Sciurus niger niger (Southern Fox Squirrel) Density and the
Diurnal Patterns, Occupancy, and Detection of Sympatric
Southern Fox Squirrels and S. carolinensis (Eastern Gray
Squirrel) on Spring Island, South Carolina
Katelyn Amspacher, Beau Bauer, Jayme Waldron, Ernie Wiggers, and Shane Welch
Southeastern Naturalist, Volume 18, Issue 2 (2019): 321–333
Full-text pdf (Accessible only to subscribers.To subscribe click here.)
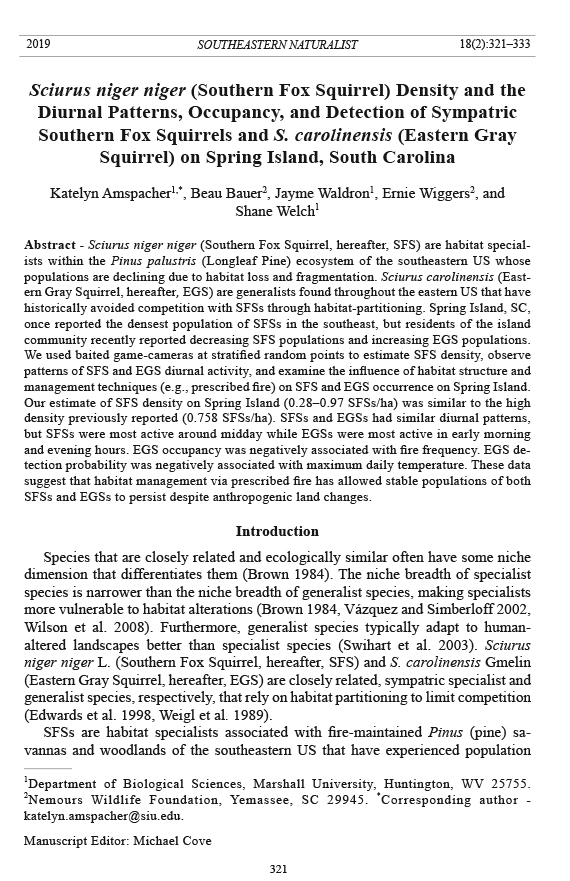
Southeastern Naturalist
321
K. Amspacher, B. Bauer, J. Waldron, E. Wiggers, and S. Welch
22001199 SOUTHEASTERN NATURALIST 1V8o(2l.) :1382,1 N–3o3. 32
Sciurus niger niger (Southern Fox Squirrel) Density and the
Diurnal Patterns, Occupancy, and Detection of Sympatric
Southern Fox Squirrels and S. carolinensis (Eastern Gray
Squirrel) on Spring Island, South Carolina
Katelyn Amspacher1,*, Beau Bauer2, Jayme Waldron1, Ernie Wiggers2, and
Shane Welch1
Abstract - Sciurus niger niger (Southern Fox Squirrel, hereafter, SFS) are habitat specialists
within the Pinus palustris (Longleaf Pine) ecosystem of the southeastern US whose
populations are declining due to habitat loss and fragmentation. Sciurus carolinensis (Eastern
Gray Squirrel, hereafter, EGS) are generalists found throughout the eastern US that have
historically avoided competition with SFSs through habitat-partitioning. Spring Island, SC,
once reported the densest population of SFSs in the southeast, but residents of the island
community recently reported decreasing SFS populations and increasing EGS populations.
We used baited game-cameras at stratified random points to estimate SFS density, observe
patterns of SFS and EGS diurnal activity, and examine the influence of habitat structure and
management techniques (e.g., prescribed fire) on SFS and EGS occurrence on Spring Island.
Our estimate of SFS density on Spring Island (0.28–0.97 SFSs/ha) was similar to the high
density previously reported (0.758 SFSs/ha). SFSs and EGSs had similar diurnal patterns,
but SFSs were most active around midday while EGSs were most active in early morning
and evening hours. EGS occupancy was negatively associated with fire frequency. EGS detection
probability was negatively associated with maximum daily temperature. These data
suggest that habitat management via prescribed fire has allowed stable populations of both
SFSs and EGSs to persist despite anthropogenic land changes.
Introduction
Species that are closely related and ecologically similar often have some niche
dimension that differentiates them (Brown 1984). The niche breadth of specialist
species is narrower than the niche breadth of generalist species, making specialists
more vulnerable to habitat alterations (Brown 1984, Vázquez and Simberloff 2002,
Wilson et al. 2008). Furthermore, generalist species typically adapt to humanaltered
landscapes better than specialist species (Swihart et al. 2003). Sciurus
niger niger L. (Southern Fox Squirrel, hereafter, SFS) and S. carolinensis Gmelin
(Eastern Gray Squirrel, hereafter, EGS) are closely related, sympatric specialist and
generalist species, respectively, that rely on habitat partitioning to limit competition
(Edwards et al. 1998, Weigl et al. 1989).
