Application of Mobile Dual-frequency Identification Sonar
(DIDSON) to Fish in Estuarine Habitats
Kenneth W. Able, Thomas M. Grothues, Jenna L. Rackovan, and Frances E. Buderman
Northeastern Naturalist, Volume 21, Issue 2 (2014): 192–209
Full-text pdf (Accessible only to subscribers. To subscribe click here.)
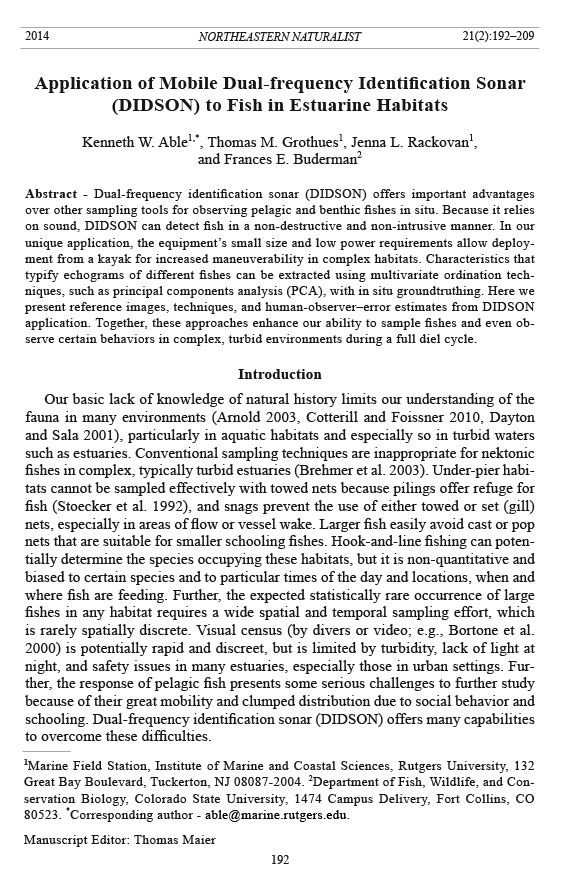
Access Journal Content
Open access browsing of table of contents and abstract pages. Full text pdfs available for download for subscribers.
Current Issue: Vol. 30 (3)

Check out NENA's latest Monograph:
Monograph 22
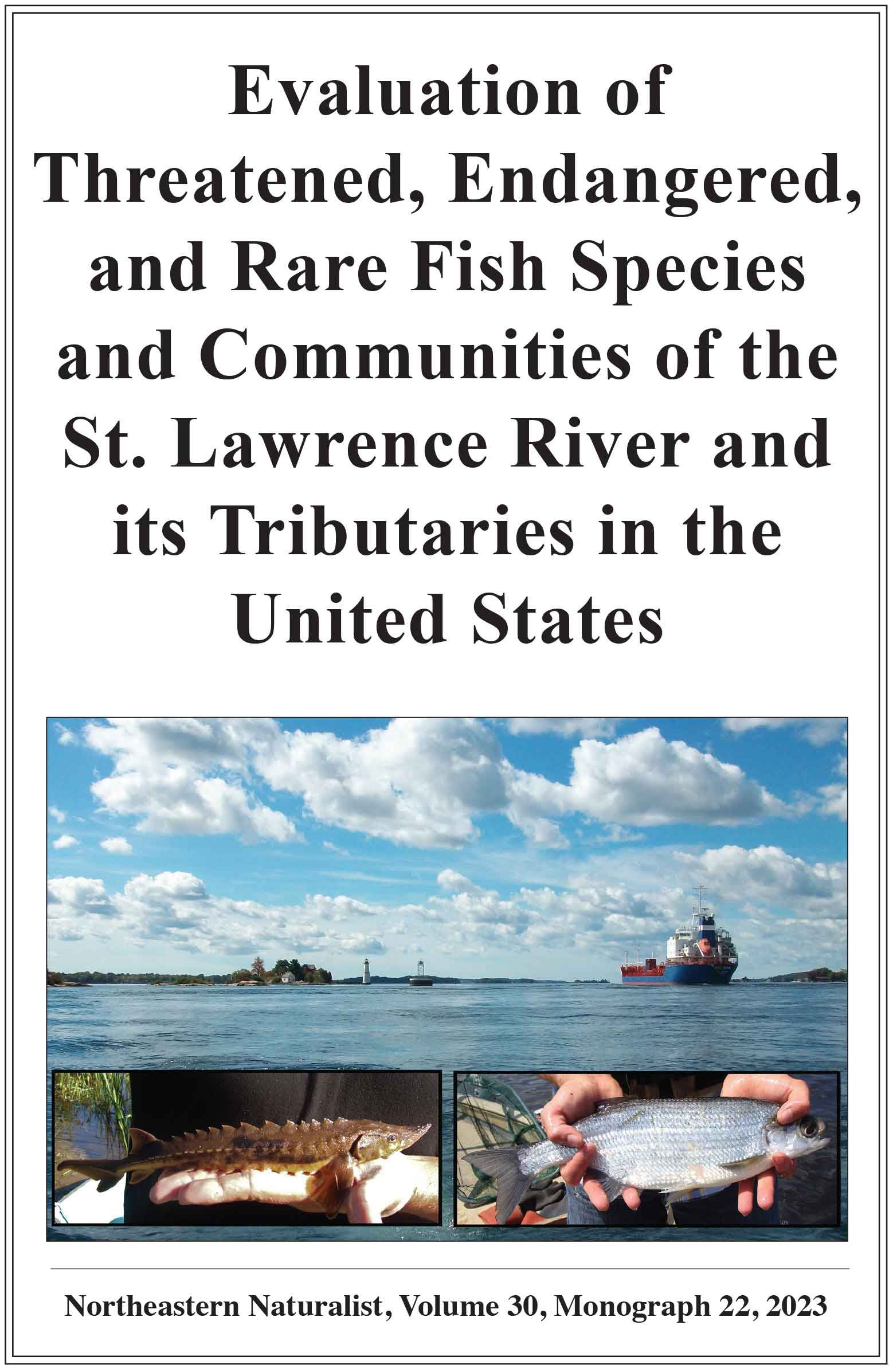
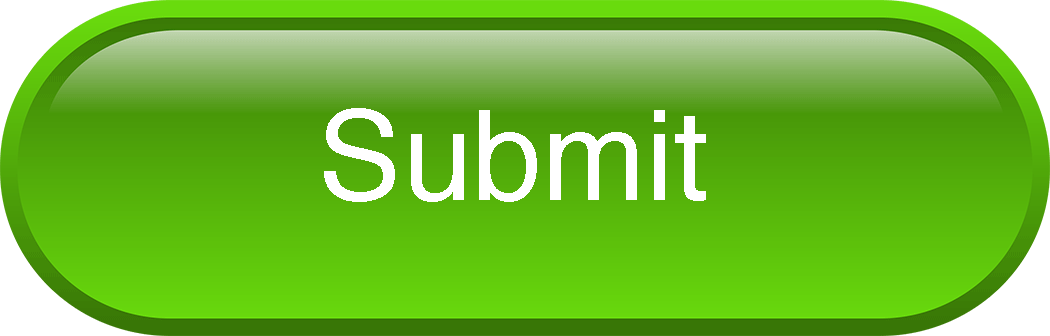


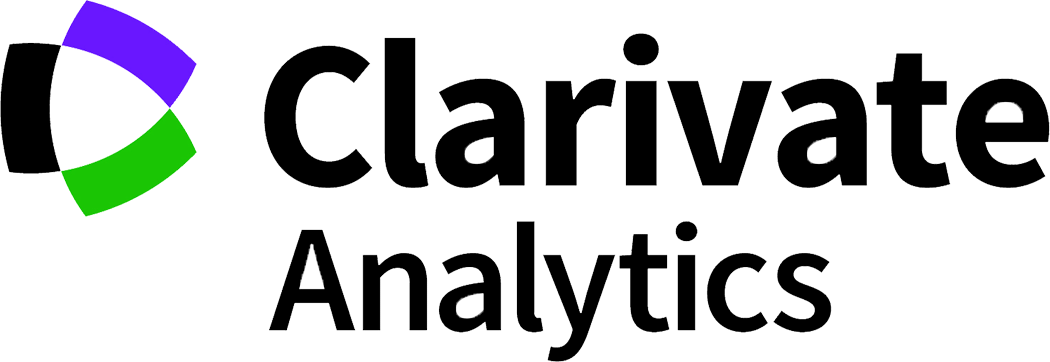
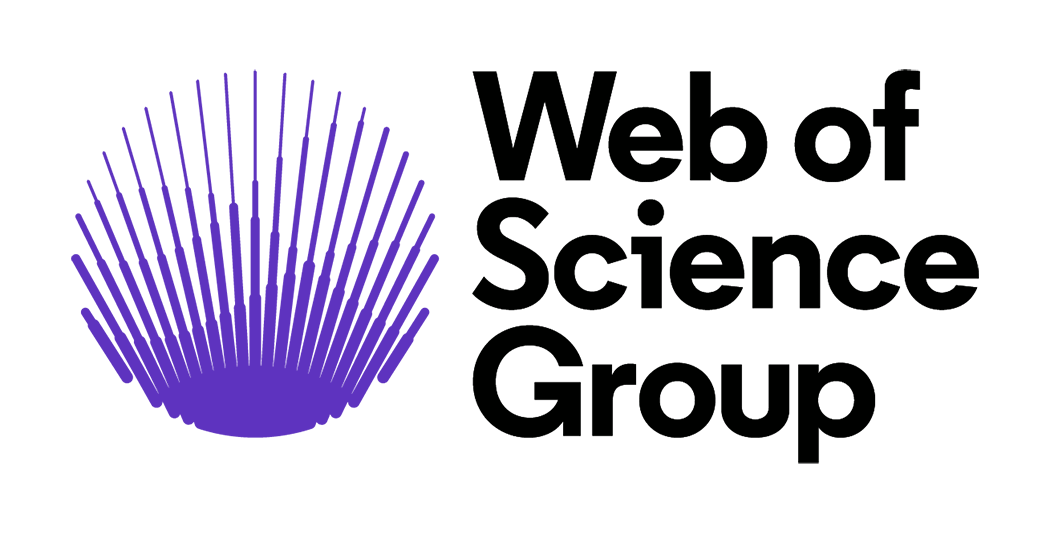
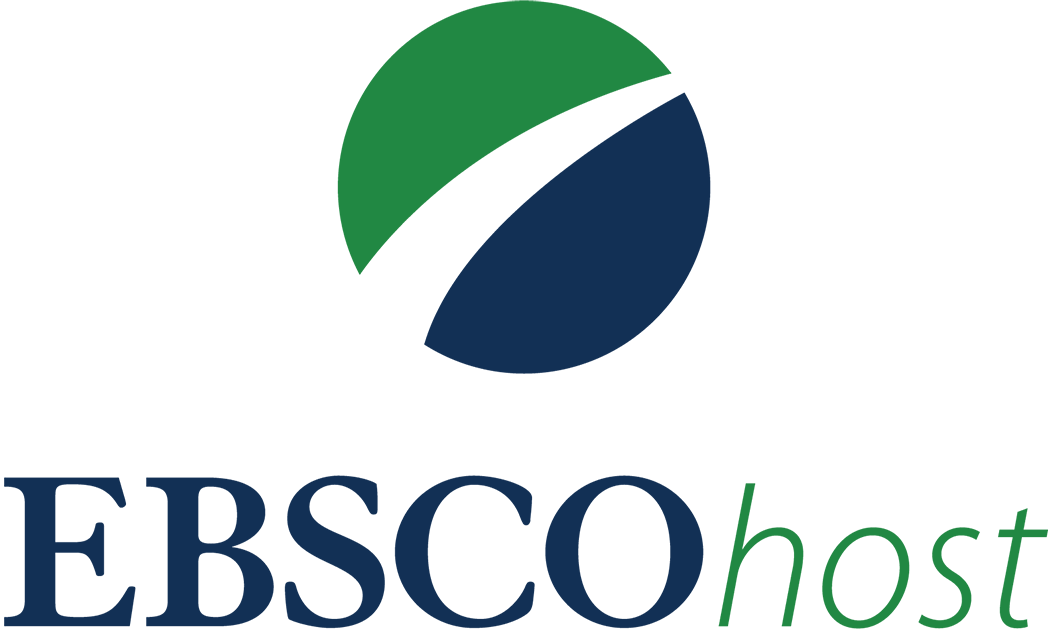
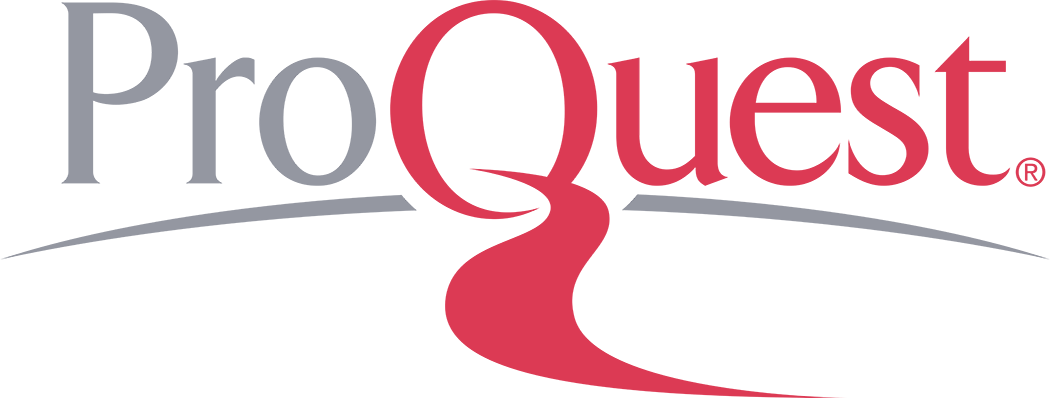
Northeastern Naturalist
192
K.W. Able, T.M. Grothues, J.L. Rackovan, and F.E. Buderman
22001144 NORTHEASTERN NATURALIST 2V1(o2l). :2119,2 N–2o0. 92
Application of Mobile Dual-frequency Identification Sonar
(DIDSON) to Fish in Estuarine Habitats
Kenneth W. Able1,*, Thomas M. Grothues1, Jenna L. Rackovan1,
and Frances E. Buderman2
Abstract - Dual-frequency identification sonar (DIDSON) offers important advantages
over other sampling tools for observing pelagic and benthic fishes in situ. Because it relies
on sound, DIDSON can detect fish in a non-destructive and non-intrusive manner. In our
unique application, the equipment’s small size and low power requirements allow deployment
from a kayak for increased maneuverability in complex habitats. Characteristics that
