather than the now paraphyletic
designation river herring that includes Alosa sapidissima (Wilson) (American
Shad). Pomoloboids are anadromous and co-occur for much of their range from
northern Florida to the Gulf of Saint Lawerence (NOAA 2013). They are an ecologically
important link between riparian and marine ecosystems because their
spawning material and carcasses support microbial and invertebrate populations
through increased nutrient availability (Durbin et al. 1979). The seaward migration
of young-of-the-year (YoY) fish reciprocally transports nutrients from freshwater
to marine environments (Limburg and Waldman 2009), enhancing the spawning
grounds for commercially important fish such as Gadus morhua (L.) (Atlantic Cod;
Ames 2004) and for endangered Salmo salar (L.) (Atlantic Salmon; Saunders et al.
2006). However, pomoloboids have declined across much of their range, leading to
their recent consideration for listing under the Endangered Species Act (National
1Ecology and Evolutionary Biology, University of California Santa Cruz, Santa Cruz, CA
95064. 2Biology Department, Bowdoin College, Brunswick, ME 04011. * Corresponding
author - chkan@ucsc.edu.
Manuscript Editor: Trevor Avery
Northeastern Naturalist
484
C. Kan, J. Lichter, and V. Douhovnikoff
2017 Vol. 24, No. 4
Oceanic and Atmospheric Administration 2013). Deforestation and dams have
driven local loss in habitat and, in conjunction with overfishing and pollution,
have devastated stocks (Lichter et al. 2006). The collapse of populations, combined
with the recognition of their ecological importance, has spurred restoration efforts.
Restoration efforts such as dam removals and water-quality improvement measures
have recently shown signs of success with a robust recovery of pomoloboids
in several Maine rivers (ASMFC 2012). Continued, enhanced, and broadened efforts
are required to increase recovery of these species. A fuller understanding of
the genetic population structure would help better target restoration efforts and
allow management to operate at the appropriate spatial scale (Weston et al. 2016),
but conflicting reports exist over the level of genetic population structure in North
American pomoloboids at the inter- and intra-watershed levels (Hasselman et al.
2010, Waters et al. 2000). Watershed-level analyses are necessary because fish
move within and between freshwater bodies, lakes, and rivers, using different
oceanic outlets. Assessing the accuracy of morphological identification methods
would also benefit managers who rely on morphological identifications for stock
assessments and modeling. Knowledge of the uncertainty of the identifications is a
key variable that affects model accuracy. Therefore, a better understanding of both
the population structure of and the accuracy of morphological identification of pomoloboids
would benefit restoration and management of the specie s.
This study seeks to help (1) address whether pomoloboids show population
structure at the intra- and inter-watershed levels and (2) ascertain the accuracy of
morphological species identification of pomoloboids. Both aims will be addressed
through a microsatellite analysis (Julian and Bartron 2007). Results from this study
will help to address knowledge gaps in the study area, and help to inform current
management practices such as deciding appropriate spatial scales for restoration
and including reliable indicators of morphological identificatio n.
Materials and Methods
Field sites
Our 8 field sites were in estuaries and rivers draining into the Gulf of Maine
(Fig. 1). Most sample sites (5 of 8) were within the Merrymeeting Bay watershed,
which drains 6 rivers comprising a quarter of Maine’s geographic area and part of
New Hampshire (24,755 km2). The bay served as the focal point of this study because
its complex hydrology and multiple branches consisting of numerous ponds,
lakes, and rivers allowed the investigation of intra-watershed population structure.
The Kennebec and Androscoggin Rivers are the major rivers in the watershed
(Fig. 1). The bay, which is estuarine and has a typical salinity of less than 5 but is tidally
influenced and can have elevated salinity during periods of low river flow, has tidal
marshes and sand flats which provide important habitat for birds (Kistner and Pettigrew
2001, Wong and Townsend 1999). Most samples were taken from freshwater
rivers or lakes feeding into the bay.
All sample locations were in freshwater except the Oyster River site and Winnigance
Lake, which can be brackish depending on flow and tidal conditions. All
Northeastern Naturalist Vol. 24, No. 4
C. Kan, J. Lichter, and V. Douhovnikoff
2017
485
river and lakes in the study except Winnigance Lake had dams and fish ladders
associated with their outlets. The sample sites have generally been affected by
pollution and overfishing in the past (Köster et al. 2007). Each site has one or
more man-made structures, such as dams on the Androscoggin River or power
plants on the Kennebec River, or settlements upstream that influence flow and
ecology (Stewart et al. 2006).
Sample collection and field identification
We collected 184 samples in total, of which 106 were adults and 78 were juveniles
(Table 1). Our collections included both adult A. aestivalis and juvenile
samples that we identified to the genus level Alosa, meaning they could be 1 of 3
species: A. aestivalis, A. pseudoharengus, or A. sapidissima. Adults and juveniles
were caught and identified using different means. We collected adult A. aestivalis
with fyke nets and coastal seining between 3 June 2012 and 25 April 2012, and
the specimens were identified by experts from Dr. Theodore Willis’s lab at the
Figure 1. Map of samples sites, where A. aestivalis and A. pseudoharengus, from Southern
New Hampshire to Midcoast Maine were collected. Samples were collected with fyke nets
or beach seines in rivers and estuaries between June and August 2012. Samples sites in the
Merrymeeting Bay watershed are shown with a black square. Sample sites in other watersheds
are indicted with a black dot. Watersheds included in this study are highlighted in bold
non-italicized text. Italicized text indicate geographic features other than sample sites and
are for geographic reference only.