SFSs are habitat specialists associated with fire-maintained Pinus (pine) savannas
and woodlands of the southeastern US that have experienced population
1Department of Biological Sciences, Marshall University, Huntington, WV 25755.
2Nemours Wildlife Foundation, Yemassee, SC 29945. *Corresponding author -
katelyn.amspacher@siu.edu.
Manuscript Editor: Michael Cove
Southeastern Naturalist
K. Amspacher, B. Bauer, J. Waldron, E. Wiggers, and S. Welch
2019 Vol. 18, No. 2
322
declines throughout their range due to habitat loss and fragmentation (Perkins and
Conner 2004, Weigl et al. 1989). SFS life history might contribute to the species’
inability to rebound from population declines. SFSs are one of the largest and most
ground dwelling of all North American tree squirrels (Weigl et al. 1989). They are
relatively long-lived (7–10 y lifespan) and typically have just 1 litter per year with
an average 2.5 pups, which suggests they are a k-selected species (Tappe and Guynn
1998, Weigl et al. 1989). SFSs are normally found in low densities (less than 0.35
SFS/ha; Weigl et al. 1989).
Eastern Gray Squirrels are habitat generalists found in dense forests with woody
groundcover throughout the eastern US (Edwards et al. 1998). They typically live
8–9 y and frequently have 2 litters per year, averaging 2.6 pups per litter (Barkalow
et al. 1970). In addition, EGSs are often very abundant and reach high densities of
up to 15 squirrels/ha (Gurnell 1983).
Spring Island, SC, reported the densest SFS population in the southeast during the
1990s (Lee 1999), but residents reported decreased SFS populations and increased
EGS populations in the 2010s (C. Marsh and T. Mills, Spring Island Trust, pers.
comm.). In this study, we sought to evaluate whether changes in SFS and EGS population
changes occurred on Spring Island. We employed game cameras to estimate
SFS density, and occupancy models to examine the influence of habitat structure and
management technique (e.g., prescribed fire frequency) on SFS and EGS occurrence.
We hypothesized that decreased SFS density would be associated with the shift in
land use from open pine savanna—sustained by frequent prescribed burning—to
nature preserve/residential community in the early 1990s (Lee et al. 2008). We expected
that this shift in land use led to a subsequent shift in habitat management,
limiting prescribed fire prevalence, altering habitat structure (e.g., increased canopy
cover and denser forest), and allowing EGSs to encroach on SFS habitat. We further
hypothesized that habitat alterations limited the ability of SFSs and EGSs to partition
habitat, leading to temporal partitioning instead. We expected EGS site occupancy to
be greater than SFS site occupancy because EGSs are generalists, and SFS occupancy
to be positively associated with fire frequency and negatively associated with canopy
cover because of their association with the Pinus palustris Mill. (Longleaf Pine)
ecosystem. We predicted that the detection probability of SFSs and EGSs would be
similar because they are closely related species, and to be negatively associated with
maximum daily temperature and daily precipitation because of reduced squirrel activity
in heat and heavy rain (Weigl et al. 1989).
Field-Site Description
Spring Island is a 1200-ha sea island in South Carolina (Fig. 1). The island was
managed as a quail plantation from the mid-1900s until 1990 when it was sold for
residential development. Managers of quail plantations typically use frequent, lowintensity
prescribed fire to maintain large tracts of low basal-area pine/hardwoods
with an open, diverse understory (Moser et al. 2002, Stoddard 1931, Van Lear et al.
2005). Management with frequent, low-intensity fire also provided habitat for other
species of interest such as Picoides borealis Vieillot (Red-cockaded Woodpecker)
Southeastern Naturalist
323
K. Amspacher, B. Bauer, J. Waldron, E. Wiggers, and S. Welch
2019 Vol. 18, No. 2
and SFSs (Moser et al. 2002). Development on Spring Island led to increased human
activity and road construction, which fragmented the landscape and introduced a new
source of mortality for SFSs (Lee et al. 2008). Spring Island is now considered a lowdensity
housing development hidden behind dense vegetation along the perimeter of
the island to maintain privacy for 400 families. Land use includes a golf course, clay
range, sports complex (e.g., tennis courts and pool), and farm for residents’ use. The
Spring Island Trust, a nonprofit organization, was established to protect the environmental
and cultural history of the island and maintain 445 ha in nature preserves.
Methods
Data collection
We used historic landcover classifications (Lee 1999) and current satellite imagery
to establish 20 random points stratified by habitat class on Spring Island, SC
Figure 1. Twenty stratified random
points established on Spring
Island, SC, as sampling sites for
Southern Fox Squirrels and Eastern
Gray Squirrels.
Southeastern Naturalist
K. Amspacher, B. Bauer, J. Waldron, E. Wiggers, and S. Welch
2019 Vol. 18, No. 2
324
(Fig. 1). We set 250 m as the minimum distance between random points (Tye et al.