typify echograms of different fishes can be extracted using multivariate ordination techniques,
such as principal components analysis (PCA), with in situ groundtruthing. Here we
present reference images, techniques, and human-observer–error estimates from DIDSON
application. Together, these approaches enhance our ability to sample fishes and even observe
certain behaviors in complex, turbid environments during a full diel cycle.
Introduction
Our basic lack of knowledge of natural history limits our understanding of the
fauna in many environments (Arnold 2003, Cotterill and Foissner 2010, Dayton
and Sala 2001), particularly in aquatic habitats and especially so in turbid waters
such as estuaries. Conventional sampling techniques are inappropriate for nektonic
fishes in complex, typically turbid estuaries (Brehmer et al. 2003). Under-pier habitats
cannot be sampled effectively with towed nets because pilings offer refuge for
fish (Stoecker et al. 1992), and snags prevent the use of either towed or set (gill)
nets, especially in areas of flow or vessel wake. Larger fish easily avoid cast or pop
nets that are suitable for smaller schooling fishes. Hook-and-line fishing can potentially
determine the species occupying these habitats, but it is non-quantitative and
biased to certain species and to particular times of the day and locations, when and
where fish are feeding. Further, the expected statistically rare occurrence of large
fishes in any habitat requires a wide spatial and temporal sampling effort, which
is rarely spatially discrete. Visual census (by divers or video; e.g., Bortone et al.