Northeastern Naturalist
486
C. Kan, J. Lichter, and V. Douhovnikoff
2017 Vol. 24, No. 4
Table 1. Summary of collections made, showing sample sites, what types of samples were caught, the date(s) that they were visited, and what species, A.
pseudoharengus (Ale), A. aestivalis (BB), or hybrid, were caught based on a classification by a gen etic simulation protocol.
Sample # of # of # of
Site Dates caught type BB Ale hybrids Total # % BB % Ale % hybrid
Dresden Mills 1 June 2012 Adult 29 1 2 32 90.60% 3.13% 6.25%
Benton Falls 13 June 2012 Adult 23 2 2 27 85.20% 7.41% 7.41%
Nequasset Dam 9 August 2012 Juvenile 0 18 0 18 0.00% 100.00% 0.00%
Sheepscot River 8 July 2012, 12 July 2012 Juvenile 2 21 0 23 8.70% 91.30% 0.00%
Presumpscot River 1 August 2012 Juvenile 0 14 0 14 0.00% 100.00% 0.00%
Damriscotta River 13 August 2012 Juvenile 0 23 0 23 0.00% 100.00% 0.00%
Winnigance Lake 12 June 2012 Adult 21 0 0 21 100.00% 0.00% 0.00%
Oyster River 2 July 2012 Adult 16 4 6 26 61.50% 15.40% 23.10%
Total 91 83 10 184 49.50% 45.10% 5.43%
Northeastern Naturalist Vol. 24, No. 4
C. Kan, J. Lichter, and V. Douhovnikoff
2017
487
University of Southern Maine. The identification used the color and luminosity of
the peritoneum, light and shiny in A. pseudoharengus and dark and dusky with spots
in A. aestivalis. This is the standard method for distinguishing between A. aestivalis
and A. pseudoharengus . We were not able, due to logistical constraints, to target
rivers in which adult A. pseudoharengus predominated; therefore, adult samples
sizes of this species were low. We collected juvenile samples of this species with
a 50-foot beach seine between 1 June 2012 and 13 August 2012. Sampling information
including sampling date are provided in Table 1. We identified juvenile
samples to the genus Alosa using body shape, head shape, and mouth morphology
(Leim and Scott 1966).
We used only adult samples to test the accuracy of the peritoneum identifications.
It is very difficult to reliably identify juveniles through morphology to species
level (MacLellan et al. 1981); thus, they were not used to test morphological species
identifications. All samples were used to investigate population structure in
pomoloboids.
DNA extraction protocol, PCR amplification, and genotyping
We utilized 7 microsatellite markers that were originally developed in A. sapidissima
for the genus Alosa to characterize the samples in the study (Table 2; Julian
and Bartron 2007). Phenol-chloroform extractions were performed based on a protocol
by Taggart et al. (1992). Briefly, we placed ~150 mg of skeletal muscle tissue
in a 2.5-ml tube, and added 600 μl of CTAB Mixture, consisting of 0.2 M EDTA
(ethylenediamine-tetraacetic acid), 2% Cetyl trimethylammonium bromide, and 25
μl of protinase K solution 20 mg/ml, and incubated the sample for 8 hours at 37 °C.
Following incubation, we added 10 μl of 2 mg/ml RNAase A, DNAse and proteasefree
solution to the tube and mixed it. The sample was then incubated for 1 hour at
37 °C. After this, we aded 600 μl of Phenol-Chloroform-Isoamyl Alcohol (PCI) in
a 24:24:1 ratio to each tube. Samples were mixed and centrifuged to separate layers.
The bottom PCI layer was then removed, 600 μl of Chloroform-Isoamyl in a
24:1 ratio (CI) was added, and the tube was mixed and centrifuged again. The top
aqueous layer containing DNA was removed to a new tube using a wide bore pipet.
We repeated this PCI–CI process once more for a cleaner sample because we found
that doing so resulted in better DNA amplification for our samples.
We then added isopropyl alcohol (600 μl) and 60 μl of 10.5 M Ammonium
Acetate to the isolated DNA and decanted the solution from the precipitated DNA
after centrifuging. Thereafter, 600 μl of -20°C 99% ethanol was added to the tube,
centrifuged, and then decanted. We dried the tubes for 10 mins at room temperature
and then thoroughly washed them with 50 μl of Tris-EDTA (10mM Tris, 1mM
EDTA; ph 8.0) and allowed them to sit for 24 hours to achieve full dissolution.
We performed PCR amplification for all primers with either a standard or touchdown
protocol (Table 2). The standard protocol consisted of a hot start with (1) an
initial denaturing at 94 °C for 2 min, (2) denaturing at 94 °C for 45 s, (3) annealing
at 56 °C for 45 s, (4) extension at 72 °C for 2 min, and (5) final extension at 72 °C
for 5 min. We repeated steps 2–4 for 35 cycles. The touchdown protocol involved
Northeastern Naturalist
488
C. Kan, J. Lichter, and V. Douhovnikoff
2017 Vol. 24, No. 4
Table 2. Information about the primers used and resulting amplicons for A. pseudoharengus (Ale) and A. aestivalis (BB). All primers used the FAM fluorophore
except AsaD042, which used MAX (VIC substitute). Primers were original ly from Julian and Bartron (2007).