2015), but in practice, the minimum distance between sampled points was 285 m
and all other points were >300 m apart. We used a convex spherical crown densiometer
(Forestry Suppliers, Inc., Jackson, MS) to measure canopy cover at each point
in early June 2016, when sites were established. We digitized and georeferenced
25 y of historical prescribed burn maps from 1992 to 2017 using ArcGIS (Esri,
Redlands, CA) and calculated the average number of times each point was burned
during a 5-y period to reflect historical fire-return intervals (Stambaugh et al. 2011).
We placed a game camera (Bushnell 14 MP Trophy Cam HD Aggressor, Bushnell
Outdoor Products, Overland Park, KS) 70 cm above ground and 1.5 m from a pecan/
corn bait pile (~19 L) at each point and set motion-activated cameras to take
3 pictures every 10 sec on normal sensitivity settings (Tye et al. 2015). We visited
cameras every other day to change SD cards and replenish bait. We conducted camera
surveys from 24 to 30 June 2016 and from 20 to 26 April 2017.
Statistical analysis
Density and diurnal patterns. We calculated the area sampled using a 142.5-m
(half the minimum distance between points) radius around each point. A circle with
a diameter of 285 m around the random point covers 6.4 ha, which is similar to the
previously reported 6.5-ha average annual home range of SFSs on Spring Island
(Lee et al. 2008). We identified individual SFSs from photographs using unique
pelage characteristics (Tye et al. 2015). We divided the number of individuals
identified by the area sampled to estimate SFS density. We used package camtrapR
(Niedballa et al. 2017) in Program R (R Core Team 2018) to catalogue photographs
and create a record table with entries for both EGSs and SFSs. We formatted the
record table to only include records of the same species at the same station with
at least 30 min between photographs to maintain independence between records.
We used this record table and package camtrapR in Program R to calculate kernel
density diel activity overlap between EGSs and SFSs using the overlap coefficient
Dhat1 (Ridout and Linkie 2009). We assumed an overlap coefficient ≥0.5 indicated
similar species activity patterns and no avoidance behavior.
Occupancy. We used single-species multi-season occupancy models in program
PRESENCE (MacKenzie et al. 2002) to identify patterns of occupancy and
detection for both SFSs and EGSs. We assumed constant local extinction (ε) and colonization
(γ) for all models. We formatted encounter history with 2 seasons (2016
and 2017) and 7 replicates per season (14 total replicates) for each random point.
The SFS multi-season model had poor fit, so we adjusted SFS occupancy analysis to
2 separate single-species, single-season occupancy models. The 2017 single-season
SFS models were of poor fit and we excluded them from analysis. We retrieved
maximum daily temperature and daily precipitation data from the National Oceanic
and Atmospheric Administration (NOAA) station at the Marine Corps Air Station
Beaufort (38.6 km from Spring Island), z-standardized them, and incorporated the
data in models as detection covariates (Table 1). We used canopy cover and burn
frequency as occupancy covariates (Table 1). We used Spearman rho correlation to
Southeastern Naturalist
325
K. Amspacher, B. Bauer, J. Waldron, E. Wiggers, and S. Welch
2019 Vol. 18, No. 2
examine collinearity among covariates, retaining covariates with coefficients less
than r = 0.7. We constructed 1 constant model, 2 models examining single-detection
covariates, 2 models testing single-occupancy covariates, and 11 additive models
for each species (16 EGS models and 16 SFS models; Tables 2, 3). We ranked models
with Akaike’s information criterion (AIC) and adjusted SFS models to QAIC due
to overdispersion (ĉ2016 = 2.64; Akaike 1973, Burnham and Anderson 2002).
Results
We captured 12,584 photographs of SFSs on 9 cameras and 13,742 photographs
of EGSs on 9 cameras in 2016. We captured 37,645 photographs of
SFSs on 17 cameras and 25,895 photographs of EGSs on 11 cameras in 2017.
We identified 36 individual SFSs during the summer 2016 sampling season and
124 individual SFSs during the spring 2017 sampling season. We estimated 0.28
SFSs/ha in 2016, and 0.97 SFSs/ha in 2017. After adjusting the record table to
maintain independence between records, we incorporated 774 photographs of
SFSs and 563 photographs of EGSs in the diurnal pattern analysis. Activity overlap
indicated that SFSs and EGSs had similar diel activity patterns, but EGSs
were most active in early morning and late evening hours while SFSs were most
active around midday (Dhat1 = 0.62; Fig. 2).
Occupancy and detection covariates were not correlated (r = -0.47 and 0.19,
respectively). Only one EGS occupancy model, ψ(PF+CC), γ(.), ε(.), p(Temp),
Table 1. Explanation of occupancy and detection covariates.