2000) is potentially rapid and discreet, but is limited by turbidity, lack of light at
night, and safety issues in many estuaries, especially those in urban settings. Further,
the response of pelagic fish presents some serious challenges to further study
because of their great mobility and clumped distribution due to social behavior and
schooling. Dual-frequency identification sonar (DIDSON) offers many capabilities
to overcome these difficulties.
1Marine Field Station, Institute of Marine and Coastal Sciences, Rutgers University, 132
Great Bay Boulevard, Tuckerton, NJ 08087-2004. 2Department of Fish, Wildlife, and Conservation
Biology, Colorado State University, 1474 Campus Delivery, Fort Collins, CO
80523. *Corresponding author - able@marine.rutgers.edu.
Manuscript Editor: Thomas Maier
Northeastern Naturalist Vol. 21, No. 2
K.W. Able, T.M. Grothues, J.L. Rackovan, and F.E. Buderman
2014
193
In prior studies, DIDSON has been used to characterize habitat (Tiffan et al.
2004), count and measure fish (Becker et al. 2011a, Boswell et al. 2008, Holmes et
al. 2006), study fish and jellyfish behavior (Crossman et al. 2011, Han and Uye 2009,
Kimball et al. 2010, Pavlov et al. 2009, Xie et al. 2008), and estimate escapement
(Holmes et al. 2006). All of these studies were based on static deployment of DIDSON,
with the exception of Handegard and Williams (2008) and Able et al. (2013).
Further, many studies focused on the development of automation in quantification,
which is usually applicable in situations of static or homogenous backgrounds. Our
application of DIDSON, deployed from a moving kayak, provided the opportunity
to survey multiple habitats and their associated fauna over short time-spans in complex
habitats. The purpose of this paper is to present some of the advantages and
disadvantages of DIDSON in a mobile application. More specifically, we identify
fish features that are useful for target classification and provide a preliminary assessment
of the precision of target classification between observers when the use of
automated routines is counterindicated by moving and varying ba ckgrounds.
Materials and Methods
Study area
A portion of the study was carried out in the boat basin of the Rutgers University
Marine Field Station (RUMFS) in Great Bay near Tuckerton in southern New Jersey.
Other observations occurred in New York Harbor along sections of the eastern
shore of the Lower Hudson River along Manhattan. Primary surveyed habitat at this
latter site included overwater piers and uncovered pile fields from relict or collapsed
piers interspersed with open-water areas. See Able et al. (1998, 2010) for further
description of these study sites.
Acoustic techniques
We used DIDSON (Sound Metrics Corp., Seattle, WA) to image fishes. As used
with 96 beams in the high-frequency (1.8 MHz) mode at the applied range of 1.25–10
m out from the lens, the width resolution varied between 6.5 mm and 52.1 mm per
pixel, respectively. The pixel-height resolution depended on the window length,
and at the applied settings of 2.5-m to 10-m window lengths, the height resolution
varied between 4.8 mm and 19.5 mm per pixel, respectively. Thus, a fish 250 mm in
length that was oriented in profile across the screen and 2 m from the lens was represented
by an image 24 acoustic pixels long, but only 4 pixels long if it were 10 m
away from the lens. At this resolution, objects as small as 40 mm could be readily
identified as fishes in the very near field, but would be represented by only 1 pixel in
the extreme downrange. Classification of fish and targets resembling fish required
additional information or closer inspection. At that minimum size, individual fish
were difficult to discern and could not be measured, but schools or aggregations were
apparent. Sampling was conducted at a rate of 5–10 frames per second (fps), dependent
on range, because range setting affected the host software’s processing speed.
A moderate frame rate, typically 7 fps, helped discern moving fishes from a moving
background (actually a static background moving relative to a moving viewer). Fish
movement can be diagnostic, helping to break fish outlines from their background.
Northeastern Naturalist
194
K.W. Able, T.M. Grothues, J.L. Rackovan, and F.E. Buderman
2014 Vol. 21, No. 2
DIDSON images can even detect individual fins in larger fish, which generally have
low reflectance, but which are valuable to identification (Brown et al. 2007). Existing
DIDSON host software (Sound Metrics Corp. version 5.14) supports several tools to
help measure and count detected objects (DIDSON 2006).
In this study, the DIDSON was mounted with a hinge directly under and behind
the bow of a sit-on-top kayak (4 m) for easy access under piers and around pier pilings.
Based on earlier trials, we set the tilt at about 23° for an optimum viewing range
in front of the kayak. This arrangement allowed us to view the structural components
of the habitat bottom, those that extended in the water column (e.g., pier pilings), and
fishes on the bottom and in the water column at both study sites. We carried a splashproof
laptop computer in the kayak cockpit, which allowed real-time viewing so that
the paddler could adjust focus and direction for closer inspection of potential targets.
The paddler used a small red headlamp for navigation during nighttime sampling and
under the darkest piers to minimize the potential effect on the fishes. A nearby motor
skiff, kept outside of the immediate study area, provided logistical support and safety
in the Hudson River study site. Because GPS did not function under the piers, we
noted the position of the kayak using vocal annotation supported by DIDSON host
software to link it with the video. To map fish position, we correlated time stamps
from DIDSON recordings to navigation recordings. Although waters at the study
sites were often calm, especially under large piers or in relict pile fields, episodic ship
wake produced serious pitch and roll, causing smearing artifacts on the echograms.
We note here that use of the term echogram for this video-like dynamic image differs
from its use regarding the standard static echogram where the x-axis is time. The
paddler had to stabilize the kayak during these short but intense disturbances, briefly
interrupting the forward progression along the transect. Such disturbances, however,
were always either preceded or followed by calm periods, so these events never
prevented us from collecting steady echogram sequences useful for measuring and
counting fish.
Groundtruthing and image analysis
In an attempt to determine the congruence between observations made with
DIDSON and more traditional sampling devices, we compared several types of
sampling gear during the summers of 2007 and 2008 at RUMFS. We sampled 73
DIDSON transects during the day and 41 at night ranging from 30 sec to 5 min
in duration in order to capture both large and small pelagic fishes. Samples with
comparison gear included a pop net (n = 16 deployments; Hagan and Able 2003),
experimental traps (n = 30; Able et al. 1998), cast nets (n = 16; Johnston and
Sheaves 2008), and gill nets (n = 3; Able et al. 2009) during day and night. We
used visual observations (n = 60) from the surface (lying on dock next to the kayak
while DIDSON was recording) and while snorkeling. Pop nets, cast nets, and gill
nets were designed to capture fishes in the water column and the experimental traps
were designed to capture benthic fishes. Sampling at night included the use of lights
to attract and retain fishes, per Hagan and Able (2008). We identified and measured
all of the fishes captured with groundtruth sampling gears. In other instances, we
tethered Centropristis striata (Black Sea Bass) and Tautoga onitis (Tautog) to
Northeastern Naturalist Vol. 21, No. 2
K.W. Able, T.M. Grothues, J.L. Rackovan, and F.E. Buderman
2014
195
monofilament line and deployed them in front of the DIDSON sensor to record
their images as reference images for later identification of free-ranging animals. We
compared all of these images to DIDSON deployments from a kayak (n = 114).
These groundtruth samples targeted individual fishes and schools for simultaneous
DIDSON imaging and capture with various gears, and we analyzed them
to determine the accuracy of our identifications and to train observers. The task
was simplified because of the broad overlap between the fish faunas of the two
groundtruthing areas (Able and Fahay 2010). Features of the fish in both study areas
(their position in water column, length, spacing, and orientation) provided metrics
for identifying echogram targets.