PCR No. of No. of Ale BB
Genbank Repeat amplification alleles alleles size size
Primer accession no. motif conditions Primer sequences (5’–3’) (Ale) (BB) (max–min bp) (max–min bp)
AsaC010 EF014991 (GTAT)16 Touchdown F: AATAATGTTGTGCTGGATTGTG 7 7 264–308 264–308
R: TTTATTGTTATTGTGATGGAGGG
AsaC249 EF014994 (CATA)8 Standard F: TTATTACAACGGTGAATTGAGTG 7 2 193–221 193–221
(TTCT)13 R: TAAGTGCATGTTGTGTGTGATG
AsaD021 EF014996 (CTAT)15 Standard F: CTCTCTTCCCCATCACTCTTC 5 6 260–276 216–284
R: CAAAGCCCTCGTTTAGTTATTC
AsaD029 EF014997 (CTAT)20 Touchdown F: ATTATGCACAGGAATCTGGAAG 9 16 216–284 216–284
R: TGTGCTTACAAAAGTGACATGG
AsaD030 EF014998 (CTAT)23 Touchdown F: CCACAGCATCATCTCTTTACTG 6 9 84–122 84–122
R: ACCTTGAATTTCTCCTTGGG
AsaD042* EF015000 (CTAT)12 Touchdown F: ACTGGTCAATTGTAAGACACCC 7 10 144–202 144–202
R: CAAGATGACCAAGGGTTAAGAC
AsaD055 EF015001 (CTAT)10 Touchdown F: CTCTTTCACAGGGATCAAAGTC 6 12 247–312 247–213
R: CAAGCATGTTTAAATAGGAGGC
Northeastern Naturalist Vol. 24, No. 4
C. Kan, J. Lichter, and V. Douhovnikoff
2017
489
a starting annealing temperature of 64 °C, which was reduced by 0.5 °C each additional
cycle until, at cycle 10, a final annealing temperature of 54 °C was reached.
This final temperature was used for annealing in cycles 11–35. The other steps and
parameters in the touchdown protocol were the same as the stand ard protocol.
Microsatellites were genotyped on an ABI 3730xL DNA Analyzer at the Harvard
Biopolymers Facility (Cambridge, MA) and were scored using Applied Bio
Systems PeakScanner software 2.0 (Carlsbad, CA). The allele calls were double
checked by the initial researcher, and a random sample was triple checked for accuracy
by other researchers. No significant deviation between researchers was found
in allele calling. The data was checked in Microchecker v. 2.23 (Van Oosterhout et
al. 2004) for genotyping and amplification errors such as allelic dropouts, excess
small-allele amplification, and stutter products.
Genetic sample classification using a simulation-based pr otocol
We analyzed the microsatellite data to differentiate samples by species according
to the protocol by Hasselman et al. (2014). Briefly, the protocol can be broken
into 2 basic steps: (1) perform computer hybridization simulations to determine
which q-values (a numerical value indicating a sample’s likely genetic makeup and
ancestry) correspond to purebred or hybrid individuals, and (2) run programs on
collected samples to obtain q-values and use the benchmarks from simulations to
identify species. The meaning of a q-value can change based on the species studied
and the markers used (Evanno et al. 2005, Hasselman et al. 2014). Hence simulations
of hybridization and backcrosses of assumed purebreds are a best practice for
determining the likely ancestry of an individual for any given q-value.
We ran the 154 most pure individuals, defined as having a q score ≥0.9 by
STRUCTURE v2.3.4 (Pritchard et al. 2000), through simulated hybridization and
backcross events in Hybrid Lab v1.0 (Nielsenet al. 2006). Five simulated data sets
resulted from this exercise. The first step we took was using Hybrid Lab to create
200 genetic profiles for each species. These 400 purebred parental individuals were
then used to generate 100 F1 hybrids, which in turn were used to generate 100 F2
hybrids and 100 F1 hybrids backcrossed with purebred parents, hereafter referred
to as F1 backcrosses. We analyzed each of these simulated groups in STRUCTURE
and New Hybrids v1.1 (Anderson and Thompson 2002) to determine which ancestry
scores, q-values in STRUCTURE and PofZ in New Hybrids, corresponded to
different levels of introgression. This approach allowed us to have benchmarks for
each software for what constituted a purebred or a hybrid individual. It also allowed
us to assess what each program was capable of discerning. For example, whether
first generation hybrids could be distinguished from F1 backcros ses.
Each simulated dataset of hybrids was run in STRUCTURE with the admixture
model for 100k burn-in steps, 500k iterations and, 5 repetitions for each K
(the number of genetic clusters, 1 thru 6). Results were combined across repetitions
using Structure Harvester (Earl and vonHoldt 2012) and CLUMPP v1.1.2
(Jakobsson and Rosenberg 2007) and visualized with distruct v1.1 (Rosenberg
2004) or Structure Plot (Ramasamy et al. 2014). We identified the ideal K per the
Northeastern Naturalist
490
C. Kan, J. Lichter, and V. Douhovnikoff
2017 Vol. 24, No. 4
Evanno method (Evanno et al. 2005). The Evanno method expands on the original
Prichard paper, which suggested using Ln(K), also referred to as L(K), to find the
true value of K, and proposes using instead delta K for times when the real value of
K is greater than 1. The delta K metric was found to be better than Ln(K) at detecting
the true K through simulations for Ks > 1 (Evanno et al. 2005, Pritchard et al.