Covariate Description
Occupancy
Burn frequency (PF) Average number of burns in a 5-y period from 1992 to 2017
Canopy cover (CC) Percent canopy cover
Detection
Temperature (Temp) Maximum daily temperature in °C, z-standardized
Precipitation (Precip) Daily precipitation in cm, z-standardized
Figure 2. Kernel
density estimate
of Eastern Gray
Squirrel (EGS)
and Southern
Fox Squirrel
(SFS) activity
throughout
the day (0:00–
24:00). Shaded
area indicates
activity overlap
between species
(Dhat1 = 0.62).
Southeastern Naturalist
K. Amspacher, B. Bauer, J. Waldron, E. Wiggers, and S. Welch
2019 Vol. 18, No. 2
326
Table 3. Southern Fox Squirrel occupancy models ranked according to QAIC model selection, 2016.
Supported models (ΔQAIC < 2.0) are indicated by an asterisk (*). PF = prescribed fire frequency, CC
= canopy cover, Temp = maximum daily temperature, and Precip = daily precipitation.
Model QAIC ΔQAIC ωi Likelihood k Deviance
ψ(.) , p(.)* 40.67 0.00 0.2523 1.0000 2 96.68
ψ(.), p(Temp)* 42.26 1.59 0.1139 0.4516 3 95.59
ψ(PF), p(.)* 42.48 1.81 0.1021 0.4045 3 96.18
ψ(.), p(Precip)* 42.55 1.88 0.0985 0.3906 3 96.34
ψ(CC), p(.)* 42.58 1.91 0.0971 0.3848 3 96.43
ψ(PF), p(Temp) 44.07 3.40 0.0461 0.1827 4 95.09
ψ(.), p(Temp+Precip) 44.12 3.45 0.0449 0.1782 4 95.23
ψ(CC), p(Temp) 44.17 3.50 0.0438 0.1738 4 95.34
ψ(PF+CC), p(.) 44.19 3.52 0.0434 0.1720 4 95.41
ψ(PF), p(Precip) 44.36 3.69 0.0399 0.1580 4 95.84
ψ(CC), p(Precip) 44.45 3.78 0.0381 0.1511 4 96.09
ψ(PF+CC), p(Temp) 45.78 5.11 0.0196 0.0777 5 94.32
ψ(PF), p(Temp+Precip) 45.93 5.26 0.0182 0.0721 5 94.72
ψ(CC), p(Temp+Precip) 46.03 5.36 0.0173 0.0686 5 94.98
ψ(PF+CC), p(Precip) 46.06 5.39 0.0170 0.0675 5 95.06
ψ(PF+CC), p(Temp+Precip) 47.64 6.97 0.0077 0.0307 6 93.95
Table 2. Eastern Gray Squirrel occupancy models ranked according to AIC model selection. Supported
models (ΔAIC < 2.0) are indicated by and asterisk (*). PF = prescribed fire frequency, CC =
canopy cover, Temp = maximum daily temperature, and Precip = daily precipitation.
Model QAIC ΔQAIC ωi Likelihood k Deviance
ψ(PF+CC), γ(.), ε(.), p(Temp)* 203.34 0.00 0.9594 1.0000 7 189.34
ψ(PF), γ(.), ε(.), p(Temp) 210.92 7.58 0.0217 0.0226 6 198.92
ψ(PF), γ(.), ε(.), p(Temp+Precip) 212.85 9.51 0.0083 0.0086 7 198.85
ψ(PF+CC), γ(.), ε(.), p(Temp+Precip) 213.98 10.64 0.0047 0.0049 8 197.98
ψ(CC), γ(.), ε(.), p(Temp) 216.42 13.08 0.0014 0.0014 6 204.42
ψ(PF), γ(.), ε(.), p(.) 216.64 13.30 0.0012 0.0013 5 206.64
ψ(.), γ(.), ε(.), p(Temp) 217.44 14.10 0.0008 0.0009 5 207.44
ψ(PF+CC), γ(.), ε(.), p(.) 217.77 14.43 0.0007 0.0007 6 205.77
ψ(CC), γ(.), ε(.), p(Temp+Precip) 218.36 15.02 0.0005 0.0005 7 204.36
ψ(PF), γ(.), ε(.), p(Precip) 218.41 15.07 0.0005 0.0005 6 206.41
ψ(.), γ(.), ε(.), p(Temp+Precip) 219.38 16.04 0.0003 0.0003 6 207.38
ψ(PF+CC), γ(.), ε(.), p(Precip) 219.54 16.20 0.0003 0.0003 7 205.54
ψ(CC), γ(.), ε(.), p(.) 222.14 18.80 0.0001 0.0001 5 212.14
ψ(.), γ(.), ε(.), p(.) 223.19 19.85 0.0000 0.0000 4 215.19
ψ(CC), γ(.), ε(.), p(Precip) 223.92 20.58 0.0000 0.0000 6 211.92
ψ(.), γ(.), ε(.), p(Precip) 224.96 21.62 0.0000 0.0000 5 214.96
Southeastern Naturalist
327
K. Amspacher, B. Bauer, J. Waldron, E. Wiggers, and S. Welch
2019 Vol. 18, No. 2
was supported (ΔAIC < 2.0; Table 2). According to the supported model, average
EGS occupancy was 0.45 ± 0.14 (95% CI = 0.21–0.69) and average daily detection
probability was 0.72 ± 0.04 (95% CI = 0.64–0.80). The model failed to detect
a significant association between canopy cover and occupancy (β = 2.98, SE =
3.29; Table 4), but occupancy was negatively associated with burn frequency (β =
-1.751, SE = 0.864; Fig. 3). Maximum daily temperature had a negative effect on
Figure 3. Relationship between burn frequency and Eastern Gray Squirrel occupancy (ψ),
based on output from ψ(PF), γ(.), ε(.), p(Temp). Line = estimate, gray area = 95% CL.