We stored DIDSON files with time stamps and metadata in native format and
reviewed them in the laboratory as movies under direct playback control by the
reviewer. Playback controls included frame-advance rate and direction, magnification
(zoom), and graphic user interface tools such as super-position of a grid to
aid counts, and point-drag paths to measure fish lengths. Two or three independent
reviewers viewed the files, then scored and compared their results. Reviewers recorded
each fish-presence event (either school or individual fish). We determined
abundances manually or, for large schools, by making an estimate using the average
number of fish per grid square based on counts of 3 squares (using the superimposed
grid) and multiplying that by the number of squares with fish in them. We also took
a range of measurements for length and body depth of the fish. We calculated both
fish depth in the water column and bottom depth using the following formula based
on the sonar tilt and hypotenuse (tar get distance):
cos (radians (90 - sonar tilt)) * hypotenuse
If the event was a fish school, then reviewers also measured the nearest-neighbor
distance for several individuals chosen at random from the school. Reviewers also
categorized school organization on a ranked basis of 1–4 from highly organized
(parallel swimming, reaction to nearest neighbor as in a school) to random milling
(as in an aggregation; see Pitcher 1983) as a potential metric for identification of
taxon or category.
Each reviewer of the DIDSON files used the matched audio file and written notes,
as well as underwater landmarks visible in the echogram, to reference the position
of the fish relative to pilings or other habitat structures. The reviewer also scored the
amount of debris found on the bottom using a range from 1–4 (low to high) as well as
qualitatively categorizing small and large debris as a measure of structure.
Because we wanted to evaluate the potential effect that observer experience
could have on evaluating DIDSON imagery, we compared results of individual
experienced reviewers against those of an inexperienced reviewer. We assessed
both fish abundance and number of events separately because each is processed
visually by different learned skills. Ordinary least-squares regression of counts
(or events) of any one reviewer against those of another for the same file should
have resulted in a slope (regression coefficient) of 1 if the files were scored the
same by both reviewers. We examined the dispersion of residuals and coefficient
Northeastern Naturalist
196
K.W. Able, T.M. Grothues, J.L. Rackovan, and F.E. Buderman
2014 Vol. 21, No. 2
of determination (r2) even when the slope equalled 1 to determine if the slope
was caused by uniformly similar scores (low error and residual dispersion) from
both reviewers or if errors were large but compensating (unbiased). In a second
analysis, we examined the change in error variance as a function of the number
of reviewers (2 or 3). Because individuals in larger schools of fish are potentially
more difficult to enumerate than those in smaller schools, we standardized error
variance to the coefficient-of-variation (CV). If the use of multiple independent
reviewers was beneficial regardless of experience, then CV should have
decreased as reviewers increased. We chose the files using ranks assigned by a
random-number generator in MS Excel and included those with both relatively
sparse and abundant targets on various backgrounds.
We used the data captured during reviewer-training efforts at RUMFS, when fish
were observed by eye and captured in front of the instrument during DIDSON recording
(i.e., known fish), in a principal components analysis (PCA) to quantify the
importance and confidence in differentiating images into fish taxa/categories. PCA is
a multivariate iterative regression (taxa against image variables and vice versa) that
reduces variation in the composition of samples to the most important latent gradients
(eigenaxes). Graphing the regression coefficients of samples of major eigenaxes
against each other produces an ordination diagram that depicts both the gradient in
character values (such as image depth to length) relative to different taxa and also
the relative contribution of the different characters in defining that order. Further,
the percent variation explained by each of the eigenaxes is quantified as an eigenvalue
(ter Braak and Smilauer 2002). Because the data taken from each image during
review encompassed much of the information that was available to a reviewer who
had not necessarily seen the actual fish, the PCA ordination provided a way of communicating
to readers the relative value of these measures of different characteristics
to the viewer classification-process. Although it is possible to write PCA algorithms
into software code that help classify targets in near-real time (inclusive of, but not
limited to sonar imaging; see Soares et al. 2001, Turhan-Sayan 2005), we did not attempt
that nor did we apply PCA a posteriori to unknown targets.
Results
Image analysis
DIDSON imaging from the kayak during initial groundtruthing at RUMFS
provided mobility when maneuvering around surface structures and clear imagery
of the structural components of habitats. During these deployments, bulkheads,
pier pilings, and remnants of crab pots (rebar frames) were clearly visible on
the bottom and in the water column. We investigated other structures in New
York Harbor based on the same mobility. These objects included miscellaneous
dense (Fig. 1a) and light (Fig. 1b) debris, and an I-beam (Fig. 1c) on the bottom.
A potentially confounding factor in interpretation of DIDSON images was
the frequent release of bubbles from the organically rich sediment in these urban
habitats (Fig. 1d). These were easily resolved from fish in video format, but were
confusing in static images.
Northeastern Naturalist Vol. 21, No. 2
K.W. Able, T.M. Grothues, J.L. Rackovan, and F.E. Buderman
2014
197
For the fish fauna, reviewers documented as many as 5 events (different occurrences
of fishes as individuals or schools or both) on single transects. Reviewers
recorded an event when they detected objects with a discontinuous occurrence and
orientation to different structures within the transect. However, given the mobility
of large fish and the relatively narrow viewing area of the DIDSON, reviewers used
their judgement to determine the independence of these events. The difference in
judgment among experienced and inexperienced reviewers accounted for much
of the experimental error in the number of events counted, with the coefficients
of variations higher for the abundance counts than for event counts (Fig. 2).
Regardless of the type of response measure, the addition of an inexperienced reviewer
resulted in a higher CV than we calculated when there were two experienced
Figure 1. DIDSON images of various structural components on the bottom in the Hudson
River study area. The numbers along the side of each image indicate the distance (m) from the
sensor. The image includes: a) debris (high score of 4) in a piling field near Pier 57, b) light debris
at the edge of a bridge on the New Jersey side of the Hudson River (low score of 2), c) no
debris by a piling under Pier 40 (score of 1) except for I-beam, and d) vertical bubbles (shown
by white ovals) rising from the sediment surrounded by a school of small fish.
Northeastern Naturalist
198
K.W. Able, T.M. Grothues, J.L. Rackovan, and F.E. Buderman
2014 Vol. 21, No. 2
reviewers (Fig 2). Thus, additional review by an inexperienced reviewer did not
increase confidence in the solution obtained by independent, experienced reviewers.
In the regression analysis, the slopes were near unity (c = 1.2) when comparing
fish counts among two experienced reviewers, and less so (c = 1.6) when comparing
event counts among experienced reviewers. The slopes were similar if one of the
reviewers was experienced and one was inexperienced (c = 1.2 and 1.3 for count
and event count, respectively). However, the coefficient of determination was much
stronger for experienced reviewers (r2 = 0.65 and 0.68, vs. 0.50 and 0.30, for count
and event count, respectively). This result indicated that the error variance was
much greater when using inexperienced reviewers, but that the error was unbiased;
reviewers counted both too many and too few fish (or events) in equal proportion.
The splitting or lumping of events did not change the overall estimate of abundance.
For example, one reviewer might have counted 3 individual fish as 2 events
(e.g., a singleton and a pair), while another reviewer counted 3 fish as 3 events.
Both reviewers arrived at the same fish count (i.e., 3); however, this could affect
the average count per event, which was not scored in our study. Another source of
variation in abundance came from the estimation of total number from subsets in
large schools.
Identification of fish taxa and categories
We compared the results of fish identifications made using DIDSON imagery
collected during groundtruthing at RUMFS with the results from samples collected
using a variety of gears including pop nets, cast nets, and gill nets for pelagic fishes,
Figure 2. Average (bar) and standard error (error bars) of fish-response measures (abundance
and events) as counted by 2 or 3 reviewers for a random selection of DIDSON files.
The average coefficient of variation for each case is also shown. In all test cases, two reviewers
were experienced and another was considered an inexperi enced reviewer.