2000). Using this information from these methods, we assessed for each q-value the
likelihood and confidence of species classification. For a q-score in an analysis with
only 2 clusters, 0 is one purebred species and 1 is the other. Hence all individuals
are more like one cluster or the other depending on the score. For example, in our
study, if 1 is strongly A. pseudoharengus and 0 is A. aestivalis, a q-value of 0.8
in STRUCTURE might mean a sample has a 90% change of being an A. pseudoharengus,
9% of being a A. aestivalis and 1% of being a hybrid. We found during
our testing that STRUCTURE was not able to distinguish between different types
of hybrids, but was easily able to distinguish between the 2 purebred species and
between purebreds and hybrids.
New Hybrids was run for 10k burn-in steps. 200k iterations, and replicated 4 times
per simulated dataset. The program outputs a PofZ value for each sample that gives
a percent chance a given sample is a member of 6 possible classes: purebred species
1 or 2, F1 hybrid, F2 hybrid, or a F1 backcross with species 1 or species 2. We found
in our simulations that New Hybrids was not capable of distinguishing between the
hybrid categories within our data, likely due to the number of markers. We therefore
summed all the hybrid categories into a single hybrid category that resulted in a sufficient
signal to distinguish between sample types. Hence, we were only asking New
Hybrids to distinguish between 3 types of samples: pure A. aestivalis, pure A. pseudoharengus
or hybrids of any of the 4 aforementioned types.
This group structure allowed us to convert these PofZ numbers to a q-value
equivalent since New Hybrids was easily able to distinguish between pure A. aestivalis
and pure A. pseudoharengus in our simulations, i.e., if one PofZ probability
was high for one of the parent species the PofZ probability for the other would
be near zero. New Hybrids easily distinguished between the simulated purebred
samples but occasionally had difficulty distinguishing purebreds and hybrids. Using
these principals, we converted a PofZ to a q-value like number as follows.
For example, where a PofZ indicates the chance of A. aestivalis as 90%, A. pseudoharengus
as less than 1%, and the hybrid as 10%, New Hybrids treats the sample as A.
aestivalis with the small chance of it being a hybrid. If we think back to the q-value
scale in the case of K = 2, where A. aestivalis as 0 and A. pseudoharengus as 1, we
can convert the PofZ to a q-value of 0.10. One can think of the hybrid percentage
as the deviation from the purebred percentage; hence, it can be added in the case
of A. aestivalis to 0 or subtracted for A. pseudoharengus from 1. Thus, looking at
another example, a PofZ of A. aestivalis as less than 1%, A. pseudoharengus as 70%, and
the hybrid as 30% would be 1 - 0.3 = 0.7 = q-value.
We used a simple species identification consensus score for each of our 184
samples based on the information obtained from our hybridization simulations
with STRUCTURE and New Hybrids. For a sample of one of our simulations, see
Northeastern Naturalist Vol. 24, No. 4
C. Kan, J. Lichter, and V. Douhovnikoff
2017
491
Supplemental Table 1 (available online at https://www.eaglehill.us/NENAonline/
suppl-files/n24-4-N1442-Kan-s1, and, for BioOne subscribers, at https://dx.doi.
org/10.1656/N1442.s1). The synthetic consensus score was from 0 to 2, where
scores of 0, 1, or 2 were possible. Zero meant a disagreement of both programs
binning the sample into the 2 parent species, the greatest possible level of disagreement;
this score never occurred in our samples. One meant one program identified
a sample as a hybrid and the other identified it as a purebred. Two meant that both
programs identified a sample as a hybrid or as one of the parent species. The samples
that scored 1 were manually reviewed by researchers and final classification
judgments were made using the statistical information obtained from the Hybrid
Lab simulations.
Analyzing each species for population structure and other metrics
We analyzed samples identified as A. aestivalis and A. pseudoharengus for population
structure in separate species-specific STRUCTURE and New Hybrids runs
using the same burn-in steps, Ks, iterations, and repetitions parameters as above. The
Evanno method (Evanno et al. 2005) was applied again to find the most likely K and
number of genetic clusters in the sample to infer genetic structure at the inter- and
intra-watershed scale. We also used identifications from the STRUCTURE and New
Hybrids analyses to determine the accuracy of morphological field identifications
of adult samples. Deviation from the null hypothesis of no identification error was
evaluated using Fisher’s Exact Test in JMP Pro 11 (Cary, NC). We analyzsed species
data sets in Genepop v4.2 (Rousset 2008) to calculate tests for Hardy Weinburg Equlibrium
and to calculate expected and observed heterozygosity, allelic richness, and
FIS. G-statistic based P-values were calculated in FSTAT v. 2.9.3.2 (Goudet 1995).
Statistical tests underwent sequential Bonferroni correction (Holm 1979) to account
for the effects of multiple statistical tests. The Holm method is more powerful than
Bonferroni correction (Allendorf and Phelps 1981). We categorized private alleles,
alleles exclusive to one species, using GenALEx v6.5 (Peakall and Smouse 2012).