Table 4. Beta output from supported Eastern Gray Squirrel occupancy model, ψ(PF+CC), γ(.), ε(.),
p(Temp). SE = standard error, LCL = lower 95% confidence limit, and UCL = upper 95% confidence
limit. Significance is denoted by an asterisk (*). PF = prescribed fire frequency, CC = canopy cover,
Temp = maximum daily temperature, and Precip = daily precipitation.
Parameter β SE LCL UCL
ψ -3.02 2.93 -8.76 2.72
ψ.PF* -1.75 0.86 -3.45 -0.06
ψ.CC 2.98 3.29 -3.48 9.43
γ -0.56 0.63 -1.80 0.67
ε -1.26 0.80 -2.83 0.32
p* 9.72 1.24 7.29 12.15
p.Temp* -0.28 0.04 -0.35 -0.20
Southeastern Naturalist
K. Amspacher, B. Bauer, J. Waldron, E. Wiggers, and S. Welch
2019 Vol. 18, No. 2
328
detection probability (β = -0.278, SE = 0.039; Fig. 4). Burn frequency and canopy
cover were important EGS occupancy covariates, accounting for 99.7% and 96.7%
of cumulative model weights, respectively. Maximum daily temperature was the
most important detection covariate, accounting for 99.7% of model weight. Daily
precipitation was a poor predictor of detection probability and only accounted for
1.5% of cumulative model weight.
Five SFS occupancy models were supported (ΔAIC < 2.0; Table 3), but the
constant model was most supported. Model-averaged SFS occupancy from the 5
supported models was 0.30 ± 0.08 (95% CI = 0.16–0.45) in 2016. However, naïve
SFS occupancy in 2017 was 85%. Model-averaged detection probability was 0.51
± 0.04 (95% CI = 0.42 – 0.57) in 2016. Three of the 5 supported models included
constant occupancy (Table 3). Burn frequency was included in 1 supported model,
but we failed to detect a significant association between burn frequency and SFS occupancy
(β2016 = -0.31, SE2016 = 0.72; Table 5). Likewise, canopy cover was included
as an occupancy covariate in 1 supported model, but we failed to detect a significant
association between canopy cover and SFS occupancy (β2016 = -0.95, SE2016 = 3.11;
Table 5). Three of the 5 supported models included constant detection probability
(Table 3). Maximum daily temperature was included as a detection covariate in
one supported model, but we failed to detect a significant association between
Figure 4. Relationship between Eastern Gray Squirrel detection probability (P) and maximum
daily temperature, based on output from ψ(PF), γ(.), ε(.), p(Temp). Line = estimate,
gray area = 95% CL.
Southeastern Naturalist
329
K. Amspacher, B. Bauer, J. Waldron, E. Wiggers, and S. Welch
2019 Vol. 18, No. 2
maximum daily temperature and SFS detection probability (β2016 = -0.15, SE2016 =
0.13; Table 5). Daily precipitation was included in one supported model, but we
failed to detect a significant association between precipitation and SFS detection
probability (β2016 = 0.49, SE2016 = 1.37; Table 5). Burn frequency and canopy cover
were moderate predictors of SFS occupancy based on parameter weights (29.4%
and 28.4%, respectively, in 2016). Maximum daily temperature and daily precipitation
were moderate predictors of SFS detection probability based on parameter
weights (31.2% and 28.2%, respectively, in 2016).
Discussion
Lee et al. (2008) reported 0.758 SFSs/ha on Spring Island in 1999. At that time,
Spring Island SFS density was much higher than density estimates of other SFS
populations, which varied from 0.084 to 0.38 SFSs/ha (Conner et al. 1999, Edwards
et al. 1989, Hilliard 1979, Kantola and Humphrey 1990, Weigl et al. 1989). We
expected that Spring Island residents accurately perceived a decline in SFS populations
due to anthropogenic development and the general trend of declining SFS
populations (Wood and Davis 1981). We recorded 0.28 SFSs/ha and 0.97 SFSs/ha
in June 2016 and April 2017, respectively. Lee (1999) estimated density in May,
and his estimate fell about halfway between our density estimates from April and
June. The difference in our density estimates is likely associated with decreased
Table 5. Beta output from supported SFS occupancy models, 2016. SE = standard error, LCL = lower
95% confidence limit, UCL = upper 95% confidence limit, significance is denoted by an asterisk (*).