Northeastern Naturalist Vol. 21, No. 2
K.W. Able, T.M. Grothues, J.L. Rackovan, and F.E. Buderman
2014
199
and experimental traps for benthic fishes during the day and night (Table 1). Of the
15 species/taxa collected across all groundtruthing gears in the RUMFS boat basin,
reviewers of the congruent DIDSON files identified only 5 fish species, including
Brevoortia tyrannus (Atlantic Menhaden), Black Sea Bass, Menidia menidia
(Atlantic Silverside), Tautog, and Pomatomus saltatrix (Bluefish) (Table 1). It is
perhaps not surprising that reviewers of DIDSON imagery did not identify several
less-common species (e.g., Alosa mediocris [Hickory Shad] and additional Tautog
individuals). However, several abundant species were also not identified—Anchoa
mitchilli (Bay Anchovy), Alosa pseudoharengus (Alewife), Fundulus heteroclitus
(Mummichog), Sphyreana borealis (Northern Sennet), and Tautogalabrus adspersus
(Cunner). In addition, Bay Anchovy were of the same size and mixed with
aggregations of the more abundant Atlantic Silverside, as identified by cast-net
samples in front of the DIDSON, but individuals of these species could not be differentiated
in DIDSON files. However, we identified a small shark with the DIDSON
during groundtruthing at RUMFS (likely Mustelus canis (Mitchill) [Smooth
Dogfish] , based on the presence of a heterocercal caudal fin and the subcarangiform
swimming motion), which was not collected in groundtruthing nets. Several species
collected in groundtruth sampling were too small to have been detected with
DIDSON (e.g., Gobiosoma sp. [goby]) or cryptic on the bottom or buried (e.g.,
Pseudopleuronectes americanus [Winter Flounder]). Thus, the ability of DIDSON
to identify the diversity of estuarine fishes is limited to a few distinctive forms and,
in this application, it was easier to detect and identify lar ger individuals.
When these forms were subdivided by some aspects of behavior (benthic, pelagic)
and size, using a cutoff for maximum size of mature Atlantic Silverside and Bay
Table 1. Fish-species composition and relative abundance by groundtruthing and from DIDSON images
based on sampling in the Rutgers University Marine Field Station boat-basin and nearby areas in
southern New Jersey. x indicates species identified but not counted.
Groundtruth method
Pop Cast Gill
Species/Taxon net net Trap net Visual DIDSON
Anchoa mitchilli Valenciennes (Bay Anchovy) 8 1 0 0 0
Anchoa sp. 1 0 0 0 0
Alosa pseudoharengus Wilson (Alewife) 1 11 0 0 0
Alosa mediocris Mitchill (Hickory Shad) 1 0 0 0 0
Brevoortia tyrannus Latrobe (Atlantic Menhaden) 1 0 0 0 0 x
Centropristis striata L. (Black Sea Bass) 0 0 3 0 x x
Fundulus heteroclitus L. (Mummichog) 0 2 24 0 0
Gobiosoma sp. 1 0 2 0 0
Menidia menidia L. (Atlantic Silverside) 232 2 2 0 x x
Pomatomus saltatrix L. (Bluefish) 8 5 0 3 0 x
Pseudopleuronectes americanus Walbaum 1 0 1 0 0
(Winter Flounder)
Sphyraena borealis DeKay (Northern Sennet) 14 0 0 0 0
Tautoga onitis L. (Tautog) 0 0 1 0 x x
Tautogolabrus adspersus Walbaum (Cunner) 0 0 16 0 2
Unidentified Clupeid 2 1 0 0 0
Northeastern Naturalist
200
K.W. Able, T.M. Grothues, J.L. Rackovan, and F.E. Buderman
2014 Vol. 21, No. 2
Anchovy (<250 mm), we were able to sort them into reasonably discrete categories
that could be reliably replicated (Table 2). We made our distinction between schools
and aggregations (Fig. 3) based on the organization of individuals relative to each
other. Schools included objects of similar size that were tightly organized with
small distances between individuals. Aggregations were often comprised of slightly
dissimilarly sized objects with multiple orientations of individuals. We classified
schools and aggregations as large if they contained >50 individuals, otherwise we
considered them to be small. We determined that these categories would be useful
for gauging responses of fish in estuaries and for use in other complex habitat assessments
in future studies.
Although the details necessary for identification with DIDSON were less distinguishable
for smaller fish, behaviors observed in the RUMFS boat-basin added to
our ability to identify categories or even species. We compared variation in school
organization, size distribution, and individual fish spacing in echograms of schools
of small fish to composition of individuals in net captures during groundtruth efforts
and visual observations at RUMFS (Table 1). Resolution of these known fish by
PCA was moderate; the primary eigenaxis explained 29% of the variation, the second
eigenaxis explained an additional 15%, and the third and fourth explained 14%
and 12%, respectively (Fig. 4, Table 3). Thus, a 2-dimensional biplot representation
shows only 44% of the cumulative variation, but patterns explaining the additional
variation are available from individual axis-loading factors (Table 3). The number
of fish in the school was one of the most important driving variables of the first
eigenaxis: cumulative fit (cfit) as a fraction of variance = 0.59; the biggest schools
of fish in these samples were composed of small Atlantic Menhaden alone or mixed
with their predator, Bluefish. The biggest fish, however (typically Bluefish), tended
to be alone; thus, another important factor of the first eigenaxis was mean (or
Table 2. Definition of categories of fish taxa/ecological groups identified from DIDSON files during
preliminary groundtruthing efforts in the Rutgers University Marine Field Station boat-basin and the
Hudson River. These categories do not require identification of individual species, but do provide a
standardized characterization of fishes for detailed analysis.
Category Definition
Benthic fish Benthic, often finning but not swimming, no size limit, not
oriented to each other
Small aggregation of small pelagic fish Swimming in water column, <50 individuals, average
length <250 mm
Large aggregation of small pelagic fish Swimming in water column, >50 individuals, average
length <250 mm
Small school of small pelagic fish Swimming together in water column, <50 individuals,
average length <250 mm
Large school of small pelagic fish Swimming together in water column, >50 individuals,
average length <250 mm
Single small pelagic fish Swimming in water column, <250 mm
Single large pelagic fish Swimming in water column, >250 mm
School of large pelagic fish Swimming together in water column, average length >250
mm
Unidentified pelagic fish Single fish in water column, measurement not possible
Northeastern Naturalist Vol. 21, No. 2
K.W. Able, T.M. Grothues, J.L. Rackovan, and F.E. Buderman
2014
201
individual) fish length (cfit = 0.58), but this factor was highly inversely correlated
with school size (Table 3, Fig. 3).Variables relating to school organization and the
ratio of the imaged body depth to imaged length (aspect ratio), explained variation
on the second axis (Fig.3). The mean distance of a fish to its nearest neighbor (cfit
to axis 2 = 0.69), was highly correlated to the distance to the next nearest neighbor
(cfit = 0.66, Table 3), and reflected the fact that deep-bodied Atlantic Menhaden
(which present a relatively high aspect ratio) had a regular spacing among individuals,
yet were not always oriented in the same direction. In contrast, Atlantic
Silversides, also with uniform (but less) space between individuals, tended to swim
as a group with high polarity in organization (cfit = 0.48). Given the sonar tilt, we
did not attempt to match the measured aspect ratio of the echogram image with
how it translated for the actual fish. The spacing among individuals of an event
had a strong effect on both eigenaxes, but it was highly conditional on the presence
Figure 3. DIDSON images of various fish schools from the Hudson River study area including:
a) large (>50) organized school with 200+ individual fish (4–9 cm in length) near
pilings at Pier 40, b) large (>50) unorganized aggregation of fish (6–10 cm in length) near
Pier 40, c) small (less than 50) organized school of 40+ fish (7–9 cm in length) near pilings at Pier
57, and d) large aggregation of 200+ fish (8–10 cm in length) mixed with bubbles at the
edge of Pier 40.
Northeastern Naturalist
202
K.W. Able, T.M. Grothues, J.L. Rackovan, and F.E. Buderman
2014 Vol. 21, No. 2
of predators, i.e., schools of Atlantic Silverside were scattered by Bluefish when
an aggregation of mixed species was viewed as an event, whereas they could be
slightly organized to highly organized when they could be viewed continuously. By
themselves, Bluefish were highly unorganized, with individuals appearing to move
Table 3. Cumulative fit per variable as fraction of variance in PCA of groundtruthing data.Values
indicate the importance of specific measures in resolving the position of different fish classes along a
particular axis, and the frequency fitted indicates the relative importance of each axis.