Results
Of 184 samples, none scored 0 on our species identification score, 6 scored 1,
and 178 scored 2. Thus, for 97% of our samples, STRUCTURE and New Hybrids
agreed on individual identification as hybrid, A. aestivalis, or A. pseudoharengus
(Table 1). This result suggests the analysis could easily classify most samples.
The analysis did not indicate the presence of a third species in our sample (Figure
2Ai–Aiii). This result means that no A. sapidissima, the third alosid present in the
system, were found during sampling. The markers used had greater or different bp
size ranges from those originally reported for A. pseudoharengus and A. aestivalis
in Julian and Bartron (2007). We believe that we found different bp size ranges
because we used larger sample sizes of 91 and 83 per species rather than 2–3 per
species as in Julian and Bartron (2007). Some of the size variation is also likely due
to regional variation in genetic composition since our samples were from the Gulf
of Maine and those in Julian and Bartron (2007) were from Long Island Sound. The
Northeastern Naturalist
492
C. Kan, J. Lichter, and V. Douhovnikoff
2017 Vol. 24, No. 4
Figure 2. [Caption on following page.]
Northeastern Naturalist Vol. 24, No. 4
C. Kan, J. Lichter, and V. Douhovnikoff
2017
493
size ranges for both species in our sampling are identical (Table 2), which is not
surprising due to hybridization between the species facilitatin g allele exchange.
A t-test found a significant departure (P = 0.008) from the null hypothesis that
the morphological identifications had no significant difference from the genetic
classifications. Of the 106 morphologically identified adult A. aestivalis that we sequenced,
7 were later genetically classified as A. pseudoharengus and 10 as hybrids.
We calculated 2 error rates, one for errors where adult A. pseudoharengus were mistaken
as A. aestivalis and a second for those that resulted from hybrid adults, that
were likely morphologically cryptic. These rates are 6.6% and 9.4% respectively,
for an overall morphological identification error rate of 16.0%.
We found no evidence for genetic population structure at inter- or intra-watershed
levels for either species. Admixture coefficient plots generated by STRUCTURE
(Fig. 2B, C) show 2 potential intra-species clusters within A. aestivalis, and
A. pseudoharengus (Fig. 2Bi–ii , Ci–ii), but we determined that both species likely
only had a single cluster because of the low and non-significant FSTs between the
potential clusters. The FSTs were 0.01 (P = 0.67) and 0.002 (P = 0.55) for A. pseudoharengus
and A. aestivalis, respectively. Since the Evanno method cannot be
used to detect single clusters of K = 1 (Evanno et al. 2005), log probability plots,
Ln(K), were used instead (Fig. 2Biii, Ciii). The plots support the hypothesis that
the most likely number of clusters is 1, suggesting there is no detectable genetic
structure within either species in our sample. Most markers showed significant
divergences from the Hardy–Weinberg equilibrium (HWE) in both A. aestivalis
and A. pseudoharengus (Table 3). The percentage of hybrids across sites was low,
comprising 5.43% of all samples. The Oyster River, which has the slowest and narrowest
river area, had the highest percentage of hybrids (Table 1). Limited sample
sizes (Table 1) per site likely prevented us from finding any relationship between
sample site and the number of hybrids ( P = 0.310).
Discussion
Our results support no genetic population structure at the intra- or inter-watershed
level within our Gulf of Maine study region. Our study adds to a growing
line of evidence from studies covering the eastern seaboard that genetic structure
for pomoloboids is best discernable at region-wide scales like the Gulf of Maine
rather than at watershed scales (McBride et al. 2014, Palkovacs et al. 2014). Results
suggest 7 markers were sufficient for this analysis due to the strong performance
Figure 2. STRUCTURE, Evanno method, and log probability plots to identify the correct
number of genetic clusters. Panel A shows the STRUCTURE and Evanno plots for a combined
sample of A. pseudoharengus and A. aestivalis at K = 2 (Ai.) and K = 3 (Aii.) (FST
= 0.227). The subsequent 2 panels for (B) A. pseudoharengus and (C) A. aestivalis show
STRUCTURE plots at K = 2 and K = 3 with 2 clusters evident. However, low FST values
(0.017 and 0.002, respectively) indicate that there is only 1 true cluster in both species. The
Evanno method cannot detect only 1 cluster, so a graph of the recommended alternative
method using the log probability of the data is shown in the right column as Biii and Ciii.
All STRUCTURE plots are K = 2 on top and K = 3.
Northeastern Naturalist
494
C. Kan, J. Lichter, and V. Douhovnikoff
2017 Vol. 24, No. 4
that New Hybrids and STRUCTURE exhibited in simulations when distinguishing
between purebreds and hybrids. We caution though that STRUCTURE and New
Hybrids results should be analyzed in the context of significant deviations from
HWE in most markers, which can affect the results of both models (Table 3). The
deviations could be a result of introgression. The evidence from our study and
others suggests that the Gulf of Maine is likely a genetic unit that has significant
internal gene flow and that regional-scale management for fisheries and conservation
may be appropriate for pomoloboids.
Our results along with the recent genetic studies by Hasselman et al. (2014) and
Mcbride et al. (2014) suggest that pomoloboids have a clear potential for hybridization.
Our study found 5.4% of our samples were hybrids; however, it was not
feasible to determine the precise type of hybrid due to insufficient statistical power.