PF = prescribed fire frequency, CC = canopy cover, Temp = maximum daily temperature, and Precip
= daily precipitation.
Model/Parameter β SE LCL UCL
ψ(.), p(.)
ψ -0.20 0.73 -1.63 1.23
p* 1.16 0.48 0.22 2.11
ψ(.), p(Temp)
ψ -0.20 0.73 -1.63 1.23
p 6.34 4.57 -2.60 15.29
p.Temp -0.15 0.13 -0.42 0.11
ψ(PF), p(.)
ψ 0.12 1.03 -1.91 2.14
ψ.PF -0.31 0.72 -1.71 1.10
p* 1.16 0.48 0.22 2.11
ψ(.), p(Precip)
ψ -0.20 0.73 -1.63 1.23
p 1.01 0.64 -0.24 2.25
p.Precip 0.49 1.67 -2.19 3.16
ψ(CC), p(.)
ψ 0.55 2.58 -4.51 5.62
ψ.CC -0.95 3.11 -7.03 5.14
p * 1.16 0.48 0.22 2.11
Southeastern Naturalist
K. Amspacher, B. Bauer, J. Waldron, E. Wiggers, and S. Welch
2019 Vol. 18, No. 2
330
activity of SFSs during summer months due to increased temperature and decreased
food availability (Weigl et al. 1989), consequently leading to decreased ability to
detect SFSs during summer months. Our SFS occupancy models did not indicate a
significant effect of temperature on detection probability, likely because the variation
in temperatures within sampling seasons was far less than variation between
sampling seasons. Thus, the spring 2017 density estimate (0.97 SFSs/ha) is likely
representative of the current Spring Island SFS population. Our hypothesis that
Spring Island SFS density had declined since the 1990s was not supported, as SFS
density appears to be similar to what Lee reported in 1999. Though our estimate of
SFS density (0.97 SFSs/ha) is high for the species, it is still much lower than densities
achieved by many EGS populations (up to 15 EGSs/ha; Gurnell 1983).
Habitat cues such as canopy cover and illumination influence prey species’ behavior
due to perceived predation risk (Thorson et al. 1992). Anthropogenic activity
altered the habitat structure on Spring Island and may have affected the behavior
of both SFSs and EGSs and the population metrics perceived by residents. For instance,
as habitat changed from open pine savannas and woodlands managed with
fire to denser mixed pine–hardwood forests, EGSs likely began foraging in these
newly suitable areas, giving the perception of many EGSs where there had once
been SFSs. In addition, citizen scientists and opportunistic data are often biased
towards detections in frequently travelled areas, especially when searching for
low-density populations such as SFSs (Crall et al. 2010, Fitzpatrick et al. 2009).
Observations near homes with dense vegetation likely affected EGS and SFS population
perceptions on Spring Island.
We expected that the shift in land use from managed quail plantation to nature
preserve/residential community in the early 1990s altered the degree to which
prescribed fire was used to manage wildlife habitat. We predicted this change allowed
EGSs to disperse as habitat grew increasingly denser, increasing interactions
between SFSs and EGSs. We observed both SFSs and EGSs at the same camera at
half the sites sampled. Spring Island SFSs and EGSs had similar activity patterns,
indicating that they were not avoiding each other temporally (Fig. 2), but EGSs did
appear to be most active in early morning and late evening hours; SFSs were most
active around midday. Lashley et al. (2018) observed that SFSs in North Carolina
were also most active in the middle of the day.
Occupancy analysis indicated that EGSs were negatively associated with prescribed
fire (Fig. 3). Fire maintains an open understory and prevents woody stem
encroachment within the Longleaf Pine ecosystem of the Southeast (Frost 1993),
but EGSs prefer dense forests with woody undergrowth (Edwards et al. 1998). SFSs
prefer open, grassy understories (Edwards et al. 1998), but our SFS occupancy
analysis for this high-density population failed to detect significant effects of burn
frequency or canopy cover on occupancy. Previous SFS analyses similar to ours
have varied results. Prince et al. (2016) found no direct effect of fire on SFSs and
indicated the importance of hardwoods as a source of food and refugia within pine
savannas and woodlands. However, Boone et al. (2017) indicated a negative association
between occupancy by Fox Squirrels and fire interval. Greene and McCleery
Southeastern Naturalist
331
K. Amspacher, B. Bauer, J. Waldron, E. Wiggers, and S. Welch
2019 Vol. 18, No. 2
(2017) observed that open habitat structure was important for S. n. shermani Moore
(Sherman’s Fox Squirrel) even when they occurred in altered/human-impacted
landscapes, linking the subspecies to the fire-maintained Longleaf Pine ecosystem
and indicating the importance of management to maintain open canopies and
understories. Occupancy analysis indicated that EGS detection probability was
negatively associated with maximum daily temperature (Fig. 4). SFS density analysis
suggested a negative relationship between maximum daily temperature and
SFS detection, reflecting reduced activity during hot summer months when food is
generally scarce (Weigl et al. 1989).