Variable Axis 1 Axis 2 Axis 3 Axis 4
Frequency fitted 0.2932 0.1502 0.1387 0.1221
Number of individuals 0.5861 0.6009 0.6408 0.6460
Orientation 0 (two or fewer) 0.1468 0.3101 0.3775 0.5100
Highly ordered 0.0723 0.4819 0.5144 0.7242
Moderately ordered 0.0008 0.0165 0.5203 0.6182
Slightly ordered 0.0880 0.2636 0.2844 0.9110
Random 0.2042 0.2744 0.4707 0.6093
Mean fish length 0.5775 0.5894 0.7778 0.8299
Mean fish height 0.4770 0.7162 0.7168 0.8232
Mean fish aspect ratio 0.0005 0.2746 0.7072 0.7238
Distance to nearest neighbor 0.5076 0.6916 0.7581 0.7654
Distance to next nearest neighbor 0.4472 0.6632 0.7088 0.7366
Depth of fish or school 0.4101 0.4377 0.5082 0.5526
Figure 4. Principle
components analysis
(PCA) of fish classification
from DIDSON
images of schooling
fish as groundtruthed
by net sampling and visual
observations at the
Rutgers University Marine
Field Station boatbasin
in Great Bay near
Tuckerton, NJ. Menidia
menidia (Atlantic Silverside)
were common
in groundtruth samples
and exhibited a wide
size range and suite
of organization characteristics,
but could be
readily discerned from
deeper bodied fish such
as Brevoortia tyrannus
(Atlantic Menhaden)
and Pomatomus saltatrix
(Bluefish).
Northeastern Naturalist Vol. 21, No. 2
K.W. Able, T.M. Grothues, J.L. Rackovan, and F.E. Buderman
2014
203
independently of other visible fishes; this behavior, also expressed as a low number
of individuals per event (making them occur as singletons), served to differentiate
them in the PCA. Atlantic Silverside schools took on many different school forms
based on the time of day and disturbance from predatory Bluefish or groundtruthing
activity as well as from unknown factors. These conditional factors altered relationships
seen in the first and second axes so that the major drivers could be important
but differently correlated on the third and fourth eigenaxes, a reason for the poor
2-dimensional resolution of the PCA biplot (Table 3).
In the Hudson River, other approaches by image reviewers helped differentiate
some species. For our identification of large fish, both as schools and individuals
(Fig. 5a, b), we relied on characteristics such as caudal fin shape, number of dorsal
fins, and shape of pectoral fins, as well as swimming behavior. In many cases, large
Figure 5. DIDSON images in the vicinity of piers in the Hudson River. The numbers along
the side of each image indicate the distance (m) from the sensor. The images include: a)
a school of unidentified large fish (25–30 cm in length) between 4–5 m from the sensor,
and acoustic shadows of the same fish at 5–6 m from the sensor; b) a small school of large
(30–40 cm in length) fish at approximately 2–2.5 m from the sensor, with a blurred acoustic
shadow of the same fish at 5–6 m; c) a school of large (30–40 cm in length) Striped Bass
(Morone saxatilis). Note that the dorsal view of the individual (65–70 cm in length; indicated
by white oval) at 4 m from the sensor provides a view of the pectoral fins; and d) a
single large (70–80 cm in length) Striped Bass (indicated by white oval) at approximately 5
m from the sensor with both dorsal fins evident.
Northeastern Naturalist
204
K.W. Able, T.M. Grothues, J.L. Rackovan, and F.E. Buderman
2014 Vol. 21, No. 2
Morone saxatilis (Walbaum) (Striped Bass) were readily identified in DIDSON
sonograms by the presence of two separate dorsal fins, shallow forked tails, and
thick bodies when seen in dorsal view (Fig. 5c, d). In some cases, large (≈1 m TL)
Bluefish were distinguishable from Striped Bass based on profile. Identification
was aided, in some instances, by the acoustic shadows cast by structural components
of the piers (e.g., pilings, Fig. 1) and of the fishes (Fig. 5). Nonetheless,
DIDSON file reviewers were often unable to confidently identify other individuals
that may have been Striped Bass or other long perciform fishes, such as Bluefish
or Cynoscion regalis (Bloch and Schneider) (Weakfish), largely because they were
actively swimming and quickly left the field of view. Additionally, while actively
swimming, all 3 of these perciform fishes lower their first (spiny) dorsal fin and fold
their forked caudal fin to a narrower profile, making species-specific identification
difficult. Further, all of these species commonly overlap in size range and timing of
occurrence in estuaries. As a group, these 3 species would have been distinguishable
from some other perciformes that may occur in the estuaries (e.g., Pogonias
cromis L. [Black Drum]), based on shape or maximum size, or both, but we did
not observe fishes of such distinguishable forms. Reviewers identified several individuals
to a type that suggested either Black Sea Bass or Tautog on the basis of
stiff-bodied (pectoral sculling) swimming movements and contact with the bottom
as seen during visual groundtruthing. These species and small Cunner could also be
difficult to detect by DIDSON if they did not move. In another instance, an Anguilla
rostrata (LeSueur) (American Eel) was clearly visible to reviewers, but this species
was not detected by any of the groundtruth sampling, although it has been captured
previously at the Hudson River study site (Able and Duf fy-Anderson 2005).
Discussion
Prior studies with DIDSON techniques have demonstrated both advantages and
disadvantages of the technology, which vary with type of deployment (static vs.
mobile), orientation of the sensor, and range settings at deployment. Most previous
attempts to assess fish abundance, biomass, and avoidance (Becker et al. 2011a,
Boswell et al. 2008, Crossman et al. 2011, Han and Uye 2009, Holmes et al. 2006,
Kimball et al. 2010, Xie et al. 2008) were made from a static location (but see Able
et al. 2013, Handegard and Williams 2008) with few attempts to identify faunal
components. Visual surveys, as approximated by DIDSON’s sonar-to-image processing,
may also be biased against some types of fishes by behavioral traits that
limit their detection, such as swimming immediately under the surface, associating
closely with the bottom, or by patterns of movement or burial. These same issues
have also been identified as problems for visual or video census (Bortone et al.
2000; Sullivan et al. 2000, 2003).
One major concern when using DIDSON is the potential for counting-bias. Sedentary
species (or those active, but with orientation to a small core area) are best
counted by transect designs, but very active swimmers are best counted by fixedradius
or point-count surveys (as described by Petit et al. [1995] for forest birds).
Also, benthic species may be cryptic in complex backgrounds, either because of a
Northeastern Naturalist Vol. 21, No. 2
K.W. Able, T.M. Grothues, J.L. Rackovan, and F.E. Buderman
2014
205
loss of color and pattern information with DIDSON, or because they actually hide.
Therefore, we focused only on pelagic species when surveying fish response to pier
shading with DIDSON (Able et al. 2013). Another shortcoming has been DIDSON’s
inability to detect small (less than 40 mm) fishes (Boswell et al. 2008) relative to traditional
capture techniques, or to effectively separate these from bubbles, acoustic noise,
and non-fish particulates. These problems can be resolved with higher-resolution
exchangable auxiliary lenses that concentrate or spread the beams at 1, 3, 8, or 28
degrees (J. Dorsey, Ocean Marine Industries, Inc., Chesapeake, VA, pers. comm.).
Finally, as with other acoustic techniques, studies employing DIDSON benefit
greatly from the sometimes labor-intensive practice of groundtruthing (Brehmer et
al. 2006).