Our finding that the size ranges of the microsatellite alleles for these 2 species
exactly overlap suggests that introgression has allowed the reciprocal movement
of alleles overtime. Our findings are supported by Hasselman et al. (2014), which
documented the formation of a hybrid swarm in a lake after a dam was built, and
Mcbride et al. (2014), which documented F1 hybrids and advanced backcrosses.
These studies suggest there are many opportunities for hybridization since the
species overlap over much of their range and during much of their spawning time
in freshwater. When the 2 species were found to co-occur, both Hasselman et al.
(2014) and Mcbride et al. (2014) found increased levels of hybridization These
findings are consistent with our observations of hybridization. Even though our
sampling approach at each site did not always capture both species, we believe
that this is further evidence that they can and do interbreed and that the chance of
hybridization increases with the level of interspecies contact.
Genetic and ecological evidence indicate that hybridization and the subsequent
introgression of alleles is occurring between the 2 species. Therefore, managers
should take caution to prevent the formation of hybrid swarms. It is probable that
hybrids have always occurred between these species, but elevated numbers of
hybrids backcrossing into parent species can erode the barriers to gene flow over
time leading to the merging of gene pools. Activities such as habitat modification
Table 3. Population structure measures for A. pseudoharengus (Ale) and A. aestivalis (BB). Ho and
He are observed and expected heterozygosity, respectively. P-values are g-statistic based for Hardy-
Weinburg equilibrium (HWE). * indicates significant deviations from HWE of P < 0.05.
Ale BB Ale BB
Ho He Ho He Ale BB P-Value P-value Allelic Allelic
Primer (Ale) (Ale) (BB) (BB) Fis Fis (HWE) (HWE) richness richness
AsaC010 0.349 0.241 0.582 0.409 -0.461 -0.429 less than 0.001* less than 0.001* 1.900 4.114
AsaC249 0.337 0.272 0.241 0.139 -0.243 -0.756 0.431 less than 0.001* 2.044 1.934
AsaD021 0.542 0.387 0.373 0.265 -0.407 -0.414 less than 0.001* less than 0.001* 2.371 4.689
AsaD029 0.108 0.097 0.659 0.602 -0.116 -0.095 0.870 0.095 3.213 8.012
AsaD030 0.590 0.439 0.659 0.532 -0.347 -0.324 less than 0.001* less than 0.001* 2.613 4.955
AsaD042 0.602 0.476 0.373 0.311 -0.267 -0.201 0.030* 0.012* 2.841 5.137
AsaD055 0.156 0.111 0.615 0.527 -0.424 -0.168 less than 0.001* 0.646 2.749 6.258
Northeastern Naturalist Vol. 24, No. 4
C. Kan, J. Lichter, and V. Douhovnikoff
2017
495
by dam building or bank engineering should be undertaken with caution, especially
when they may reduce spawning space or cause crowding. Similarly, stocking
could cause crowding by reducing the spawning space available per individual,
or might introduce a new species to a watershed. Both habitat modification and
stocking may lead the species to hybridize at a higher rate than historically found
in the system since it will restrict their ability to segregate activities by species.
For instance, Hasselman et al. (2014) documented that the construction of a dam
caused 2 pomoloboids populations to collapse. The habitat modification caused by
the dam perturbed the populations and they merged into a hybrid swarm. Similarly,
stocking may increase straying compared to native individuals such as in salmonids
(Quinn 1993, Stabell 1984). Therefore, managers must carefully consider events or
actions, direct or indirect, that could affect the ability for the species to segregate
when mating.
Hybridization could complicate stock monitoring and reduce the accuracy of
the morphological identifications managers typically rely on for pomoloboids.
We found that morphological identifications by experts using the peritoneum,
the most common morphological identifier to distinguish between the 2 species
(Leim and Scott 1966, MacLellan et al. 1981), had an error rate of 16%. This
rate, considered adequate by the Food and Agriculture Organization of the UN
(Fischer 2013:27), could still complicate or influence model predictions. Pomoloboids
are notoriously difficult to identify by any morphological means due
to their physical similarity. Yet, morphological methods, particularly the peritoneum,
are still used as the primary identification method for stock assessments.
Managers should note the level of error associated with these methods and incorporate
it into the models used to determine stock policies such as management
unit size and bycatch estimates.
Similar with other studies, genetic makeup may have been influenced by the history
of extensive stocking of A. pseudoharengus in Maine (Hasselman et al. 2013)
and recent recoveries (ASMFC 2012). It is possible that there were greater levels of
genetic structure prior to human intervention and that stocking has reduced genetic
population structure by homogenizing previously distinct populations (McBride et
al. 2015). The effects of stocking are thought to be varied in pomoloboids depending
on the level of selection in the populations (Bentzen et al . 2011).
Future work is still needed to clarify the parameters of alosine migration in
recovering areas. Messieh (1977), using multivariate analysis of morphological
characteristics, and Jessop (1994), using genetic markers, measured fidelity to
natal rivers varying from 63% to 97%. It would be informative to know if fidelity
to natal rivers changes with habitat degradation or recovery. For example,
lower fidelity during recovery would facilitate re-colonization of newly available
habitat. The measurement of this parameter would assist in models of population
restoration or colonization that are critical to the conservation of A. aestivalis and
A. pseudoharengus. Additional work regarding the frequency and mechanism of
hybridization within pomoloboids would greatly inform restoration and efforts to
preserve the genetic integrity of this taxon .