Spring Island, SC, still supports a high-density population of SFSs. Exurbanization
influences habitat structure, particularly near heavily trafficked areas. The
perceived decline in SFS population on Spring Island is likely due to the dense
vegetation surrounding and hiding houses and observations biased towards areas
with high human activity. SFSs are typically found in comparatively low densities
in more open areas, often managed with fire. In contrast, EGSs are found in higher
densities in thicker vegetation that is not managed with fire. Residential communities
within the Longleaf Pine ecosystem that strive to maintain natural diversity
should preserve and manage habitat for specialists with management tools such as
prescribed fire or mechanical thinning.
Acknowledgments
We thank Chris Marsh, Tony Mills, and Tom Murphy for their assistance in establishing
this project and David Barron for his assistance in the field. Funding for this project was
provided by the Spring Island Trust, Nemours Wildlife Foundation, and Marshall University.
This material is based upon work supported by the National Science Foundation under Cooperative
Agreement No. OIA-1458952.
Literature Cited
Akaike, H. 1973. Information theory and an extension of the maximum likelihood principle.
Pp. 267–281, In B.N. Petrov and F. Cazakil (Eds.). Proceedings of the 2nd International
Symposium Information Theory. Akademiai Kidao, Budapest, Hungary.
Barkalow, F.S., Jr., R.B. Hamilton, and R.F. Soots Jr. 1970. The vital statistics of an unexploited
gray squirrel population. Journal of Wildlife Management 34(3):489–500.
Boone, W.W., R.A. McCleery, and B.E. Reichert. 2017. Fox Squirrel response to forest
restoration treatments in Longleaf Pine. Journal of Mammalogy 98(6):1594–1603.
Brown, J.H. 1984. On the relationship between abundance and distribution of species. The
American Naturalist 124(2):255–279.
Burnham, K.P., and D.R. Anderson. 2002. Model Selection and Multimodel Inference, A
Practical Information–Theoretic Approach. Springer-Verlag New York, NY. 487 pp.
Conner, L.M., J.L. Landers, and W.K. Michener. 1999. Fox Squirrel and Gray Squirrel associations
within minimally disturbed Longleaf Pine forests. Proceedings of the Annual
Conference of the Southeastern Association of Fish and Wildlife Agencies 53:364–374.
Crall, A.W., G.J. Newman, C.S. Jarnevich, T.J. Stohlgren, D.M. Waller, and J. Graham.
2010. Improving and integrating data on invasive species collected by citizen scientists.
Biological Invasions 12:3419–3428.
Southeastern Naturalist
K. Amspacher, B. Bauer, J. Waldron, E. Wiggers, and S. Welch
2019 Vol. 18, No. 2
332
Edwards, J.W., D.C. Guynn, and M.R. Lennartz. 1989. Habitat use by Southern Fox Squirrel
in coastal South Carolina. Proceedings of the Annual Conference of the Southeastern
Association of Fish and Wildlife Agencies 43:337–345.
Edwards, J.W., D.G. Heckel, and D.C. Guynn. 1998. Niche overlap in sympatric populations
of Fox and Gray Squirrels. Journal of Wildlife Management 62(1):354–363.
Fitzpatrick, M.C., E.L. Preisser, A.M. Ellison, and J.S. Elkinton. 2009. Observer bias and
the detection of low-density populations. Ecological Applications 19(7):1673–1679.
Frost, C.C. 1993. Four centuries of changing landscape patterns in the Longleaf Pine ecosystem.
Proceedings of the Tall Timbers Fire Ecology Conference 18:17-43.
Gurnell, J. 1983. Squirrel numbers and the abundance. Mammal Review 13(2/3/4):133–148.
Greene, D.U., and R.A. McCleery. 2017. Multi-scale responses of Fox Squirrels to landuse
changes in Florida: Utilization mimics historic pine savannas. Forest Ecology and
Management 391:42–51.
Hilliard, T.H. 1979. Radio-telemetry of Fox Squirrels in the Georgia coastal plain. M.Sc.
Thesis. University of Georgia, Athens, GA. 113 pp.
Kantola, A.T., and S.R. Humphrey. 1990. Habitat use by Sherman’s Fox Squirrel (Sciurus
niger shermani) in Florida. Journal of Mammalogy 71:411–419.
Lashley, M.A., M.V. Cove, M.C. Chitwood, G. Penido, B. Gardner, C.S. DePerno, and
C.E. Moorman. 2018. Estimating wildlife activity curves: Comparison of methods and
sample size. Scientific Reports 8:4173.
Lee, J.C. 1999. Ecology of the Southern Fox Squirrel on Spring Island, South Carolina.
M.Sc. Thesis. University of Georgia, Athens, GA. 64 pp.