Despite these shortcomings, the advantages of DIDSON are numerous. This study
extends DIDSON’s utility by demonstrating the effectiveness of surveying fauna
from a mobile platform, pinpointing characters that can enhance the identification of
fishes, and evaluating reviewer effects on image analysis. DIDSON images can be
generated with similar clarity during the day and night, and regardless of the degree
of turbidity in the water column, at least in the two estuaries studied here. DIDSON
mounted on a kayak provides exceptional mobility, even in very shallow water,
something that is not possible with most powered-boat platforms. Additionally, DIDSON
does not disturb or attract fauna, as the light necessary for typical video imaging
often does. We have seldom observed a fright response by larger predators or smaller
prey fish while within the range of DIDSON (typically 1.5–7 m), and have witnessed
predation and other social interactions that suggest fish were unconcerned with our
presence. In the only other study of this type, which utilized a motorboat, fish exhibited
a disturbance response (Xie et al. 2008).
In addition, DIDSON software provides the possibility of making fish counts
and length measurements from collected images (see above Discussion for caveats).
For example, in a South African estuary, researchers used standard DIDSON
metrics—size, abundance, and schooling behavior—to infer ecological function
relative to turbidity (Becker et al. 2011a) and day–night behavior (Becker et al.
2011b). Further, images of the undisturbed fauna taken from a kayak allow continuous
observations that provide details regarding fish schools, shoals, and aggregations
(Pitcher 1983), their surface area (Brehmer et al. 2006), and the morphology
of individual fish, including the shape of the body and head, the number of dorsal
fins, and shape of caudal fins for larger fish. This information is especially helpful
because large fish are the most challenging to sample, due to their relative rarity.
In addition, continuous imaging provides aspects of swimming behavior, such as
mode of propulsion (e.g., carangiform, anguilliform, fin locomotion), degree of
schooling, and predator–prey interactions, which can aid identification and give insights
into ecological interactions. Thus, DIDSON use is similar to camera use with
image–recognition software (Lee et al. 2008, Rova and Dill 2007, Strachan 1993),
without the benefit of color. Like video, the data produced can either be enhanced
by movement, in the case of static backgrounds, or distorted by the movement of
artifacts in moving backgrounds.
Northeastern Naturalist
206
K.W. Able, T.M. Grothues, J.L. Rackovan, and F.E. Buderman
2014 Vol. 21, No. 2
The dynamics of motion are difficult to express; thus, experience is important in
reducing error associated with any DIDSON fish-census from a moving platform. A
number of potential measurements taken from static DIDSON freezeframes relate
to movements as derivatives (such as measuring distance among nearest neigbors in
a school) and can therefore be codified into multivariate ordinations such as PCA.
However, even a PCA on our visually groundtruthed data set described considerable
overlap among fish, and much of this related to motion and changes of school
structure based on the presence of predators. Directly quantifying movement is
possible and even routine, as evidenced by the tools provided within the DIDSON
software package (DIDSON 2006), but it becomes much more difficult with the
currently available software when the background moves relative to the viewer.
Thus, the PCA is very useful as a communication tool for quantifying what a reviewer
sees in a static DIDSON image, but is not yet a tool for use in automation
in a mobile application.
Although we have discussed the disadvantages of a heterogeneous and moving
background, we note that this belies an important advantage that DIDSON brings
to fish surveys, i.e., the ability to simultaneously sample physical habitat structure
(Fig. 1). There are few other sampling methods that can do this. Movement also
helped in classification, because, unlike a static deployment, it offered different
perspectives. One example is in viewing bubbles, which can be confused with small
fish. Bubbles, like fish, move in both the horizontal and vertical dimensions because
of movement of the kayak and because even very weak currents displace them, and
so are difficult to discern, even when using the classic static echogram (time on the
x-axis) DIDSON software feature. Bubbles, however, do not have tail beats, which
are evident during playback, with even fairly small fish.
Future improvements and applications
The DIDSON approach, used here to focus on piers and other shallow water
habitats, can be applied to other complex urban and natural shoreline habitats, and
classification schemes can be constructed and their confidence quantified by extension
of the methods presented herein. For example, canonical variates analysis
(CVA) uses the same multiple regression algorithm, but combines a known data
set with a previously identified data set so that the percent correct identifications
of a test set can be quantified. These approaches, including the groundtruthing
techniques we have applied in this study and those of others using static DIDSON
deployments (e.g., Becker et al. 2011a, b), offer the possibility of determining total
juvenile and adult fish-abundance (to some lower size limit), biomass, species or
categories composition (with further experience), and selected behavior (schooling,
predator–prey, and other social interactions). Together, these approaches can
provide parameter estimates during either day or night, which has seldom been accomplished
in previous aquatic studies in natural or urbanized habitats (Able and
Fahay 2010).
Future improvements will further expand DIDSON capabilities when coupled
with enhanced groundtruthing (which may need to be location- and habitatspecific
for benthic and pelagic fish). Continued development of a static-image
Northeastern Naturalist Vol. 21, No. 2
K.W. Able, T.M. Grothues, J.L. Rackovan, and F.E. Buderman
2014
207
and movie-clip reference library will expedite the applicability of DIDSON
techniques. Enhanced processing power will expand the size range and other capabilities
of this system, e.g., higher processor speed will allow tail-beat counts
in naturally turbid or dark environments (Mueller et al. 2010). DIDSON recordings
from transects can now be merged into mosaics using image landmarks as
common reference points without the use of external spatial referencing. Finally,
emerging competitive products offering different resolution and pricing will
encourage further development and niche-application diversification, including
large and small robotic vehicle mounts, diver units with mask displays, and integration
with acoustic telemetry technology that identifies acoustically tagged fish
with a screen icon (Larry Egan, Lotek Wireless Inc., St. Johns, NL, Canada, pers.
comm.). All of these improvements could further expand our understanding of
fishes and turbid underwater habitats.
Acknowledgments
Numerous individuals assisted in these efforts, including summer interns Merrill Rudd
and Iris Kemp and volunteers Pat Filardi and Steve Zeck. Technicians from the Rutgers
University Marine Field Station—Tom Malatesta, Jamie Caridad, Jen Smith, and Matt
Taynor—participated in the field sampling in sometimes trying conditions during the day
and night. Franny Buderman conducted preliminary analysis of Pier 57 data as part of her
NSF-REU at Rutgers University Research Internships in Ocean Science (RIOS) internship.
Hudson River Park Trust personnel assisted with the permitting process. Four anonymous
reviewers provided helpful comments on this manuscript, as did the manuscript editor,
Thomas J. Maier. Funding for this project was provided by the Hudson River Foundation.
This paper is Rutgers University Institute of Marine and Coastal Science Contribution
No. 2013-6.
Literature Cited
Able, K.W., and J.T. Duffy-Anderson. 2005. A synthesis of impacts of piers on juvenile fishes
and selected invertebrates in the Lower Hudson River. Rutgers University, Institute
of Marine and Coastal Sciences Technical Report #2005-13, New Brunswick, NJ. 38 pp.
Able, K.W, and M.P. Fahay. 2010. Ecology of Estuarine Fishes. Johns Hopkins Press, Bal -
timore, MD. 566 pp.
Able, K.W., J.P. Manderson, and A.L. Studholme. 1998. The distribution of shallow-water
juvenile fishes in an urban estuary: The effects of man-made structures in the lower
Hudson River. Estuaries 21(4b):731–744.
Able, K.W., K.M.M. Jones, and D.A. Fox. 2009. Large nektonic fishes in marsh-creek habitats
in the Delaware Bay estuary. Northeastern Naturalist 16(1):27–44.
Able, K.W., J. Dobarro, and A.M. Muzeni-Corino. 2010. An evaluation of boat-basin
dredging effects: Response of fishes and crabs in a New Jersey estuary. North American
Journal of Fisheries Management 30:1001–1015.
Able, K. W., T.M. Grothues, and I.M. Kemp. 2013. Fine-scale distribution of pelagic fishes
relative to a large urban pier. Marine Ecology Progress Series 476:185–198.
Arnold, S.J. 2003. Too much natural history, or too little? Animal Behavior 65:1065–1068.
Northeastern Naturalist
208
K.W. Able, T.M. Grothues, J.L. Rackovan, and F.E. Buderman
2014 Vol. 21, No. 2
Becker, A., A.K. Whitfield, P.D. Cowley, J. Jarnegren, and T.F. Naesje. 2011a. An assessment
of the size structure, distribution, and behavior of fish populations within a temporarily
closed estuary using dual-frequency identification sonar (DIDSON). Journal of
Fish Biology 79:761–775.