Northeastern Naturalist
496
C. Kan, J. Lichter, and V. Douhovnikoff
2017 Vol. 24, No. 4
Acknowledgments
The authors thank their generous funders of this project: the Rusack Coastal Studies
Fellowship, the Grua/o'Connell Research Award, the Roberts Fund, the Howard Hughes
Medical Institute Fellowship, and Bowdoin Biology. We are also grateful to Theodore Willis
for his assistance with analysis and samples. Finally, thank you to Daniel Hasselman,
Nate Grey, Gail Wippelhauser ,and Mark Lazzari for their invaluable help with data collection
and analysis.
Literature Cited
Allendorf, F.W., and S.R. Phelps. 1981. Use of allelic frequencies to describe population
structure. Canadian Journal of Fisheries and Aquatic Sciences 38:1507–1514.
Ames, E.P. 2004. Atlantic cod stock structure in the Gulf of Maine. Fisheries 29 :10–28.
Anderson, E.C., and E.A. Thompson. 2002. A model-based method for identifying species
hybrids using multilocus genetic data. Genetics 160:1217–1229.
Atlantic States Marine Fisheries Commission (ASMFC). 2012. River Herring Benchmark
Stock Assessment. Volumes I and II. Arlington, VA.
Bentzen, P., I. Paterson, M. McBride, and T.V. Willis. 2011. Genetic analysis of anadromous
Alewife populations in Maine. Maine Rivers, Yarmouth, ME.
Bowen, B.R., B.R. Kreiser, P.F. Mickle, J.F. Schaefer, and S.B. Adams. 2008. Phylogenetic
relationships among North American Alosa species (Clupeidae). Journal of Fish Biology
72:1188–1201.
Durbin, A.G., S.W. Nixon, and C.A. Oviatt. 1979. Effects of the spawning migration of the
Alewife, Alosa Pseudoharengus, on Freshwater Ecosystems. Ecology 60:8–17.
Earl, D.A., and B.M. vonHoldt. 2012. STRUCTURE HARVESTER: A website and program
for visualizing STRUCTURE output and implementing the Evanno method. Conservation
Genetics Resources 4:359–361.
Evanno, G., S. Regnaut, and J. Goudet. 2005. Detecting the number of clusters of individuals
using the software structure: A simulation study. Molecular Ecology 14:2611–2620.
Faria, R., S. Weiss, and P. Alexandrino. 2006. A molecular phylogenetic perspective on the
evolutionary history of Alosa spp. (Clupeidae). Molecular Phylogenetics and Evolution
40:298–304.
Fischer, J. (Ed.). 2013. Fish identification tools for biodiversity and fisheries assessments:
Review and guidance for decision-makers. Food and Agriculture Organization of the
United Nations, Rome, Italy.
Goudet, J. 1995. FSTAT (Version 1.2): A computer program to calculate F-statistics. Journal
of Heredity 86:485–486.
Hasselman, D.J., R.G. Bradford, and P. Bentzen. 2010. Taking stock: Defining populations
of American Shad (Alosa sapidissima) in Canada using neutral genetic markers. Canadian
Journal of Fisheries and Aquatic Sciences 67:1021–1039.
Hasselman, D.J., D. Ricard, and P. Bentzen. 2013. Genetic diversity and differentiation in
a wide-ranging anadromous fish, American Shad (Alosa sapidissima), is correlated with
latitude. Molecular Ecology 22:1558–1573.
Hasselman, D.J., E.E. Argo, M.C. McBride, P. Bentzen, T.F. Schultz, A.A. Perez-Umphrey,
and E.P. Palkovacs. 2014. Human disturbance causes the formation of a hybrid swarm
between two naturally sympatric fish species. Molecular Ecology 23:1137–1152.
Holm, S. 1979. A simple sequentially rejective multiple test procedure. Scandinavian Journal
of Statistics 6:65–70.
Northeastern Naturalist Vol. 24, No. 4
C. Kan, J. Lichter, and V. Douhovnikoff
2017
497
Jakobsson, M., and N.A. Rosenberg. 2007. CLUMPP: a cluster matching and permutation
program for dealing with label switching and multimodality in analysis of population
structure. Bioinformatics 23:1801–1806.
Jessop, B.M. 1994. Homing of Alewives (Alosa pseudoharengus) and Blueback Herring (A.
aestivalis) to and within the Saint John River, New Brunswick, as indicated by tagging
data. Department of Fisheries and Oceans Canada, Biological Sciences Branch, Freshwater
and Anadromous Division, Halifax, NS, Canada.
Julian, S.E., and M.L. Bartron. 2007. Microsatellite DNA markers for American Shad (Alosa
sapidissima) and cross-species amplification within the family Clupeidae. Molecular
Ecology Notes 7:805–807.
Kistner, D.A., and N.R. Pettigrew. 2001. A variable turbidity maximum in the Kennebec
estuary, Maine. Estuaries 24:680–687.
Köster, D., J. Lichter, P.D. Lea, and A. Nurse. 2007. Historical eutrophication in a river–estuary
complex in mid-coast maine. Ecological Applications 17:765–778.