Lee, J.C., D.A. Osborn, and K.V. Miller. 2008. Characteristics of a high density population
of Southern Fox Squirrels. American Midland Naturalist 159(2):385–393.
MacKenzie, D.I., J.D. Nichols, G.B. Lachman, S. Droege, J.A. Royle, and C.A. Langtimm.
2002. Estimating site-occupancy rates when detection probabilities are less than one.
Ecology 83(8):2248–2255.
Moser, W.K., S.M. Jackson, V. Podrazsky, and D.R. Larsen. 2002. Examination of stand
structure on quail plantations in the Red Hills region of Georgia and Florida managed
by the Stoddard–Neel system: An example for forest managers. Forestry 75(4):443–449.
Niedballa, J., A. Courtiol, R. Sollmann, J. Mathai, S.T. Wong, A.T.T. Nguyen, A.B. Mohamed,
A. Tilker, and A. Wilting. 2017. camtrapR: Camera-trap data management and
preparation of occupancy and spatial capture–recapture analyses. R package version
0.99.9. Pp. 1–62. Available online at https://CRAN.R-project.org/package=camtrapR.
Accessed 1 August 2017.
Perkins, M.W., and L.M. Conner. 2004. Habitat use of Fox Squirrels in southwestern Georgia.
Journal of Wildlife Management 68(3):509–513.
Prince, A., M.C. Chitwood, M.A. Lashley, C.S. DePerno, and C.E. Moorman. 2016. Resource
selection by Southeastern Fox Squirrels in a fire-maintained forest system. Journal
of Mammalogy 97(2):631-638.
R Core Team. 2018. R: A language and environment for statistical computing. R Foundation
for Statistical Computing, Vienna, Austria. Available online at http://www.R-project.
org/. Accessed 1 January 2018.
Ridout, M.S., and M. Linkie. 2009. Estimating overlap of daily activity patterns from
camera-trap data. Journal of Agricultural, Biological, and Environmental Statistics
14:322–337.
Stambaugh, M.C., R.P. Guyette, and J.M. Marschall. 2011. Longleaf Pine (Pinus palustris
Mill.) fire scars reveal new details of a frequent fire regime. Journal of Vegetation Science
22:1094–1104.
Southeastern Naturalist
333
K. Amspacher, B. Bauer, J. Waldron, E. Wiggers, and S. Welch
2019 Vol. 18, No. 2
Stoddard, H.L., Sr. 1931. The Bobwhite Quail: Its Habits, Preservation, and Increase.
Charles Scribner’s Sons, New York, NY. 559 pp.
Swihart, R.K., T.M. Gehring, M.B. Kolozsvary, and T.E. Nupp. 2003. Responses of “resistant”
vertebrates to habitat loss and fragmentation: The importance of niche breadth and
range boundaries. Diversity and Distributions 9:1–18.
Tappe, P.A., and D.C. Guynn Jr. 1998. Southeastern Fox Squirrels: r- or K-selected? Implications
for management. Pp. 239–249, In M.A. Steele, J.F. Merritt, and D.A. Zegers
(Eds.). Ecology and Evolutionary Biology of Tree Squirrels. Virginia Museum of Natural
History Special Publication 6. Martinsville, VA. 84 pp.
Thorson, J.M., R.A. Morgan, J.S. Brown, and J.E. Norman. 1992. Direct and indirect cues
of predatory risk and patch use by Fox Squirrels and Thirteen-lined Ground Squirrels.
Behavioral Ecology 9(2):151–157.
Tye, C.A., D.U. Greene, W.M. Giuliano, and R.A. McCleery. 2015. Using camera-trap photographs
to identify individual Fox Squirrels (Sciurus niger) in the Southeastern United
States. The Wildlife Society Bulletin 39(3):645–650.
Van Lear, D.H., W.D. Carroll, P.R. Kapeluck, and R. Johnson. 2005. History and restoration
of the Longleaf Pine–grassland ecosystem: Implications for species at risk. Forest
Ecology and Management 211:150–165.
Vázquez, D.P., and D. Simberloff. 2002. Ecological specialization and susceptibility to
disturbance: Conjectures and refutations. The American Naturalist 159(6):606–623.
Weigl, P.D., M.A. Steele, L.J. Sherman, J.C. Ha, and T.L. Sharpe. 1989. The Ecology of the
Fox Squirrel (Sciurus niger) in North Carolina: Implications for Survival in the Southeast.
Bulletin of Tall Timbers Research Station, Number 24. 93 pp.
Wilson, S.K., S.C. Burgess, A.J. Cheal, M. Emslie, R. Fisher, I. Miller, N.V.V. Polunin, and
H.P.A. Sweatman. 2008. Habitat utilization by coral reef fish: Implications for specialists
vs. generalists in a changing environment. Journal of Animal Ecology 77:220–228.
Wood, G.W., and J.R. Davis. 1981. A survey of perceptions of Fox Squirrels in South Carolina.
Clemson University Forestry Bulletin, 29:1–6.