Becker, A., P.D. Cowley, A.K. Whitfield, J. Jarnegren, and T.F. Naesje. 2011b. Diel fish
movements in the littoral zone of a temporarily closed South African estuary. Journal of
Experimental Marine Biology and Ecology 406:63–70.
Bortone, S.A., M.A. Samoilys, and P. Francour. 2000. Fish and macroinvertebrate assessment
methods. Pp. 127–164, In W. Seaman, Jr. (Ed.). Artificial Reef Evaluation. CRC
Press, Boca Raton, FL. 264 pp.
Boswell, K.M, M.P. Wilson, and J.H. Cowan. 2008. A semi-automated approach to estimating
fish size, abundance, and behavior from dual-frequency identification sonar (DIDSON)
data. Journal of Fisheries Management 28:799–807.
Brehmer, P., F. Gerlotto, J. Buillard, F. Sanguinéde, Y. Guénnegan, and D. Buestel. 2003.
New applications of hydroacoustic methods for monitoring shallow-water aquatic ecosystems:
The case of mussel-culture grounds. Aquatic Living Resources 16:333–338.
Brehmer, P., T.D. Chi, and D. Mouillot. 2006. Amphidromous-fish-school migration revealed
by combining fixed sonar monitoring (horizontal beaming) with fishing data.
Journal of Experimental Marine Biology and Ecology 334:139–150.
Brown, L.M., K.J. Magowan, D.A. Fox, and J.E. Hightower. 2007. Comparison of splitbeam
and hydroacoustic gears for conducting sturgeon surveys. Presented at Mid-
Atlantic Chapter of the American Fisheries Society Meeting, Lewes, DE, 1–3 February.
Cotterill, F.P.D., and W. Foissner. 2010. A pervasive denigration of natural history misconstrues
how biodiversity inventories and taxonomy underpin scientific knowledge.
Biodiversity and Conservation 19:291–303.
Crossman, J.A., G. Martel, P.N. Johnson, and K. Bray. 2011. The use of dual-frequency
identification sonar (DIDSON) to document White Sturgeon activity in the Columbia
River, Canada. Journal of Applied Ichthyology 27 (Suppl.2):53–57.
Dayton, P., and E. Sala. 2001. Natural history: The sense of wonder, creativity, and progress
in ecology. Scientia Marina 65 (Suppl 2):199–206.
DIDSON 2006. Fish-assessment selected advanced tools tutorial version 5.07.01 or later,
Sound Metrics Corp, Bellevue, WA.
Hagan, S.M., and K.W. Able. 2003. Seasonal changes of the pelagic fish assemblage in a
temperate estuary. Estuarine, Coastal, and Shelf Science 56:15-29.
Hagan, S.M., and K.W. Able. 2008. Diel variation in the pelagic-fish assemblage in a temperate
estuary. Estuaries and Coasts 31:3–42.
Han, C-H., and S-I. Uye. 2009. Quantification of the abundance and distribution of the common
jellyfish Aurelia aurita s.l. with a dual-frequency identification sonar (DIDSON).
Journal of Plantkon Research 31(8):805–814.
Handegard, N.O., and K. Williams. 2008. Automated tracking of fish trawls using DIDSON
(dual-frequency identification sonar). ICES Journal of Marine Sc ience 65:636–644.
Holmes, J.A., G.M.W. Cronkite, H.J. Enzenhofer, and T.J. Mulligan. 2006. Accuracy and
precision of fish-count data from a dual-frequency identification sonar (DIDSON) imaging
system. ICES Journal of Marine Science 63:543–555.
Johnston, R., and M. Sheaves. 2008. Cross-channel distribution of small fish in tropical
and subtropical coastal wetlands is trophic-, taxonomic-, and wetland-depth-dependent.
Marine Ecology Progress Series 357:255–270.
Northeastern Naturalist Vol. 21, No. 2
K.W. Able, T.M. Grothues, J.L. Rackovan, and F.E. Buderman
2014
209
Kimball, M.E., L.P. Rozas, K.M. Boswell, and J.H. Cowan, Jr. 2010. Evaluating the effect of
slot size and environmental variables on the passage of estuarine nekton through a watercontrol
structure. Journal of Experimental Marine Biology and Ecology 395:181–190.
Lee, D-J., J.K. Archibald, R.B. Schoenberger, A.W. Dennis, D.K.Shiozawa. 2008 Contour
matching for fish-species recognition and migration monitoring. Applications of Computational
Intelligence in Biology. 122:183–207
Mueller, A., D.L. Burwen, K.M. Boswell, and T. Mulligan. 2010. Tail-beat pattern in dualfrequency
identification sonar sonograms and their potential use for species identification
and bioenergetic studies. Transactions of the American Fisheries Society 139:900–910.
Pavlov, D.S., E.S. Borisenko, and V.M. Pashin. 2009. Investigations of spawning migration
and assessment of abundance of the Kamchatka Steelhead (Parasalmo mykiss) from the
Utkholok River by means of DIDSON dual-frequency identification sonar. Journal of
Ichthyology 49(11):1042–1064.
Petit, D.R., L.J. Petit, V.A. Saab, and T.E. Martin. 1995. Fixed-radius point-counts in forests:
Factors influencing effectiveness and efficiency. Pp. 49–56, In C.J. Ralph, J.R.
Sauer, and S. Droege (Eds.). Monitoring Bird Populations by Point Counts. USDA Forest
Service General Technical Report PSW-GTR-149. USDA Forest Service, Southwest
Research Station, Albany, CA 181 pp.
Pitcher, T.J. 1983. Heuristic definitions of shoaling behavior. Animal Behaviour 31:611–613.
Rova, G.M., and L.M. Dill. 2007. One fish, two fish, butterfish, trumpeter: Recognizing fish
in underwater video. In IAPR Conference on Machine Vision Applications. Pp 404–407.
Soares Filho, W., J. Manoel de Seixas, and L.Pereira Caloba. 2001. Principal component
analysis for classifying passive sonar signals. International Symposium on Circuits and
Systems, Sydney, Australia, 2:592–595.
Stoecker, R.R., J. Collura, and P.J. Fallon, Jr. 1992. Aquatic studies at the Hudson River
Center Site. Pp. 407–427, In C.L. Smith (Ed.). Estuarine Research in the 1980s. The
Hudson River Environmental Society Seventh Symposium on Hudson River Ecology,
State University of New York Press, Albany, NY.
Strachan, N.J.C. 1993. Recognition of fish species by colour and shape. Image Vision Computing
11(1):2–10
Sullivan, M.C., R.K.Cowen, K.W. Able, and M.P. Fahay. 2000. Spatial scaling of recruitment
in four continental-shelf fishes. Marine Ecology Progress S eries 207:141–154.
Sullivan, M.C., R.K. Cowen, K.W. Able, and M.P. Fahay. 2003. Effects of anthropogenic
and natural disturbance on a recently settled continental-shelf flatfish. Marine Ecology
Progress Series 260:237–253.
ter Braak, C.J.F., and P. Smilauer. 2002. CANOCO Reference manual and CanoDraw for
Windows user guide: Software for Canocoal Community Ordination (version 4.5). Microcomputer
power, Ithaca, NY.
Tiffan, K.F., D.W. Rondorf, and J.L. Skalicky. 2004. Imaging fall Chinook Salmon redds in
the Columbia River with a dual-frequency identification sonar. North American Journal
of Fisheries Management 24:1421–1426.
Turhan-Sayan, G. 2005. Real time electromagnetic-target classification using a novel
feature-extraction technique with PCA-based fusion. IEEE Transactions on Antennas
and Propagation 53(2):766–776.
Xie, Y., C.G.J. Michielsens, A.P. Gray, F.J. Martens, and J.L. Boffey. 2008. Observations of
avoidance reactions of migrating salmon to a mobile survey vessel in a riverine environment.
Canadian Journal of Fisheries and Aquatic Sciences 65:2178–2190.