Leim, A.H., and W.B. Scott. 1966. Fishes of the Atlantic coast of Canada. Bulletin of the
Fisheries Research Board of Canada 155. Ottawa, ON, Canada.
Lichter, J., H. Caron, T.S. Pasakarnis, S.L. Rodgers, T.S. Squiers, and C.S. Todd. 2006. The
ecological collapse and partial recovery of a freshwater tidal ecosystem. Northeastern
Naturalist 13:153–178.
Limburg, K.E., and J.R. Waldman. 2009. Dramatic declines in North Atlantic diadromous
fishes. BioScience 59:955–965.
MacLellan, P., G.E. (Buck) Newsome, and P.A. Dill. 1981. Discrimination by External Features
between Alewife (Alosa pseudoharengus) and Blueback Herring (A. aestivalis).
Canadian Journal of Fisheries and Aquatic Sciences 38:544–546.
McBride, M.C., T.V. Willis, R.G. Bradford, and P. Bentzen. 2014. Genetic diversity and
structure of two hybridizing anadromous fishes (Alosa pseudoharengus, Alosa aestivalis)
across the northern portion of their ranges. Conservation Gen etics 15:1281–1298.
McBride, M.C., D.J. Hasselman, T.V. Willis, E.P. Palkovacs, and P. Bentzen. 2015. Influence
of stocking history on the population genetic structure of anadromous Alewife
(Alosa pseudoharengus) in Maine rivers. Conservation Genetics 1–15.
Messieh, S.N. 1977. Population structure and biology of Alewives (Alosa pseudoharengus)
and Blueback Herring (A. aestivalis) in the Saint John River, New Brunswick. Environmental
Biology of Fishes 2:195–210.
National Oceanic and Atmospheric Administration (NOAA). 2013. Endangered and threatened
wildlife and plants; Endangered Species Act listing determination for Alewife and
Blueback Herring. Federal Register 78:48944–48994.
Nielsen, E.E., L.A. Bach, and P. Kotlicki. 2006. hybridlab (version 1.0): A program for
generating simulated hybrids from population samples. Molecular Ecology Notes
6:971–973.
Palkovacs, E.P., D.J. Hasselman, E.E. Argo, S.R. Gephard, K.E. Limburg, D.M. Post, T.F.
Schultz, and T.V. Willis. 2014. Combining genetic and demographic information to prioritize
conservation efforts for anadromous Alewife and Blueback Herring. Evolutionary
Applications 7:212–226.
Peakall, R., and P.E. Smouse. 2012. GenAlEx 6.5: Genetic analysis in Excel. Population
genetic software for teaching and research—an update. Bioinform atics 28:2537–2539.
Pritchard, J.K., M. Stephens, and P. Donnelly. 2000. Inference of population structure using
multilocus genotype data. Genetics 155:945–959.
Northeastern Naturalist
498
C. Kan, J. Lichter, and V. Douhovnikoff
2017 Vol. 24, No. 4
Quinn, T.P. 1993. Biological interactions of natural and enhanced stocks of salmon: A review
of homing and straying of wild and hatchery-produced salmon. Fisheries Research
18:29–44.
Ramasamy, R.K., S. Ramasamy, B.B. Bindroo, and V.G. Naik. 2014. STRUCTURE PLOT:
A program for drawing elegant STRUCTURE bar plots in user friendly interface.
SpringerPlus 3. Accessed 15 April 2015.
Rosenberg, N.A. 2004. distruct: A program for the graphical display of population structure.
Molecular Ecology Notes 4:137–138.
Rousset, F. 2008. genepop’007: A complete re-implementation of the genepop software for
Windows and Linux. Molecular Ecology Resources 8:103–106.
Saunders, R., M.A. Hachey, and C.W. Fay. 2006. Maine’s diadromous fish community.
Fisheries 31:537–547.
Stabell, O.B. 1984. Homing and olfaction in salmonids: A critical review with special reference
to the Atlantic Salmon. Biological Reviews 59:333–388.
Stewart, G., J.M. Caldwell, A.R. Cloutier, and L.E. Flight. 2006. Water resources data
maine water year 2005. US Geological Survey, Augusta, ME. Available online at https://
pubs.usgs.gov/wdr/2005/wdr-me-05-1/. Accessed 28 March 2015.
Taggart, J.B., R.A. Hynes, P.A. Prodöuhl, and A. Ferguson. 1992. A simplified protocol for
routine total DNA isolation from salmonid fishes. Journal of Fish Biology 40:963–965.
Van Oosterhout, C., W.F. Hutchinson, D.P.M. Wills, and P. Shipley. 2004. micro-checker:
software for identifying and correcting genotyping errors in microsatellite data. Molecular
Ecology Notes 4:535–538.
Waters, J.M., J.M. Epifanio, T. Gunter, and B.L. Brown. 2000. Homing behaviour facilitates
subtle genetic differentiation among river populations of Alosa sapidissima: Microsatellites
and mtDNA. Journal of Fish Biology 56:622–636.
Weston, K.A., S.S. Taylor, and B.C. Robertson. 2016. Identifying populations for management:
Fine-scale population structure in the New Zealand Alpine Rock Wren (Xenicus
gilviventris). Conservation Genetics 17:691–701.
Wong, M.W., and D.W. Townsend. 1999. Phytoplankton and hydrography of the Kennebec
estuary, Maine, USA. Marine Ecology Progress Series 178:133–144.